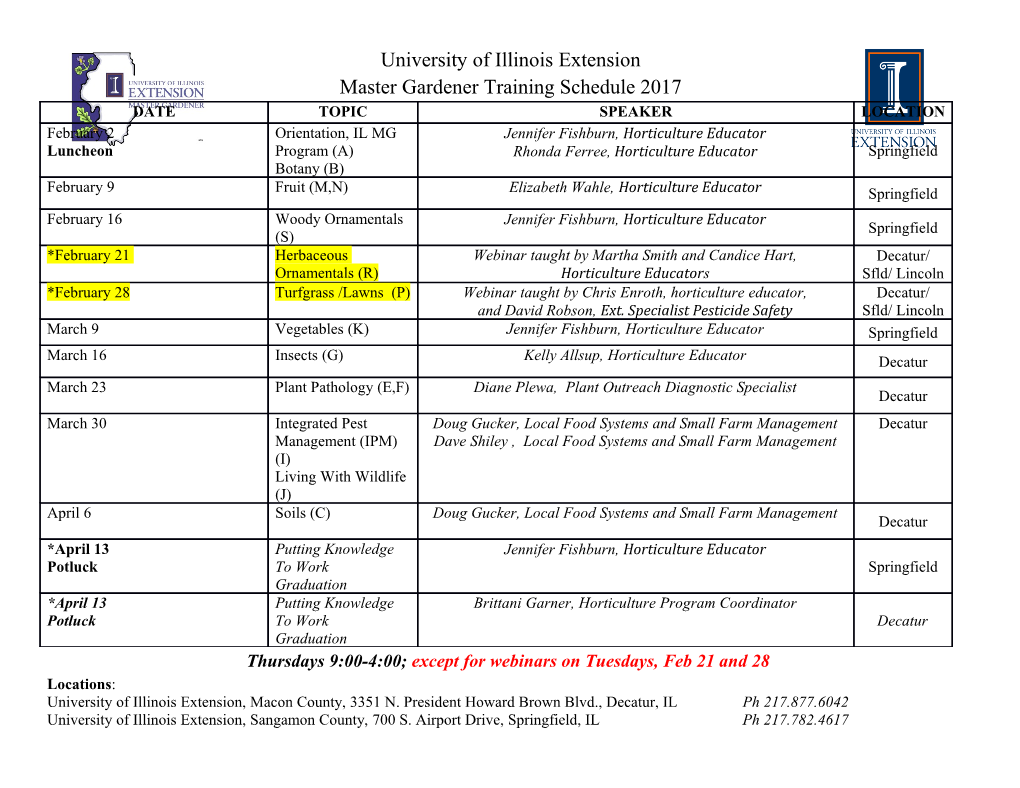
ELEG-636: Statistical Signal Processing Gonzalo R. Arce Department of Electrical and Computer Engineering University of Delaware Spring 2010 Gonzalo R. Arce (ECE, Univ. of Delaware) ELEG-636: Statistical Signal Processing Spring 2010 1 / 34 Course Objectives & Structure Course Objectives & Structure Objective: Given a discrete time sequence {x(n)}, develop Statistical and spectral signal representation Filtering, prediction, and system identification algorithms Optimization methods that are Statistical Adaptive Course Structure: Weekly lectures [notes: www.ece.udel.edu/∼arce] Periodic homework (theory & Matlab implementations) [15%] Midterm & Final examinations [85%] Textbook: Haykin, Adaptive Filter Theory. Gonzalo R. Arce (ECE, Univ. of Delaware) ELEG-636: Statistical Signal Processing Spring 2010 2 / 34 Course Objectives & Structure Course Objectives & Structure Broad Applications in Communications, Imaging, Sensors. Emerging application in Brain-imaging techniques Brain-machine interfaces, Implantable devices. Neurofeedback presents real-time physiological signals from MRIs in a visual or auditory form to provide information about brain activity. These signals are used to train the patient to alter neural activity in a desired direction. Traditionally, feedback using EEGs or other mechanisms has not focused on the brain because the resolution is not good enough. Gonzalo R. Arce (ECE, Univ. of Delaware) ELEG-636: Statistical Signal Processing Spring 2010 3 / 34 Wiener (MSE) Filtering Theory Wiener Filtering Problem Statement Produce an estimate of a desired process statistically related to a set of observations £ ¤ ¢ £ ¤ ¦ § ¦ ¥ ¢ ¡ ¡ ¢ £ ¤ ¦ § ¥ § ¦ ¤ ¨ © ¤ ¦ § ¦ ¦ ¥ Historical Notes: The linear filtering problem was solved by Andrey Kolmogorov for discrete time – his 1938 paper “established the basic theorems for smoothing and predicting stationary stochastic processes” Norbert Wiener in 1941 for continuous time – not published until the 1949 paper Extrapolation, Interpolation, and Smoothing of Stationary Time Series Gonzalo R. Arce (ECE, Univ. of Delaware) ELEG-636: Statistical Signal Processing Spring 2010 5 / 34 Wiener (MSE) Filtering Theory Wiener Filtering System restrictions and considerations: Filter is linear Filter is discrete time Filter is finite impulse response (FIR) The process is WSS Statistical optimization is employed Gonzalo R. Arce (ECE, Univ. of Delaware) ELEG-636: Statistical Signal Processing Spring 2010 6 / 34 Wiener (MSE) Filtering Theory Wiener Filtering For the discrete time case ∗ ∗ ∗ " " ! ! # − % $ The filter impulse response is finite and given by w ∗ for k = 0, 1, ··· , M − 1 h = k k 0 otherwise The output dˆ(n) is an estimate of the desired signal d(n) x(n) and d(n) are statistically related ⇒ dˆ(n) and d(n) are statistically related Gonzalo R. Arce (ECE, Univ. of Delaware) ELEG-636: Statistical Signal Processing Spring 2010 7 / 34 Wiener (MSE) Filtering Theory Wiener Filtering In convolution and vector