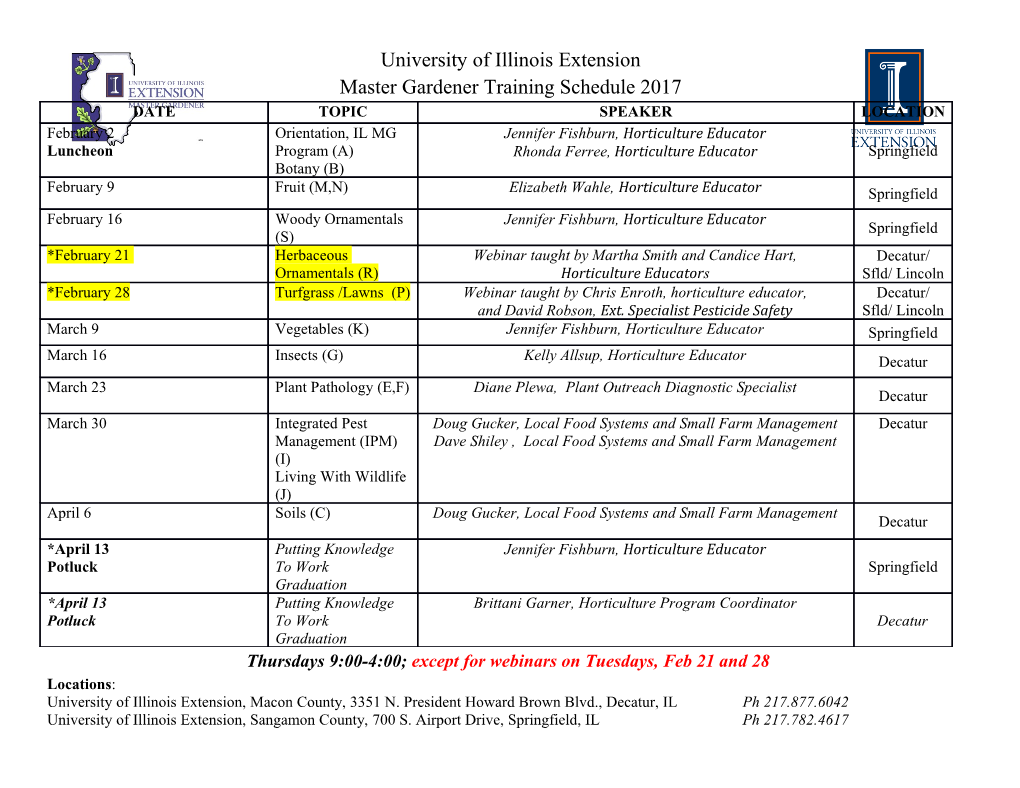
SIVO: Semantically Informed Visual Odometry and Mapping by Pranav Ganti A thesis presented to the University of Waterloo in fulfillment of the thesis requirement for the degree of Master of Applied Science in Mechanical and Mechatronics Engineering Waterloo, Ontario, Canada, 2018 c Pranav Ganti 2018 I hereby declare that I am the sole author of this thesis. This is a true copy of the thesis, including any required final revisions, as accepted by my examiners. I understand that my thesis may be made electronically available to the public. ii Abstract Accurate localization is a requirement for any autonomous mobile robot. In recent years, cameras have proven to be a reliable, cheap, and effective sensor to achieve this goal. Visual simultaneous localization and mapping (SLAM) algorithms determine camera motion by tracking the motion of reference points from the scene. However, these references must be static, as well as viewpoint, scale, and rotation invariant in order to ensure accurate localization. This is especially paramount for long-term robot operation, where we require our references to be stable over long durations and also require careful point selection to maintain the runtime and storage complexity of the algorithm while the robot navigates through its environment. In this thesis, we present SIVO (Semantically Informed Visual Odometry and Mapping), a novel feature selection method for visual SLAM which incorporates machine learning and neural network uncertainty into an information-theoretic approach to feature selection. The emergence of deep learning techniques has resulted in remarkable advances in scene understanding, and our method supplements traditional visual SLAM with this contextual knowledge. Our algorithm selects points which provide significant information to reduce the uncertainty of the state estimate while ensuring that the feature is detected to be a static object repeatedly, with a high confidence. This is done by evaluating the reduction in Shannon entropy between the current state entropy, and the joint entropy of the state given the addition of the new feature with the classification entropy of the feature from a Bayesian neural network. Our method is evaluated against ORB SLAM2 and the ground truth of the KITTI odometry dataset. Overall, SIVO performs comparably to ORB SLAM2 (average of 0.17% translation error difference, 6:2×10−5deg/m rotation error difference) while removing 69% of the map points on average. As the reference points selected are from static objects (building, traffic signs, etc.), the map generated using our algorithm is suitable for long- term localization. iii Acknowledgements First and foremost, I would like to thank my supervisor, Dr. Steven L. Waslander, for his guidance, encouragement, and support throughout the development of this work. Thank you for giving me an opportunity to develop skills in a field I knew little about, and for allowing me to explore areas I was passionate about as I gained experience in this amazing field. My experience at the WAVELab has helped me grow immensely both personally and professionally. Thanks to all of my colleagues at the WAVELab for making the last two years fun, even through the stress swirling around us. I would like to thank my awesome roommates, Ben, Leo, Jung, and Sean, for making sure we could never separate our work life from our home life. Thank you to Mike, Chris, and Nima for the fun conversations and advice over the countless journeys to get lunch and coffee. Thank you to Stan for convincing me to join the WAVELab and for the advice and guidance that followed. Lastly, I would like to thank Arun and Jason for not only sharing their SLAM secrets, but also for always being willing to take a break so that we could watch the Raptors lose. I would like to thank Jordan and Jonathan for their help preparing the training data and testing various configurations of the neural network used in this project. Thank you Rebecca, for your love and support from across the country. I could not have achieved this without your unconditional support and encouragement. Lastly, I would like to thank my parents for, well, everything. Thank you for your immeasurable support, patience, guidance, dedication, love, encouragement, and advice. Thank you for all of the sacrifices you have made and for giving me the opportunity to pursue my goals and dreams. iv Dedication This thesis is dedicated to my parents. v Table of Contents List of Tablesx List of Figures xi 1 Introduction1 1.1 Motivation....................................2 1.2 Related Works..................................4 1.2.1 Information-Theoretic Approaches to SLAM.............4 1.2.2 Semantic SLAM.............................7 1.3 Statement of Contributions.......................... 11 1.4 Thesis Organization............................... 