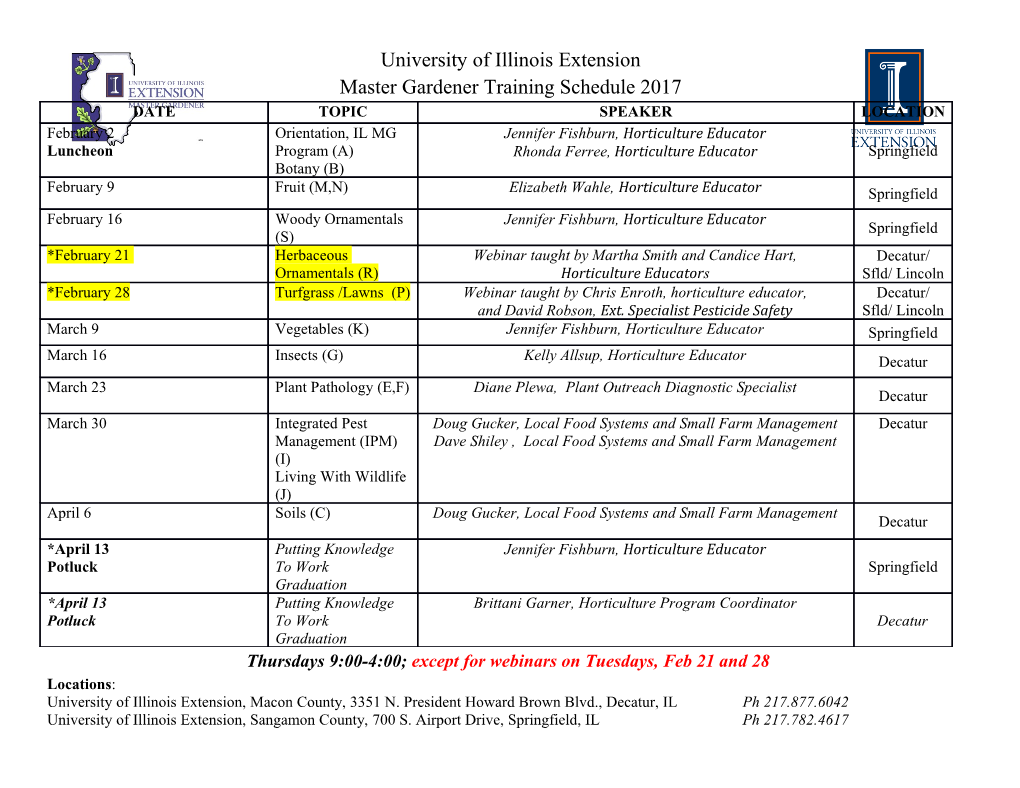
C HA P TER 1 1 MULTILEVEL MOdeLING FOR PSYCHOLOGISTS John B. Nezlek Multilevel analyses have become increasingly com- including different aspects of analyses such as cen- mon in psychological research, although unfortu- tering, modeling error, weighted analyses, and cate- nately, many researchers’ understanding of gorical independent and dependent measures. I also multilevel analysis has lagged behind this increased offer suggestions about how to interpret the results interest and use. Many researchers have heard of of analyses and how to report results in papers. and are curious about multilevel modeling (MLM), Finally, although they are in flux, I discuss software but they are unfamiliar with it, perhaps so unfamil- options. iar that they do not know where to start. This unfa- This chapter is intended as an introduction for miliarity is probably due in part to the fact that those who are not familiar with MLM. When writing many graduate programs in psychology do not offer this chapter, the only statistical training I assumed (or have not offered) courses in multilevel analysis. readers would have was an understanding of basic This chapter is an attempt to meet this need by ordinary least squares (OLS) regression. Analysts familiarizing readers with MLM as it pertains to psy- who are familiar with the basics of MLM may find chological research broadly defined. some value in my treatment, but advanced topics are In writing this chapter, I had two goals in mind. not covered. Other chapters in this handbook cover First, I wanted readers to learn the basics of multi- some of these topics, such as Chapters 18, 19, and level analysis. Second, I wanted to increase readers’ 20 of this volume. awareness of the multilevel perspective so that they might recognize the multilevel features of the data DefininG Multilevel Analysis they have collected and would be able to be formu- late more clearly research questions that might What Is Meant by Multilevel? involve multilevel data. As Kreft and de Leeuw A multilevel data structure is one in which observa- (1998) noted, “Once you know that hierarchies tions at one level of analysis are nested (or clustered exist you see them everywhere” (p. 1). Conversely, or grouped) within observations at another level of if you do not know how to conceptualize a multi- analysis. Sometimes, multilevel data structures are level data structure and the accompanying analyses, described simply as nested or as hierarchically nested. you may not see or recognize hierarchies anywhere. The critical, defining feature of such multilevel data In this chapter, I provide a rationale for MLM: is that observations at one level of analysis are not why it is necessary, its advantages over other tech- independent of each other—there is an interdepen- niques, and so forth. I describe the basic structure of dence among the data that needs to be taken into univariate multilevel analyses: the nature of the account. In this chapter, I focus on two-level data models and the types of parameters they can esti- structures, but the framework and logic I use to mate and how to conduct multilevel analyses, describe two-level structures are readily applicable DOI: 10.1037/13621-011 APA Handbook of Research Methods in Psychology: Vol. 3. Data Analysis and Research Publication, H. Cooper (Editor-in-Chief) 219 Copyright © 2012 by the American Psychological Association. All rights reserved. John B. Nezlek to data structures with more than two levels. I dis- Table 11.1 cuss how to conceptualize the levels of a model in a separate section later in this chapter. Relationships: Positive at Within-Group Level and The lack of independence among observations is Negative at Between-Group Level exemplified in studies of groups (e.g., students in classes or workers in work groups). Individuals in the Group 1 Group 2 Group 3 same group all share the characteristics associated X Y X Y X Y with their group, whereas they differ from each other 26 31 29 29 31 26 in terms of individual-level characteristics. In addi- 27 32 30 30 32 27 tion, group-level characteristics such as teacher expe- 28 33 31 31 33 28 rience or the style of group leaders are likely to vary 29 34 32 32 34 29 30 35 33 33 35 30 across groups. Therefore, individual differences such as performance can be examined in terms of explana- Mean 28 33 31 31 33 28 tory variables at two levels of analysis—the individual (skill, motivation, etc.) and the group (e.g., teacher characteristics)—and members of different groups may Table 11.2 vary in terms of measures at both levels of analysis. Relationships: Negative at Within-Group Level and The question is how to disentangle relationships Positive at Between-Group Level between an outcome of interest and measures at multiple levels of analysis. For example, why are Group 1 Group 2 