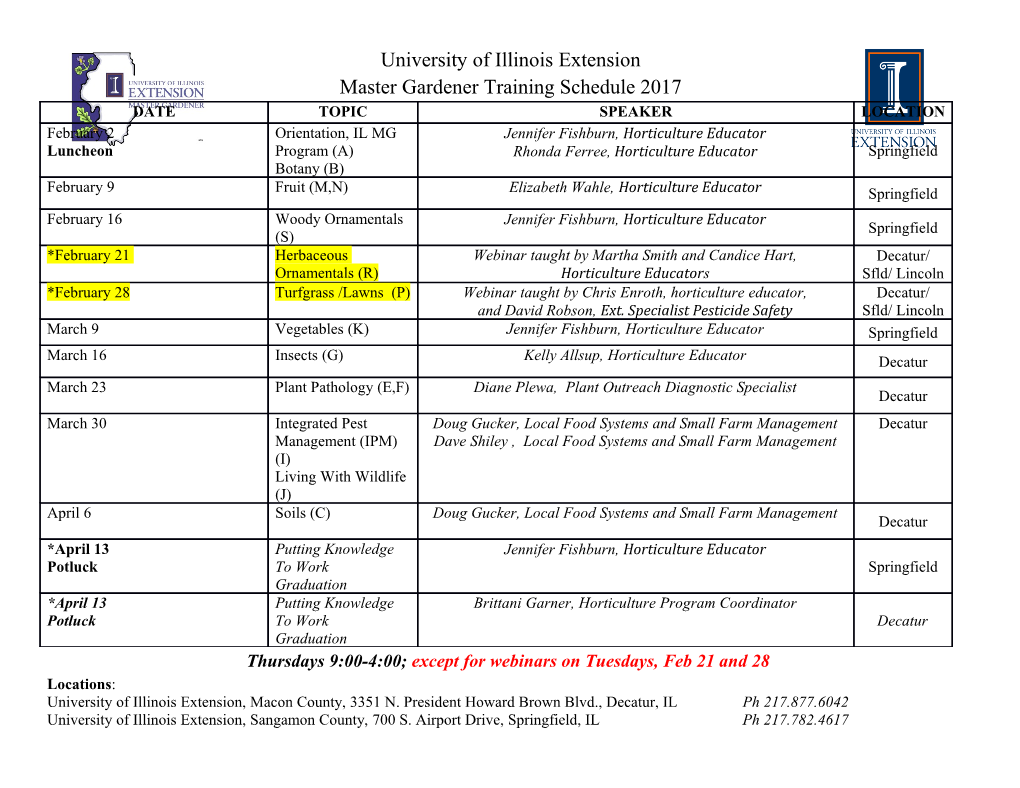
Bioprospecting the flora of southern Africa: optimising plant selections Dissertation for Master of Science Errol Douwes 2005 Submitted in fulfilment of the requirements for the degree of Master of Science in the School of Biological and Conservation Sciences at the University of KwaZulu-Natal Pietermaritzburg, South Africa ii Preface The work described in this dissertation was carried out at the Ethnobotany Unit, South African National Biodiversity Institute, Durban and at the School of Biological and Conservation Sciences, University of KwaZulu-Natal, Pietermaritzburg from January 2004 to November 2005 under the supervision of Professor TJ. Edwards (School of Biological and Conservation Sciences, University of KwaZulu-Natal, Pietermaritzburg) and Dr N. R. Crouch (Ethnobotany Unit, South African National Biodiversity Institute, Durban). These studies, submitted for the degree of Master of Science in the School of Biological and Conservation Sciences, University of KwaZulu-Natal, Pietermaritzburg, represent the original work of the author and have not been submitted in any form to another university. Use of the work of others has been duly acknowledged in the text. We certify that the above statement is correct Novem:RE. Douwes Professor T.J. Edwards /JIo-~rA ..............................~ ...~ Dr N.R. Crouch iii Acknowledgements Sincere thanks are due to my supervisors Prof. Trevor Edwards and Dr Neil Crouch for their guidance and enthusiasm in helping me undertake this project. Dr Neil Crouch is thanked for financial support provided by way of SANSI (South African National Siodiversity Institute) and the NDDP (Novel Drug Development Platform). Prof. Trevor Edwards and Prof. Dulcie Mulholland are thanked for financial support provided by way of NRF (National Research Foundation) grant-holder bursaries. The Ajax Foundation, WESSA (Wildlife and Environmental Society of South Africa), as well as Chris Davidson and Sharon Christoph are thanked for financial support. Thanks to Prof. Dulcie Mulholland for her gracious manner in dealing with the many natural product chemistry queries, and Dr Robin Mackey for her assistance with some of the statistical obstacles. The staff at SANSI are thanked for their assistance and support. These include: Hannelie Snyman for provision of maps and databases; Olwen Grace for her insights and for the provision of a list of keywords used in plant selection; Mathabo Qoko for her help with datacapture; and Yolande Steenkamp for provision of a list of endemic plants. Dr Maureen Wolfson, Yashica Singh, Helen Noble and Dr Hugh Glen are thanked for their insights and assistance. Thanks also to the many others who helped in different ways. These include: Chris Dalzell, Prof. Daniel E. Moerman, Prof. Joe Felsenstein, Prof. Alan Amory, Ian Galvin, and Anneita Ramkisson. Finally, thanks to my family for their unending support, encouragement, inspiration and love. iv Abstract Focused procedures which streamline and optimise plant prioritisation and selection in bioprospecting have the potential to save both time and resources. A variety of semi­ quantitative techniques were assessed for their ability to prioritise ethnomedicinal taxa in the Flora