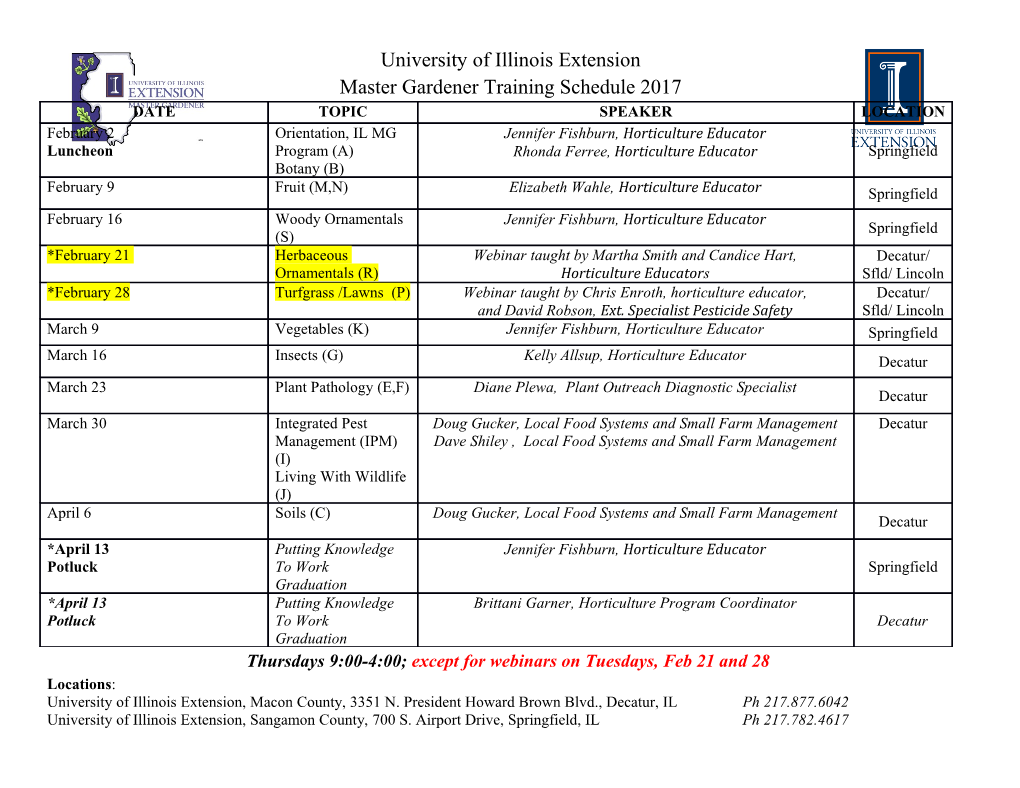
Answers The mean daily SP return is Descriptive Statistics Variable N N Mean Median Tr Mean StDev SE Mean S P re Variable Min Max Q Q S P re With roughly trading days p er year this works out to an annualized return of or an annualized return Use of the arithmetic mean requires that the data b e a random sample from a p opulation that is at least reasonably Gaussian Here is a time series plot of the values There do esnt app ear to b e auto correlation but there are unusual values in the p erio d around the sto ckmarket crash of 0.1 0.0 -0.1 S & P return -0.2 0 1000 2000 3000 4000 5000 6000 7000 8000 9000 Day Even more imp ortantly averaging returns this way do esnt make much sense since a increase followed by a decrease do es not result in zero change in principal that is To get the geometric mean of the returns we calculate the change in the logged SP index values determine the mean of these values and then antilog Here is the required information c Jerey S Simono Descriptive Statistics Variable N N Mean Median Tr Mean StDev SE Mean Change i Variable Min Max Q Q Change i The geometric mean of the returns is or smaller than what would b e predicted by the arithmetic mean It results in an annualized return of or a annualized return The geometric mean requires that the changes in logged index be a random sample from a p opulation that is at least reasonably Gaussian Here is a time series plot of the values which is similar to that of the returns 0.00 -0.05 Change in logged index -0.10 0 1000 2000 3000 4000 5000 6000 7000 8000 9000 Day The semilog estimate of the return is obtained by regressing the log of the SP index on time c Jerey S Simono Regression Analysis The regression equation is Logged S P index Day Predictor Coef StDev T P Constant Day S RSq RSqadj Analysis of Variance Source DF SS MS F P Regression Error Total The semilog estimate is or smaller than what would b e predicted by the geometric mean It results in an annualized return of or a annualized return The semilog estimate requires that the errors from the regression b e a random sample from a p opulation that is at least reasonably Gaussian Here is a time series plot of the standardized residuals which clearly not appropriate here and we prefer the geometric mean shows auto correlation The semilog estimate is of the returns as the b est estimate c Jerey S Simono 2.0 1.5 1.0 0.5 0.0 -0.5 -1.0 -1.5 Standardized residuals -2.0 -2.5 0 1000 2000 3000 4000 5000 6000 7000 8000 9000 Day It is very apparent that the p erio d around the sto ckmarket crash of Octob er is unusually volatile This can b e quantied by lo oking at the changes in logged index for all of the days except Octob er February and the values for those days Descriptive Statistics Variable N N Mean Median Tr Mean StDev SE Mean Change i Variable Min Max Q Q Change i Variable N Mean Median Tr Mean StDev SE Mean Change i Variable Min Max Q Q Change i c Jerey S Simono The estimated return without those days was for an annualized return of More imp ortantly the standard deviation of the change in logged index has dropp ed from to In contrast the days around the crash were money losing geometric mean or an annualized return of and tremendously volatile standard deviation of change in logged index of or more than times larger than the standard deviation of the surrounding days First lets lo ok at a few scatter plots Rememberthatalower value for academic reputation is a go o d thing 200 100 Academic reputation 0 0 102030405060708090100 Top 10% freshman c Jerey S Simono 200 100 Academic reputation 0 20 30 40 50 60 70 80 90 100 Acceptance rate We see the exp ected relationship of a higher p ercentage of students from the top of their high scho ol classes b eing asso ciated with b etter reputation and higher acceptance rate b eing asso ciated with worse reputation Each of the plots hints at p ossible nonlinearity though might logged reputation b e a b etter choice here c Jerey S Simono 2 1 Logged reputation 0 20 30 40 50 60 70 80 90 100 Acceptance rate Logs do esnt really seem to have help ed much A plot of reputation versus freshman retention rate lo oks particularly promising c Jerey S Simono 200 100 Academic reputation 0 60 70 80 90 100 Freshman retention rate Lets rst try a regression on all of the predictors after logging the long righttailed exp enditure variable Regression Analysis The regression equation is Academic reputation Top freshman Acceptance rate Logged expenditure Freshman retention rate Graduation rate