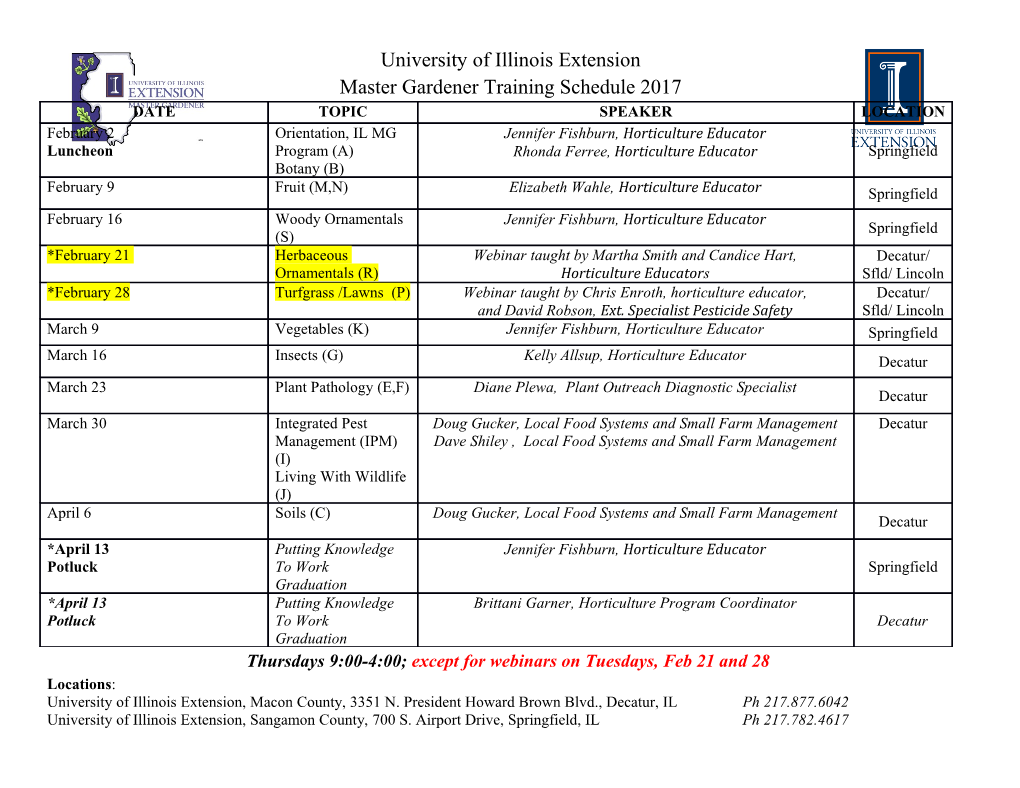
bioRxiv preprint doi: https://doi.org/10.1101/2020.10.04.325175; this version posted October 5, 2020. The copyright holder for this preprint (which was not certified by peer review) is the author/funder. All rights reserved. No reuse allowed without permission. Rational Inattention and Tonic Dopamine John G. Mikhael1;2∗, Lucy Lai1, Samuel J. Gershman3 1Program in Neuroscience, Harvard Medical School, Boston, MA 02115 2MD-PhD Program, Harvard Medical School, Boston, MA 02115 3Department of Psychology and Center for Brain Science, Harvard University, Cambridge, MA 02138 ∗Corresponding Author Correspondence: john [email protected] 1 bioRxiv preprint doi: https://doi.org/10.1101/2020.10.04.325175; this version posted October 5, 2020. The copyright holder for this preprint (which was not certified by peer review) is the author/funder. All rights reserved. No reuse allowed without permission. 1 Abstract 2 Slow-timescale (tonic) changes in dopamine (DA) contribute to a wide variety of processes in reinforce- 3 ment learning, interval timing, and other domains. Furthermore, changes in tonic DA exert distinct 4 effects depending on when they occur (e.g., during learning vs. performance) and what task the subject 5 is performing (e.g., operant vs. classical conditioning). Two influential theories of tonic DA|the average 6 reward theory and the Bayesian theory in which DA controls precision|have each been successful at 7 explaining a subset of empirical findings. But how the same DA signal performs two seemingly distinct 8 functions without creating crosstalk is not well understood. Here we reconcile the two theories under the 9 unifying framework of `rational inattention,' which (1) conceptually links average reward and precision, 10 (2) outlines how DA manipulations affect this relationship, and in so doing, (3) captures new empirical 11 phenomena. In brief, rational inattention asserts that agents can increase their precision in a task (and 12 thus improve their performance) by paying a cognitive cost. Crucially, whether this cost is worth paying 13 depends on average reward availability, reported by DA. The monotonic relationship between average re- 14 ward and precision means that the DA signal contains the information necessary to retrieve the precision. 15 When this information is needed after the task is performed, as presumed by Bayesian inference, acute 16 manipulations of DA will bias behavior in predictable ways. We show how this framework reconciles a 17 remarkably large collection of experimental findings. In reinforcement learning, the rational inattention 18 framework predicts that learning from positive and negative feedback should be enhanced in high and low 19 DA states, respectively, and that DA should tip the exploration-exploitation balance toward exploitation. 20 In interval timing, this framework predicts that DA should increase the speed of the internal clock and 21 decrease the extent of interference by other temporal stimuli during temporal reproduction (the central 22 tendency effect). Finally, rational inattention makes the new predictions that these effects should be 23 critically dependent on the controllability of rewards, that post-reward delays in intertemporal choice 24 tasks should be underestimated, and that average reward manipulations should affect the speed of the 25 clock|thus capturing empirical findings that are unexplained by either theory alone. Our results suggest 26 that a common computational repertoire may underlie the seemingly heterogeneous roles of DA. 27 Keywords: tonic dopamine, rational inattention, average reward, Bayesian inference, precision, reinforce- 28 ment learning, interval timing, controllability, post-reward delay 29 Author Summary 30 The roles of tonic dopamine (DA) have been the subject of much speculation, partly due to the variety of 31 processes it has been implicated in. For instance, tonic DA modulates how we learn new information, but also 2 bioRxiv preprint doi: https://doi.org/10.1101/2020.10.04.325175; this version posted October 5, 2020. The copyright holder for this preprint (which was not certified by peer review) is the author/funder. All rights reserved. No reuse allowed without permission. 32 affects how previously learned information is used. DA affects the speed of our internal timing mechanism, 33 but also modulates the degree to which our temporal estimates are influenced by context. DA improves 34 performance in some tasks, but seems only to affect confidence in others. Are there common principles that 35 govern the role of DA across these domains? In this work, we introduce the concept of `rational inattention,' 36 originally borrowed from economics, to the DA literature. We show how the rational inattention account of 37 DA unites two influential theories that are seemingly at odds: the average reward theory and the Bayesian 38 theory of tonic DA. We then show how this framework reconciles the diverse roles of DA, which cannot be 39 addressed by either theory alone. 