ofSouthern Africa (FSA) region. These techniques were subsequently expanded upon for application in plant selection for the Novel Drug Development Platform bioprospecting programme. Least squares regression analyses were used to test the hypothesis that ethnomedicinal plant use in southern Africa is strictly random, i.e. no order or family contains significantly more medicinal plants, than any other order or family. This hypothesis was falsified revealing several 'hot' plant orders. The distribution of southern African ethnomedicinal taxa was investigated, and revealed low ethnomedicinal plant usage in the Western Cape and Northern Cape. The historical settlement of Bantu tribes in the eastern regions of southern Africa was one explanation for this discrepancy. Growth forms of ethnomedicinal taxa in 'hot' orders (identified in the regression analysis) were analysed. The results indicated no clear preferences across orders, but rather a preference for particular growth forms in certain orders. With respect to distribution, endemism and Red Data List status of ethnomedicinal taxa, the Western Cape had the greatest proportion of endemics and Namibia had the highest proportion of Red Data Listed ethnomedicinal taxa. With respect to chemotaxonomy, the Asteraceae contained the highest proportion of terpenoids, the Rubiaceae the highest proportion of alkaloids and the Fabaceae the highest proportion of f1avonoids. v The predictive value of regression analyses was tested against an existing analysis of anti-malarials and the subsequent in vitro bioassays on Plasmodium fa/ciparum. In particular, the ability of these analyses to identify plants with antiplasmodial IC50 values of ~ 10 Ilgjml was assessed. Most species in 'hot' genera showed comparatively good antiplasmodial activities (IC50 ~ 10 Ilgjml). Plant candidates were prioritised for screening anti-tuberculosis, anti-diabetes and immune-modulatory compounds, using a weighting system based on; their ethnomedicinal application, chemotaxonomic potential, frequency in ethnomedicinal trade, association with the relative disease, toxicity, Red Data status, indigenous or endemic status, and family selection in ethnomedicine (identified through regression analyses). Other taxa were short-listed due to their presence in biodiversity hotspots where few ethnomedicinal plant use records are documented, and still others were incorporated due to their taxonomic association with efficacious exotic allies. Statistical analyses of the weighting processes employed were not possible in the absence of screening results which are due only in December 2006. The legislation governing bioprospecting in South Africa is discussed and several recommendations are presented to minimise negative impacts on the industry. vi Table of contents Preface ii Acknowledgements iii Abstract iv Table of contents vi List of figures x List of tables ·.. ·· .. ·· ··· xii List of abbreviations xvi Chapter 1 Introduction 1 1.1 Bioprospecting - history and current global overview 2 1.2 Bioprospecting in the flora of southern Africa 5 1.3 The Antimalarials Consortium (Figure 1.3) 9 1.3.1 Plant selection for antimalarial drug development 10 1.4 The Novel Drug Development Platform (Figure 1.3) 10 1.4.1 