Group 3 Mary’s test scores higher than Jane’s? Is this because Mary is smarter or works harder than Jane (an indi- X Y X Y X Y vidual-level relationship), or is it because Mary’s 11 18 19 23 19 28 teacher is better than Jane’s and so the students in 12 17 20 22 20 27 13 16 21 21 21 26 Mary’s class tend to have higher grades on average 14 15 22 20 22 25 (a group-level relationship), or is it both? Moreover, 15 14 23 19 23 24 it is possible that the individual-level relationship between how intelligent a student is and her grades Mean 13 16 21 21 21 26 varies across classes. The relationship may be stron- ger in some classes than in others, and it may be of positive and some could be negative. Seeing data such interest to understand (model) the differences as these, one might ask, “Which one is correct?” The between classes in such relationships. answer is that neither is correct. If an analyst is inter- When addressing such questions it is critical to ested in constructs defined at the between-group level, recognize that relationships at different levels of anal- then the between-group relationships are correct. Cor- ysis are mathematically independent. In a study in respondingly, if the interest is in constructs defined at which persons are nested within groups, relationships the within-group level, then the within-group relation- at the between-group level tell us nothing about rela- ships are correct, with the caveat that these relation- tionships at the within-group level, and vice versa. ships may not be consistent across groups. This independence is illustrated by the data in Tables In MLM the term group refers to an organizing 11.1 and 11.2. In Table 11.1, the within-group rela- unit or cluster. For studies of actual groups (e.g., tionships are positive and the between-group rela- work groups) this creates no confusion; however, tionships are negative, and in Table 11.2, the for other types of nesting (e.g., observations within within-group relationships are negative and the person, such as in a diary study), the term group can between-group relationships are positive. be confusing. So when observations are nested Moreover, these two examples do not exhaust the within people, a person constitutes a group. In a possible combinations. Relationships at the within- cross-cultural study, cultures might be the groups; group level might vary across groups; some could be in a community psychology study, communities 220 Multilevel Modeling for Psychologists might be the groups, and so forth. Using the term they assume that relationships between the outcome group in this way is a tradition in MLM, and as and the predictors are identical in all groups, an confusing as it may be for those unfamiliar with untenable assumption, and second, they do not MLM, I follow this tradition. model error properly. Even if interaction terms between the dummy variables and the predictors are Analytic Strategies for Analyzing included (which can be unwieldy with many groups Multilevel Data and multiple predictors), the two sources of error Before describing multilevel random coefficient mod- are not modeled properly. eling, which is the currently accepted “gold stan- In a two-level multilevel data structure, there are dard” for analyzing multilevel data sets, I briefly two sources of error: one associated with sampling review other methods, in part to highlight some of Level-1 observations, and the other associated with the strengths of the MLM techniques I consider in sampling Level-2 observations. For example, in a detail. One way to distinguish such methods is to diary study in which observations are nested within distinguish aggregation versus disaggregation meth- persons, there is error associated with sampling per- ods. In aggregation methods, within-group summary sons and with sampling days. The error associated statistics are calculated (e.g., means) and then ana- with sampling persons is well understood, but a lyzed. For example, a researcher might calculate for coefficient representing the within-person relation- each U.S. state means for two variables (literacy and ship between daily stress and daily anxiety for an percentage of immigrants) and then calculate a corre- individual also has a sampling error. A coefficient lation at the state level. Such relationships are per- that is based on the 2 weeks a study was conducted fectly acceptable providing one does not commit will probably be similar to, but not the same as, a what is called the ecological fallacy (Robinson, 1950), coefficient based on another 2-week period. More- which occurs when it is assumed that the between- over, the unreliability of such a coefficient (how group relationships exist at the within-group level.
Details
-
File Typepdf
-
Upload Time-
-
Content LanguagesEnglish
-
Upload UserAnonymous/Not logged-in
-
File Pages23 Page
-
File Size-