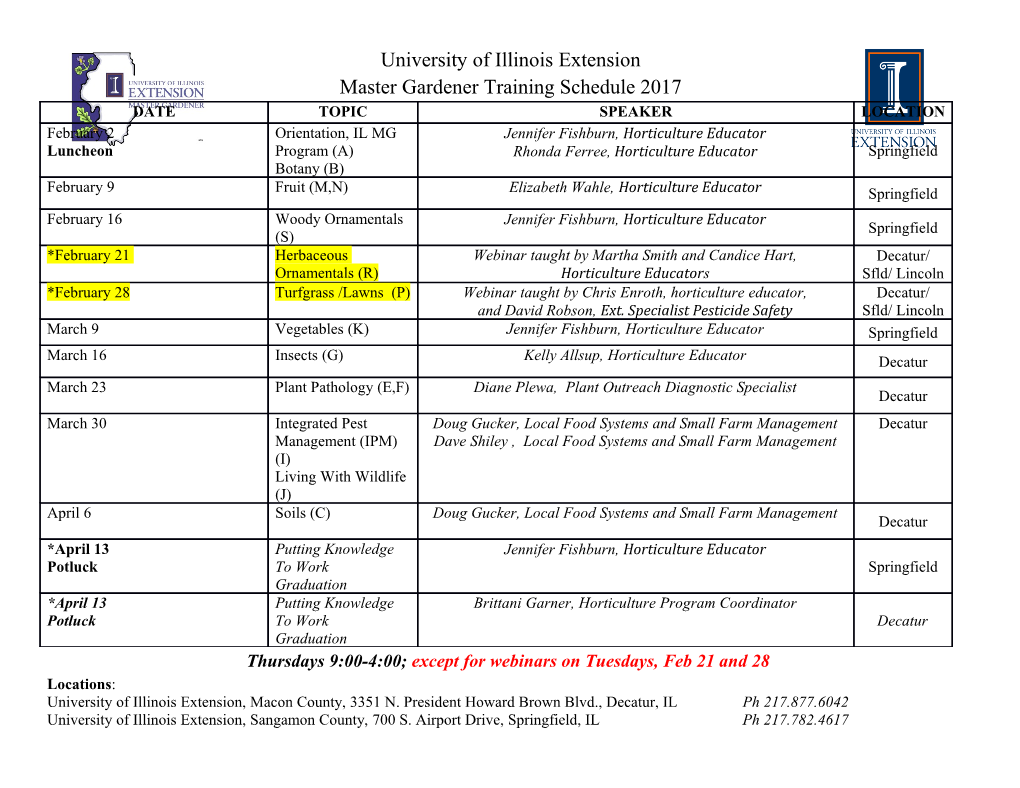
The Beta Moyal: An Useful Skew Distribution Gauss M. Cordeiro Departamento de Estatística e Informática, Universidade Federal Rural of Pernambuco, 52171-900, Recife, PE, Brazil e-mail:[email protected] Juvêncio S. Nobre Departamento de Estatística e Matemática Aplicada, Universidade Federal do Ceará, Fortaleza, Ceará, CE, Brazil e-mail:[email protected] Rodrigo R. Pescim Departamento de Ciências Exatas, Universidade de São Paulo, 13418-900, Piracicaba, SP, Brazil. e-mail:[email protected] Edwin M. M. Ortega Departamento de Ciências Exatas Universidade de São Paulo, 13418-900, Piracicaba, SP, Brazil. e-mail:[email protected] Abstract For the rst time, we propose a so-called beta Moyal distribution, which generalizes the Moyal distribution, and study its properties. We derive expansions for the cumulative distribution func- tion as a weighted power series of the Moyal cumulative distribution. We provide a comprehensive mathematical treatment of the new model and derive expansions for its moments, moment generating function, mean deviations, density function of the order statistics and their moments. We discuss maximum likelihood estimation of the model parameters. We illustrate the superiority of the new distribution as compared to the beta normal, skew-normal and Moyal distributions by means of two real data sets. Keywords: Expected information; Hazard rate function; Maximum likelihood estimation; Moyal dis- tribution; Survival function. 1 Introduction One major benet of the class of beta generalized distributions proposed by Eugene et al. (2002) is its ability of tting skewed data that can not be properly tted by existing distributions. Starting from a 1 parent cumulative distribution function (cdf) G(x), this class is dened by Z G(x) 1 a¡1 b¡1 F (x) = IG(x)(a; b) = ! (1 ¡ !) d!; (1) B(a; b) 0 where and are additional positive parameters, is the incomplete beta a b R Iy(a; b) = By(a; b)=B(a; b) function ratio, y a¡1 b¡1 is the incomplete beta function, By(a; b) = 0 w (1¡w) dw B(a; b) = ¡(a)¡(b)=¡(a+ b) is the beta function and ¡(a) is the gamma function. This class of generalized distributions has been receiving considerable attention over the last years in particular after the work of Jones (2004). Eugene et al. (2002), Nadarajah and Kotz (2004), Nadarajah and Gupta (2004), Nadarajah and Kotz (2005), Lee et al. (2007) and Akinsete et al. (2008) dened the beta normal, beta Gumbel, beta Fréchet, beta exponential, beta Weibull and beta Pareto distributions by taking G(x) to be the cdf of the normal, Gumbel, Fréchet, exponential, Weibull and Pareto distributions, respectively. More recently, Barreto-Souza et al. (2009) and Pescim et al. (2010) introduced the beta generalized exponential and the beta generalized half-normal distributions, respectively. The probability density function (pdf) corresponding to (1) is g(x) f(x) = G(x)a¡1f1 ¡ G(x)gb¡1; (2) B(a; b) where g(x) = dG(x)=dx is the parent density function. The density f(x) will be most tractable when both functions G(x) and g(x) have simple analytic expressions. Except for some special choices of these functions, f(x) will be dicult to deal with some generality. In this article, we introduce a four parameter distribution, so-called the beta Moyal (BMo) distri- bution, which contains as a sub-model the Moyal distribution. The BMo distribution is convenient for modeling comfortable upside-down bathtub-shaped failure rates and as a competitive model to the Moyal, half normal, beta normal, skew normal and Gumbel distributions. The article is organized as follows. In Section 2, we dene the BMo distribution, present some special sub-models and provide expansions for its distribution and density functions. Section 3 gives general expansions for the moments, moment generating function (mgf), mean deviations and Rényi entropy. In Section 4, we derive expansions for the moments of order statistics. Maximum likelihood estimation and inference issues are addressed in Section 5. Section 6 illustrates the importance of the BMo distribution through two real data sets. Finally, concluding remarks are given in Section 7. 2 Beta Moyal Distribution The Moyal distribution was proposed for J. E. Moyal (1955) as an approximation for the Landau distri- bution. It was also shown that it remains valid taking into account quantum resonance eects and details of atomic structure of the absorber. Let X be a random variable following the Moyal standard density function given by 1 gX (x) = p expf¡[x + exp(¡x)]=2g; ¡1 < x < 1: (3) 2¼ A location parameter ¹ and a scale factor σ can be introduced to dene the random variable Z = σX + ¹ having a Moyal distribution, say Mo(¹; σ), given by ½ · ½ ¾¸¾ 1 1 ³x ¡ ¹´ ³x ¡ ¹´ gZ (x) = p exp ¡ + exp ¡ ; (4) 2¼σ 2 σ σ where ¡1 < x; ¹ < 1 and σ > 0. The cumulative distribution function (cdf) corresponding to (4) is © £ ¡ ¢¤ª γ 1 ; 1 exp ¡ x¡¹ 2 2 σ (5) GZ (x) = 1 ¡ 1 ; ¡( 2 ) R which depends on the incomplete gamma function x ®¡1 ¡t . γ(®; x) = 0 t e dt 2 The Moyal distribution is a universal form for the energy loss by ionization for a fast charged particle and the number of ion pairs produced in this process. The cumulants of the standard Moyal distribution (3) are ³1´ ³1´ · = ¡ log(2) ¡ à = log(2) + γ and · = (¡1)nÃn¡1 = (n ¡ 1)!(2n ¡ 1)³(n); n ¸ 2; 1 2 n 2 where γ ¼ 0:57721 is Euler's constant, Ã(n) denotes polygamma functions and ³(:) is the Riemann's zeta function dened by Z X1 1 1 1 xu¡1 ³(u) = = dx for u > 1: ku ¡(u) ex ¡ 1 k=1 0 The moments can be easily obtained from these cumulants. Those of lower order are 0 ¹1 = E(X) = , 0 ¼2 , 0 and 0 7¼4 . For the distribution (4), log(2) + γ ¼ 1:27036 ¹2 = V ar(X) = 2 ¼ 4:9348 ¹3 = 14³3 ¹4 = 4 2 E(Z) = σE(X) + ¹, V ar(Z) = σ V ar(X) and, more generally, the central moments of Z (¹n;Z ) are n easily obtained