Top school cases used cases contain missing values Predictor Coef StDev T P VIF Constant Top Acceptan Logged e Freshman Graduati Top s S RSq RSqadj c Jerey S Simono Analysis of Variance Source DF SS MS F P Regression Error Total Collinearity isnt a problem but several variables are apparently not needed There arent anyobvious problems with assumptions although the residuals versus tted values plot shows a bit of structure more on that later Residuals Versus the Fitted Values (response is Academic) 3 2 1 0 -1 Standardized Residual -2 0 100 200 Fitted Value c Jerey S Simono Normal Probability Plot of the Residuals (response is Academic) 3 2 1 0 -1 Standardized Residual -2 -2.5 -2.0 -1.5 -1.0 -0.5 0.0 0.5 1.0 1.5 2.0 2.5 Normal Score Here is the regression t on the variables that seem to help Regression Analysis The regression equation is Academic reputation Logged expenditure Freshman retention rate Top school cases used cases contain missing values Predictor Coef StDev T P VIF Constant Logged e Freshman Top s S RSq RSqadj c Jerey S Simono Analysis of Variance Source DF SS MS F P Regression Error Total The regression is highly signicant F p with the three predictors accounting for roughly of the variability in academic reputation Given freshman retention rate and top status are held xed multiplying exp enditure p er studentby is asso ciated with reputation status that is places higher holding exp enditure and top status xed a p oint increase in freshman retention rate is asso ciated with places higher in reputation and holding exp enditure and freshman retention rate xed a top scho ol is rated almost places higher than a nontop scho ol Regression diagnostics lo ok okay here the California Institute of Technology is a leverage p oint due to its high exp enditure p er student but removing it changes little Row University SRES HI COOK Andrews University Auburn University Baylor University Biola University Boston College Boston University Brandeis University California Institute of Technology Carnegie Mellon University Catholic University of America Clark University Clarkson University Dartmouth College DePaul University Duke University Duquesne University Florida Institute of Technology George Mason University George Washington University Georgetown University Georgia Institute of Technology Idaho State University Illinois Institute of Technology Indiana Univ of Pennsylvania Indiana UnivPurdue UnivIndianapolis Kansas State University Lehigh University Louisiana State Univ Baton Rouge Massachusetts Inst of Technology Montana State University North Carolina State UnivRaleigh Northeastern University Northwestern University Nova Southeastern University Ohio University c Jerey S Simono Oklahoma State University Oregon State University Pace University Pennsylvania State Univ San Diego State University St Johns University St Louis University Stevens Institute of Technology SUNYAlbany SUNYBinghamton Syracuse University Tennessee State University Texas AM UnivCollege Station Texas Womans University Tulane University U of North CarolinaChapel Hill Union Institute Univ of Arkansas Fayetteville Univ of CaliforniaLos Angeles Univ of CaliforniaSan Diego Univ of CaliforniaSanta Cruz Univ of Maryland Baltimore County Univ of MassachusettsLowell Univ of MinnesotaTwin Cities Univ of MissouriColumbia Univ of Southern California Univ of Southern Mississippi Univ of WisconsinMadison Univ of WisconsinMilwaukee University of CaliforniaBerkeley University of CaliforniaDavis University of Delaware University of Denver University of Florida University of Houston University of Iowa University of Louisville University of Memphis University of Miami University of Montana University of NevadaReno University of North Texas University of Northern Colorado University of Pennsylvania University of Pittsburgh University of Rochester University of San Francisco University of South Dakota University of South Florida University of Texas Dallas University of TexasAustin University of Vermont University of Virginia c Jerey S Simono Vanderbilt University Worcester Polytechnic Inst Yale University Here is output that gives the desired prediction interval for NYU The estimated academic reputation is whichistoohighNYUwas actually rated at Note that the prediction interval includes imp ossible negativevalues which reects the high variability and right tail in the reputation variable building
Details
-
File Typepdf
-
Upload Time-
-
Content LanguagesEnglish
-
Upload UserAnonymous/Not logged-in
-
File Pages33 Page
-
File Size-