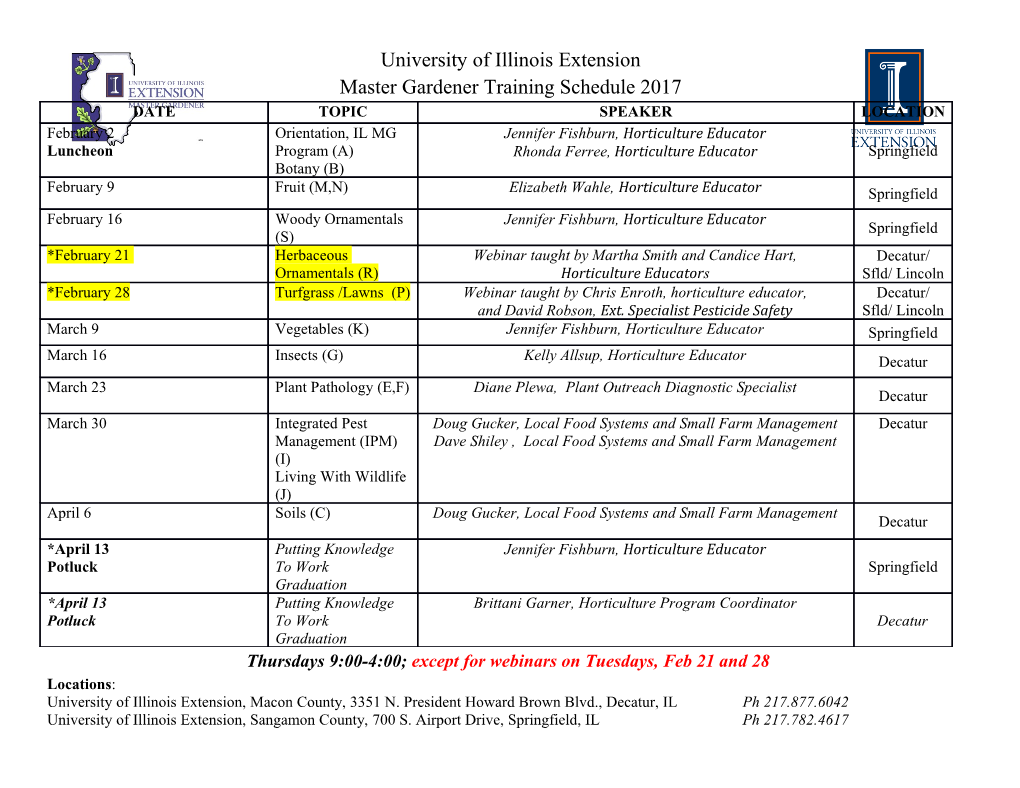
Fardo et al. BMC Proceedings 2011, 5(Suppl 9):S28 http://www.biomedcentral.com/1753-6561/5/S9/S28 PROCEEDINGS Open Access Exploration and comparison of methods for combining population- and family-based genetic association using the Genetic Analysis Workshop 17 mini-exome David W Fardo1,2,3*, Anthony R Druen4, Jinze Liu3,4, Lucia Mirea5,6, Claire Infante-Rivard7, Patrick Breheny1 From Genetic Analysis Workshop 17 Boston, MA, USA. 13-16 October 2010 Abstract We examine the performance of various methods for combining family- and population-based genetic association data. Several approaches have been proposed for situations in which information is collected from both a subset of unrelated subjects and a subset of family members. Analyzing these samples separately is known to be inefficient, and it is important to determine the scenarios for which differing methods perform well. Others have investigated this question; however, no extensive simulations have been conducted, nor have these methods been applied to mini-exome-style data such as that provided by Genetic Analysis Workshop 17. We quantify the empirical power and false-positive rates for three existing methods applied to the Genetic Analysis Workshop 17 mini-exome data and compare relative performance. We use knowledge of the underlying data simulation model to make these assessments. Background Because analyzing the disparate types of data in isolation Study designs for genetic association studies fall into two most often results in nonoptimal statistical power, investi- broad categories: (1) population-based studies that recruit gators have proposed several methods for efficiently com- unrelated individuals and (2) family-based studies that col- bining these data. We briefly summarize three methods to lect some number of related pedigrees. Often, both study be applied to the Genetic Analysis Workshop 17 designs are used for a particular investigation. For exam- (GAW17) data in the Methods section. Each approach is ple, when a linkage study has been performed and family distinguished by the study designs for which it is appropri- data are collected, follow-up analysis can include associa- ate, the assumptions necessary for valid inference, and the tion using a new unrelated study population. The analytic handling of population stratification (whether it is formally methods appropriate for either design differ, thus making or informally tested or whether it is taken into account by difficult the aggregation of the association metrics across means of adjustments). Operationally, these methods are the study designs. Heuristically, population-based metrics distinguishable by computation and implementation con- attempt to quantify a measure of correlation or association siderations and by empirical performance. We assess the between some function of genotype at a given marker and performance in this paper. Other researchers have investi- the disease phenotype, whereas family-based association gated the question of relative performance [1]; however, measures use properties of Mendelian transmissions from no simulations have been conducted for comparison. parents to offspring and are inherently conditional. An important consideration to keep in mind through- out this investigation is the underlying causal model that * Correspondence: [email protected] was used to generate the GAW17 data [2]. First, rather 1 Department of Biostatistics, University of Kentucky College of Public Health, than reflecting the common disease/common variant 121 Washington Avenue, Lexington, KY 40536, USA Full list of author information is available at the end of the article hypothesis that the established methods presented © 2011 Fardo et al; licensee BioMed Central Ltd. This is an open access article distributed under the terms of the Creative Commons Attribution License (http://creativecommons.org/licenses/by/2.0), which permits unrestricted use, distribution, and reproduction in any medium, provided the original work is properly cited. Fardo et al. BMC Proceedings 2011, 5(Suppl 9):S28 Page 2 of 6 http://www.biomedcentral.com/1753-6561/5/S9/S28 address, the data-generating mechanism used was consis- Because the approach by Chen and Lin [7] is not tent with the multiple rare variant or the common dis- immediately generalizable to pedigrees, we extracted ease/rare variant (CDRV) hypothesis, which suggests that nuclear families and then sampled 194 trios from the common disease susceptibility is garnered through multi- nuclear families to provide a uniform comparison ple rare variants with moderate to high penetrance. Intui- between the methods. These sampled data (697 unre- tively, the current methods do not perform well in lated case or control individuals and 582 family mem- identifying rare single-nucleotide polymorphisms (SNPs); bers from the 194 trios) are used for our comparisons. in this paper we intend to assess this performance and to We assume an additive mode of inheritance throughout. motivate possible modifications that would be successful when the CDRV hypothesis is true. In addition, the dis- Chen and Lin’s