from the central moments of X (¹n;X ) by ¹n;Z = σ ¹n;X for n ¸ 2. The characteristic function of the Moyal distribution (3) is 2¡it ³1 ´ Á(t) = E(eitz) = p ¡ ¡ it ; ¼ 2 where the gamma function ¡(:) with complex argument is valid when the real part of the argument is positive which is indeed true in the case at hand. The Moyal distribution can be dened in a nite interval. For this purpose, the transformation X = tan(Y ) yields the density function of Y as 1 1£ ¤ ¼ ¼ ¼(y) = p expf¡ tan(y) + expf¡ tan(y)g g; ¡ · y · : 2¼ cos2(y) 2 2 2 This density function has a maximum of about 0:91 and it is widely used to generate Moyal variates. We now introduce the four parameter BMo distribution by taking G(x) in (1) to be the cdf (5) of the Moyal distribution. The BMo cumulative function is given by x¡¹ γf 1 ; 1 exp[¡( )]g Z 1¡ 2 2 σ 1 ¡( 1 ) F (x) = 2 !a¡1(1 ¡ !)b¡1d!: (6) B(a; b) 0 Inserting (4) and (5) into (2) yields the BMo density function © £ © ª ¤ª ( © £ ¡ ¢¤ª)a¡1 exp ¡ 1 ( x¡¹ ) + exp ¡( x¡¹ ) γ 1 ; 1 exp ¡ x¡¹ f(x) = p2 σ ¡ ¢ σ 1 ¡ 2 2 σ £ 1 b¡1 ¡( 1 ) 2¼σB(a; b)¡ 2 2 ½ ½ · µ ¶¸¾¾ 1 1 x ¡ ¹ b¡1 γ ; exp ¡ ; ¡1 < x < 1; (7) 2 2 σ where ¡1 < ¹ < 1 is the location parameter, σ > 0 is the scale parameter and a > 0 and b > 0 are shape parameters. For a = b = 1, it reduces to the Moyal distribution. For ¹ = 0 and σ = 1, we obtain the standard BMo density function given by 8 h i9a¡1 exp(¡x) 1 < 1 = ½ · ¸¾b¡1 expf¡ [x + exp(¡x)]g γ 2 ; 2 1 exp(¡x) f(x) = p 2 ¡ ¢ 1 ¡ ¡ ¢ γ ; : (8) 1 b¡1 : ¡ 1 ; 2 2 2¼B(a; b)¡ 2 2 Plots of the density function (8) are illustrated in Figure 1 for selected parameter values, including the special case of the standard Moyal distribution. 3 a=1; b=0.3 a=0.3; b=1 a=1; b=0.7 a=1; b=1 a=1; b=2 a=5; b=1 a=1; b=3 a=15; b=1 a=1; b=5 a=30; b=1 f(x) f(x) 0.00 0.05 0.10 0.15 0.20 0.00 0.05 0.10 0.15 0.20 0.25 0.30 0.35 0 5 10 15 0 5 10 15 x x Figure 1: Plots of the density function (8) for some parameter values. The hazard rate function corresponding to (7) becomes ½ ¾a¡1 © £ © ª¤ª γf 1 ; 1 exp[¡( x¡¹ )]g p 1 1 x¡¹ x¡¹ 2 2 σ exp ¡ 2 ( σ ) + exp ¡( σ ) 1 ¡ 1 2¼σ ¡( 2 ) h(x) = 8 9 : (9) £ ¡ ¢¤ < = © © £ ¡ ¢¤ªª 1 b¡1 1 1 x¡¹ ¡(b¡1) ¡ B(a; b) 1 ¡ I 1 1 x¡¹ (a; b) γ ; exp ¡ 2 γf ; exp[¡( )]g 2 2 σ : 1¡ 2 2 σ ; ¡( 1 ) 2 Plots of some standard BMo hazard rate functions for selected parameter values are given in Figure 2. If X is a random variable with density function (7), we write X »BMo(a; b; ¹; σ). The BMo distribu- tion is easily simulated from F (x) in (6) as follows: if V has a beta distribution with parameters a and b, then the solution of the nonlinear equation n o X ¡ ¹ £ ¤2 = ¡ log 2 erf¡1(1 ¡ V ) σ ¡ ¢ p p yields the BMo distribution, where 1 erf and the error function erf is de- (a; b; ¹; σ) γ 2 ; x = ¼ ( x) (x) R x 2 ned by erf p2 ¡t .
Details
-
File Typepdf
-
Upload Time-
-
Content LanguagesEnglish
-
Upload UserAnonymous/Not logged-in
-
File Pages20 Page
-
File Size-