12 2 Background 13 2.1 Notation..................................... 13 2.1.1 Coordinate Frames........................... 13 2.1.2 Homogeneous Coordinates....................... 16 2.2 Gaussian Distributions and Gaussian Processes............... 16 2.2.1 Gaussian Random Variables...................... 16 2.2.2 Gaussian Processes........................... 18 2.3 Computer Vision................................ 19 2.3.1 Camera Projection........................... 19 vi 2.3.2 Stereo Projection Measurement Model................ 22 2.4 ORB SLAM2.................................. 22 2.5 Semantic Segmentation............................. 23 2.5.1 Convolutional Neural Networks (CNNs)............... 24 2.5.2 SegNet.................................. 25 2.6 Uncertainty in Machine Learning....................... 25 2.6.1 Gaussian Processes and Bayesian Neural Networks......... 26 2.6.2 Dropout................................. 26 2.6.3 Classification Loss........................... 28 2.6.4 Gaussian Processes for Machine Learning............... 28 2.6.5 Bayesian Neural Networks....................... 29 2.6.6 Variational Inference.......................... 30 2.6.7 Approximating the Bayesian Neural Network using Variational In- ference.................................. 30 2.6.8 Bayesian SegNet............................ 31 2.7 Information Theory............................... 32 2.7.1 Entropy................................. 32 2.7.2 Joint Entropy.............................. 33 2.7.3 Conditional Entropy.......................... 33 2.7.4 Entropy of a Gaussian Random Variable............... 33 2.7.5 Relative Entropy and Mutual Information.............. 34 2.7.6 Relationship Between Entropy and Mutual Information....... 35 2.7.7 Mutual Information of a Multivariate Gaussian........... 36 2.7.8 Uncertainty in Classification Results for a Bayesian Neural Network 36 3 SIVO Feature Selection Criteria 38 3.1 Information-Theoretic Feature Selection Methods.............. 38 3.2 SIVO Feature Selection............................. 40 vii 4 Results 46 4.1 Implementation Details............................. 46 4.1.1 Training................................. 46 4.1.2 Network Inference and Hardware................... 49 4.2 Experiments and Notation........................... 49 4.3 Results on KITTI Trajectories......................... 50 4.3.1 Summary................................ 50 4.3.2 Trajectory 09.............................. 52 4.3.3 Trajectory 00.............................. 56 4.3.4 Trajectory 08.............................. 59 4.3.5 Trajectory 01.............................. 62 4.3.6 Discussion................................ 65 5 Conclusion 69 5.1 Future Work................................... 70 References 72 APPENDICES 79 A Fundamentals 80 A.1 Lie Groups and Lie Algebra.......................... 80 A.1.1 SO(3): Special Orthogonal Lie Group in 3D............. 81 A.1.2 SE(3): Special Euclidean Lie Group in 3D.............. 83 A.2 Probability................................... 86 A.2.1 Notation and Random Variables.................... 86 A.2.2 Mean, Variance, and Covariance.................... 87 A.2.3 Joint Probability............................ 88 A.2.4 Conditional Probability........................ 88 viii A.2.5 Likelihood and Posterior........................ 89 A.3 Computer Vision................................ 90 A.3.1 Oriented Fast and Rotated BRIEF (ORB) Features......... 90 B SIVO Jacobians 92 B.1 Jacobian of Rectified Stereo Projection.................... 92 B.2 Jacobian of the Transformed Point...................... 93 B.3 Full Projection Jacobian............................ 93 B.4 Motion Model Propagation........................... 94 B.4.1 Jacobian of Motion Model Propagation................ 95 C Detailed Results on KITTI Trajectories 97 C.1 Trajectory 02.................................. 98 C.2 Trajectory 03.................................. 101 C.3 Trajectory 04.................................. 104 C.4 Trajectory 05.................................. 107 C.5 Trajectory 06.................................. 110 C.6 Trajectory 07.................................. 112 C.7 Trajectory 10.................................. 115 ix List of Tables 4.1 Translation error, rotation error, and map reduction for ORB SLAM2 and SIVO on the KITTI dataset........................... 51 4.2 Keyframe and map point comparisons for KITTI Trajectory 09....... 53 4.3 Keyframe and map point comparisons for KITTI Trajectory 00....... 57 4.4 Keyframe and map point comparisons for KITTI Trajectory 08....... 60 4.5 Keyframe and map point comparisons for KITTI Trajectory 01....... 63 C.1 Keyframe and map point comparisons for KITTI Trajectory 02....... 99 C.2 Keyframe and map point comparisons
Details
-
File Typepdf
-
Upload Time-
-
Content LanguagesEnglish
-
Upload UserAnonymous/Not logged-in
-
File Pages130 Page
-
File Size-