method ease was simulated to have ≫ 30% prevalence, which vio- Chen and Lin’s [7] approach uses the conditional on lates the often-invoked rare disease assumption. parental genotypes (CPG) approach of Schaid and Som- mer [22] to construct the likelihood of the case-trio Methods samples. An estimate for the RR is obtained from the ˆ The first attempts to combine population- and family- CPG likelihood and is denoted b trio .Thisestimateis based association data were developed by Nagelkerke et al. then compared to a traditional logistic regression esti- ˆ [3], who used a likelihood framework to combine case- mate of the genotype log odds ratio, bCC ,usingthe control data with family data by exploiting the likelihood case-control sample, which is composed of case-trio formulation [4] of the transmission disequilibrium test probands and the unrelated control subjects. Chen and (TDT) [5]. This approach assumes Hardy-Weinberg equi- Lin use a Wald-type test to determine whether the effect librium (HWE), random mating, and a multiplicative estimates are consistent. If this test is not rejected, a model of allelic effect. Although no formal test of the weighted least-squares estimator for the combined appropriateness of combining the two types of data has genetic effect is then constructed for inference as: been developed, we discuss ad hoc procedures. ˆˆ=+ ˆ Epstein et al. [6] generalized this work by relaxing the bbWW12trio bCC (1) assumptions of HWE, random mating, and the assumed multiplicative mode of inheritance. In addition, they where W1 and W2 are weights derived from linear described a formal test for the appropriateness of combin- model theory assuming the parameter estimates follow a ing case-control and case-trio data by comparing genotype multivariate normal distribution (see Chen and Lin [7] relative risk (RR) estimates from between-individual and for details). Here, the assumptions of a rare disease and within-family analyses, respectively. The proposed two- no population stratification are necessary for validity. stage procedure facilitates valid model selection in the pre- However, the test used to reject the appropriateness of sence of population stratification. Further extensions of combining the RR estimates is not well powered, as evi- this approach were made by Chen and Lin [7]. Their denced by our simulations, which often did not confer method uses weighted least squares to aggregate the dispa- sufficient evidence to reject the null hypothesis of para- rate RRs and requires no assumptions for mating-type meter equivalence even though the simulated disease is distributions. not, in fact, rare—a necessary condition for such equiva- Epstein et al.’s and Chen and Lin’s methods rely on two lence. This method was designed for case-trio and unre- strong assumptions: a rare disease and the absence of lated control subjects; however, in our analyses control population stratification. Later work has been targeted at offspring from the control trios are added to the case- both relaxing the rare disease assumption and adjusting control subsample. for population stratification. Zhu et al. [8] used a princi- pal components strategy to adjust for population stratifi- Zhu et al.’s method cation and to aggregate families and case-control samples In Zhu et al.’s [8] approach, principal components are by means of a linear regression framework. Within-family calculated from the genotypes of all unrelated indivi- correlations were empirically estimated from the data duals (trio parents and unrelated case and control sub- andincorporatedintothevariance of the test statistic. jects), and both the genotypes and the phenotypes of Zhang et al. [9] proposed a similar method in which they these individuals are then separately regressed on the defined a score test and used generalized estimating principal components. The resulting linear regression equations [10] to account for familial correlation. Their parameter estimates are used to calculate genotypic and method can be more easily applied to multivariate out- phenotypic residuals, yij and gij , respectively, where i comes. Other useful approaches, some with a focus on indexes families and j indexes individuals within a genome-wide association, have been proposed but are family. The covariance between these residuals is mea- not evaluated here [11-21]. sured as: Fardo et al. BMC Proceedings 2011, 5(Suppl 9):S28 Page 3 of 6 http://www.biomedcentral.com/1753-6561/5/S9/S28 N ki adjusts for population stratification in family data [24] is gyij ij T = ∑∑ , (2) used within the set of related individuals to define: N i j== 11 T ⎛ gg+ ⎞ =−− im if Ry∑ ()ij m ⎜ gij ⎟, (4) where N is the number of families, ki is the number of ⎝ 2 ⎠ ij individuals in the ith family, and NT is the total number of individuals. Within-family correlations are taken into where g and g are the mother’sandfather’sgeno- account in the calculation of the variance of T to con- im if types in the ith family, respectively. The score Z = U + struct a Wald test.
Details
-
File Typepdf
-
Upload Time-
-
Content LanguagesEnglish
-
Upload UserAnonymous/Not logged-in
-
File Pages6 Page
-
File Size-