Plant selection by the NDDP 11 1.5 Approaches to selecting plants for bioprospecting 11 1.5.1 Focused plant selection methods 13 1.5.2 Chemotaxonomy, phylogeny and the search for novel drugs 15 1.5.3 Plant selection in current bioprospecting programmes 17 1.6 Bioprospecting legislation in South Africa 18 Chapter 2 Prioritisation of ethnomedicinal plants for bioprospecting: a multi- disciplinary approach 20 Abstract 20 2.1 Introduction 21 2.2 Methods ~ 26 2.2.1 Data source and organisation 26 2.2.2 Primary regression analyses 26 2.2.2.1 Residual values 27 2.2.2.2 Plotting regression data 27 2.2.2.3 Analysis of families within selected orders 27 2.2.2.4 Secondary regression analyses 28 2.2.3 Analysis of plant growth forms 28 2.2.4 Regional distribution, endemicity and Red Data List status 28 vii 2.2.5 Evaluation of phytochemical trends in 'hot' families 29 2.3 Results ".29 2.3.1 Primary regression analyses 29 2.3.1.1 Residual values 30 2.3.1.2 Plotting regression data 31 2.3.1.3 Analysis of families within selected orders .32 2.3.1.4 Secondary regression analyses 35 2.3.2 Analysis of plant growth forms 36 2.3.3 Regional distribution, endemicity and Red Data List status 37 2.3.4 Evaluation of phytochemical trends in 'hot' families .42 2.4 Discussion 45 2.4.1 Statistical evaluation 47 2.4.2 Growth form 48 2.4.3 Regional distribution, endemicity and Red Data List status .49 2.4.4 Historical use of ethnomedicinal plants in the FSA 52 2.4.5 Phytochemical evaluation 55 2.5 Conclusion 56 Chapter 3 Bioprospecting antimalarials in southern Africa: retrospective analyses of plant selections 58 Abstract 58 3.1 Introduction 59 3.2. Methods 61 3.2.1 Selection of plant candidates 62 3.2.2. Primary regression analyses 67 3.2.2.1 Residual values 67 3.2.2.2 Plotting regression data 68 3.2.2.3 Analysis of families within selected orders 68 3.2.2.4 Assessing bioassay results from taxa in 'hot' families 68 3.2.3 Secondary regression analyses 68 3.3. Results 69 3.3.1 Selection of plant candidates 69 3.3.2. Primary regression analyses 70 3.3.2.1 Residual values 70 3.3.2.2 Plotting regression data 71 3.3.2.3 Analysis of families within selected orders 72 3.3.2.4 Assessing bioassay results from taxa in 'hot' families 74 3.3.3 Secondary regression analyses 76 viii 3.4. Discussion 77 3.4.1 Keyword associations 79 3.4.2 Primary regression analyses 80 3.4.3 Secondary regression analyses 82 3.5 Conclusion 82 Chapter 4 Bioprospecting for anti-tuberculosis, anti-diabetes and immune- modulatory plants in the FSA 84 Abstract 84 4.1 Introduction 85 4.2 Methods 89 4.2.1 Generation of an ethnodirected plant candidate list (Set 1) 89 4.2.1.1 Keywords (Column N) 91 4.2.1.2 Taxon names (Column B) 98 4.2.1.3 Family (Column C) 99 4.2.1.4 Indigenous status of taxa (Column D) 99 4.2.1.5 Endemic status of taxa (Column E) 100 4.2.1.6 Ethnomedicinal status of taxa in the FSA region (Column F) 100 4.2.1.7 Explicit use of taxa for disease treatment (Column G) 100 4.2.1.8 Documented positive or negative associations (Column H) 100 4.2.1.9 Toxicity of recorded taxa (Column I) 101 4.2.1.10 Red Data Listed taxa in the
Details
-
File Typepdf
-
Upload Time-
-
Content LanguagesEnglish
-
Upload UserAnonymous/Not logged-in
-
File Pages274 Page
-
File Size-