40 Introduction 41 The functions of dopamine (DA) have been a subject of debate for several decades, due in part to the 42 bewildering variety of processes in which it participates. DA plays diverse roles in reinforcement learning 43 and action selection [1{9], motor control [10{12], vision [13, 14], interval timing [15{18], and attention [19]. 44 Furthermore, DA functions through at least two channels: fast-timescale (phasic) signals and slow-timescale 45 (tonic) signals [20]. While a large body of evidence has shown that phasic DA corresponds remarkably 46 well with a `reward prediction error' in reinforcement learning models [1{3, 21, 22], the functions of tonic 47 DA, which span the domains above, remain unclear. Does this diversity of function reflect a heterogeneous 48 computational repertoire? Or are there common principles that govern the role of tonic DA across these 49 domains? 50 Across both experiments and theory, tonic DA is most widely studied in the domains of reinforcement learning 51 and decision making. Experiments with Parkinson's patients and healthy subjects have suggested that, when 52 learning the associations between actions (or stimuli) and rewards, high DA facilitates learning from positive 53 feedback, and low DA facilitates learning from negative feedback across a variety of tasks [4, 5, 23{27]. On 54 the other hand, when this learned information must subsequently be used to select actions, DA seems to 55 control the exploration-exploitation trade-off: Here, high DA promotes exploitation of actions with higher 56 learned value and increases motivation. Low DA, on the other hand, promotes exploration [28{30] (but see 57 [31, 32] and Discussion) and decreases motivation [33{35]. Recently developed computational models allow 58 DA to achieve both learning and performance roles by endowing it with separate computational machinery 59 for each [27, 36]. However, whether and how the effects of DA during learning and performance may be 60 related at an algorithmic level remain open questions. 3 bioRxiv preprint doi: https://doi.org/10.1101/2020.10.04.325175; this version posted October 5, 2020. The copyright holder for this preprint (which was not certified by peer review) is the author/funder. All rights reserved. No reuse allowed without permission. 61 Another well-studied role of DA relates to its influence on interval timing. Much like in reinforcement 62 learning, DA also seems to have two broad effects here. First, DA seems to modulate the speed of the internal 63 timing mechanism: Acutely increasing DA levels results in behaviors consistent with a faster `internal clock,' 64 and acutely decreasing DA levels results in a slower internal clock [15{17, 37, 38]. In addition, unmedicated 65 Parkinson's patients, who are chronically DA-depleted, exhibit a distinct timing phenomenon known as the 66 `central tendency': When these patients learn intervals of different durations, they tend to overproduce 67 the shorter intervals and underproduce the longer intervals [39{41]. While the central tendency has been 68 observed in healthy subjects [42{46] and animals [47], it is most pronounced in unmedicated Parkinson's 69 patients, and DA repletion in these patients rescues veridical timing [39]. How the effects of DA on the speed 70 of the internal clock and the central tendency are related, if at all, remains unclear. 71 Reinforcement learning and interval timing, though related at a theoretical and neural level [22, 48], have, 72 until recently, largely been treated separately [49{51]. This has led to separate theories of DA developing for 73 each: In reinforcement learning, an influential hypothesis has posited that tonic DA reflects average reward 74 availability in a given context [34]. In other domains, however, tonic DA's role has been best explained as 75 reflecting precision within a Bayesian framework [52], which we discuss below. The view that DA modulates 76 precision (operationalized as the signal-to-noise ratio) has empirical grounding in interval timing [53{57], 77 motor control [58], vision [13, 14], and audition [59]. Interestingly, beyond true precision, DA has also 78 been associated with estimated precision, or the agent's estimate of its actual precision (or its confidence), 79 independently of any changes in true precision [13, 60{62]. This is an important distinction, because a 80 mismatch between true and estimated precision results in an underconfident or overconfident agent, which 81 may affect behavior. In sum, the dual role of DA in true and estimated precision|and under what conditions 82 this role holds|remains elusive. Once more, the duality of DA is not well understood. 83 Inspired by recent attempts to integrate the domains of reinforcement learning and interval timing [49{51], 84 we will begin by introducing the concept of `rational inattention,' borrowed from behavioral economics [63{ 85 65]. We will show that this framework unifies the two influential, yet seemingly distinct, algorithmic theories 86 of tonic DA. We will then show that this framework predicts various empirical phenomena of reinforcement 87 learning and interval timing, which cannot be explained by either theory alone.
Details
-
File Typepdf
-
Upload Time-
-
Content LanguagesEnglish
-
Upload UserAnonymous/Not logged-in
-
File Pages66 Page
-
File Size-