form M−1 ˆ ∗ H d(n)= wk x(n − k)= w x(n) = kX0 where T w = [w0, w1, ··· , wM−1] [filter coefficient vector] x = [x(n), x(n − 1), ··· , x(n − M + 1)]T [observation vector] The error can now be written as e(n)= d(n) − dˆ(n)= d(n) − wH x(n) Question: Under what criteria should the error be minimized? Selected Criteria: Mean squared-error (MSE) ∗ J(w)= E{e(n)e (n)} (∗) Result: The w that minimizes J(w) is the optimal (Wiener) filter Gonzalo R. Arce (ECE, Univ. of Delaware) ELEG-636: Statistical Signal Processing Spring 2010 8 / 34 Wiener (MSE) Filtering Theory Wiener Filtering Utilizing e(n)= d(n) − wH x(n) in (∗) and expanding, ∗ J(w) = E{e(n)e (n)} ∗ = E{(d(n) − wH x(n))(d (n) − xH (n)w)} ∗ = E{|d(n)|2 − d(n)xH (n)w − wH x(n)d (n) +wH x(n)xH (n)w} ∗ = E{|d(n)|2}− E{d(n)xH (n)}w − wH E{x(n)d (n)} +wH E{x(n)xH (n)}w (∗∗) Let R = E{x(n)xH (n)} [autocorrelation of x(n)] ∗ p = E{x(n)d (n)} [cross correlation between x(n) and d(n)] Then (∗∗) can be compactly expressed as 2 H H H J(w)= σd − p w − w p + w Rw where we have assumed x(n) & d(n) are zero mean, WSS Gonzalo R. Arce (ECE, Univ. of Delaware) ELEG-636: Statistical Signal Processing Spring 2010 9 / 34 Wiener (MSE) Filtering Theory Wiener Filtering The MSE criteria as a function of the filter weight vector w 2 H H H J(w)= σd − p w − w p + w Rw Observation: The error is a quadratic function of w Consequences: The error is an M–dimensional bowl–shaped function of w with a unique minimum Result: The optimal weight vector, w0, is determined by differentiating J(w) and setting the result to zero ∇wJ(w)|w=w0 = 0 A closed form solution exists Gonzalo R. Arce (ECE, Univ. of Delaware) ELEG-636: Statistical Signal Processing Spring 2010 10 / 34 Wiener (MSE) Filtering Theory Wiener Filtering Example Consider a two dimensional case, i.e., a M = 2 tap filter. Plot the error surface and error contours. Error Surface Error Contours Gonzalo R. Arce (ECE, Univ. of Delaware) ELEG-636: Statistical Signal Processing Spring 2010 11 / 34 Wiener (MSE) Filtering Theory Wiener Filtering Aside (Matrix Differentiation): For complex data, wk = ak + jbk , k = 0, 1, ··· , M − 1 the gradient, with respect to wk , is ∂J ∂J ∇k (J)= + j , k = 0, 1, ··· , M − 1 ∂ak ∂bk The complete gradient is thus given by ∂J + j ∂J ∇0(J) ∂a0 ∂b0 ∇ (J) ∂J + j ∂J 1 ∂a1 ∂b1 ∇w(J)= . = . ∇ − (J) ∂J j ∂J M 1 ∂a − + ∂b − M 1 M 1 Gonzalo R. Arce (ECE, Univ. of Delaware) ELEG-636: Statistical Signal Processing Spring 2010 12 / 34 Wiener (MSE) Filtering Theory Wiener Filtering Example H Let c and w be M × 1 complex vectors. For g = c w, find ∇w(g) Note M−1 M−1 H ∗ ∗ g = c w = ck wk = ck (ak + jbk ) = = kX0 kX0 Thus ∂g ∂g ∇k (g) = + j ∂ak ∂bk ∗ ∗ = ck + j(jck )= 0, k = 0, 1, ··· , M − 1 Result: For g = cH w ∇0(g) 0 ∇1(g) 0 ∇w(g)= . = . = 0 . ∇M-1(g) 0 Gonzalo R. Arce (ECE, Univ. of Delaware) ELEG-636: Statistical Signal Processing Spring 2010 13 / 34 Wiener (MSE) Filtering Theory Wiener Filtering Example H Now suppose g = w c. Find ∇w(g) In this case, M−1 M−1 H ∗ g = w c = wk ck = ck (ak − jbk ) = = kX0 kX0 and ∂g ∂g ∇k (g) = + j ∂ak ∂bk = ck + j(−jck )= 2ck , k = 0, 1, ··· , M − 1 Result: For g = wH c ∇0(g) 2c0 ∇1(g) 2c1 ∇w(g)= . = . = 2c . ∇M-1(g) 2c − M 1 Gonzalo R. Arce (ECE, Univ. of Delaware) ELEG-636: Statistical Signal Processing Spring 2010 14 / 34 Wiener (MSE) Filtering Theory Wiener Filtering Example H Lastly, suppose g = w Qw. Find ∇w(g) In this case, M−1 M−1 ∗ g = wi wj qi,j = = Xi 0 Xj 0 M−1 M−1 = (ai − jbi )(aj + jbj )qi,j = = Xi 0 Xj 0 ∂g ∂g ⇒∇k (g) = + j ∂ak ∂bk M−1 = 2 (aj + jbj )qk,j + 0 = Xj 0 M−1 = 2 wj qk,j j=0 Gonzalo R. Arce (ECE, Univ. of Delaware) ELEG-636:X Statistical Signal Processing Spring 2010 15 / 34 Wiener (MSE) Filtering Theory Wiener Filtering Result: For g = wH Qw M−1 q0,i wi ∇ (g) i=0 0 MP−1 ∇1(g) q1,i wi ∇w(g)= . = 2 i=0 = 2Qw . . P . ∇ (g) M-1 M-1 qM−1,i wi = i 0 P Observation: Differentiation result depends on matrix ordering Gonzalo R. Arce (ECE, Univ. of Delaware) ELEG-636: Statistical Signal Processing Spring 2010 16 / 34 Wiener (MSE) Filtering Theory Wiener Filtering Returning to the MSE performance criteria 2 H H H J(w)= σd − p w − w p + w Rw Approach: Minimize error by differentiating with respect to w and set result to 0 ∇w(J) = 0 − 0 − 2p + 2Rw = 0 ⇒ Rw0 = p [normal equation] Result: The Wiener filter coefficients are defined by −1 w0 = R p Question: Does R−1 always exist? Recall R is positive semi-definite, and usually positive definite Gonzalo R. Arce (ECE, Univ. of Delaware) ELEG-636: Statistical Signal Processing Spring 2010 17 / 34 Wiener (MSE) Filtering Theory Orthogonality Principle Orthogonality Principle Consider again the normal equation that defines the optimal solution Rw0 = p H ∗ ⇒ E{x(n)x (n)}w0 = E{x(n)d (n)} Rearranging ∗ H E{x(n)d (n)}− E{x(n)x (n)}w0 = 0 ∗ H E{x(n)[d (n) − x (n)w0]} = 0 ∗ E{x(n)e0(n)} = 0 ∗ Note: e0(n) is the error when the optimal weights are used, i.e., ∗ ∗ H e0(n)= d (n) − x (n)w0 Gonzalo R. Arce (ECE, Univ. of Delaware) ELEG-636: Statistical Signal Processing Spring 2010 18 / 34 Wiener (MSE) Filtering Theory Orthogonality Principle Thus ∗ x(n)e0(n) 0 x(n − 1)e∗(n) 0 ∗ 0 E{x(n)e0(n)} = E . = . ∗ x(n − M + 1)e (n) 0 0 Orthogonality Principle A necessary and sufficient condition for a filter to be optimal is that the estimate error, e∗(n), be orthogonal to each input sample in x(n) Interpretation: The observations samples and error are orthogonal and contain no mutual “information” Gonzalo R. Arce (ECE, Univ. of Delaware) ELEG-636: Statistical Signal Processing Spring 2010 19 / 34 Wiener (MSE) Filtering Theory Minimum MSE Objective: Determine the minimum MSE −1 Approach: Use the optimal weights w0 = R p in the MSE expression 2 H H H J(w) = σd − p w − w p + w Rw 2 H H H −1 ⇒ Jmin = σd − p w0 − w0 p + w0 R(R p) 2 H H H = σd − p w0 − w0 p + w0 p 2 H = σd − p w0 Result: 2 H −1 Jmin = σd − p R p −1 where the substitution w0 = R p has been employed Gonzalo R.
Details
-
File Typepdf
-
Upload Time-
-
Content LanguagesEnglish
-
Upload UserAnonymous/Not logged-in
-
File Pages33 Page
-
File Size-