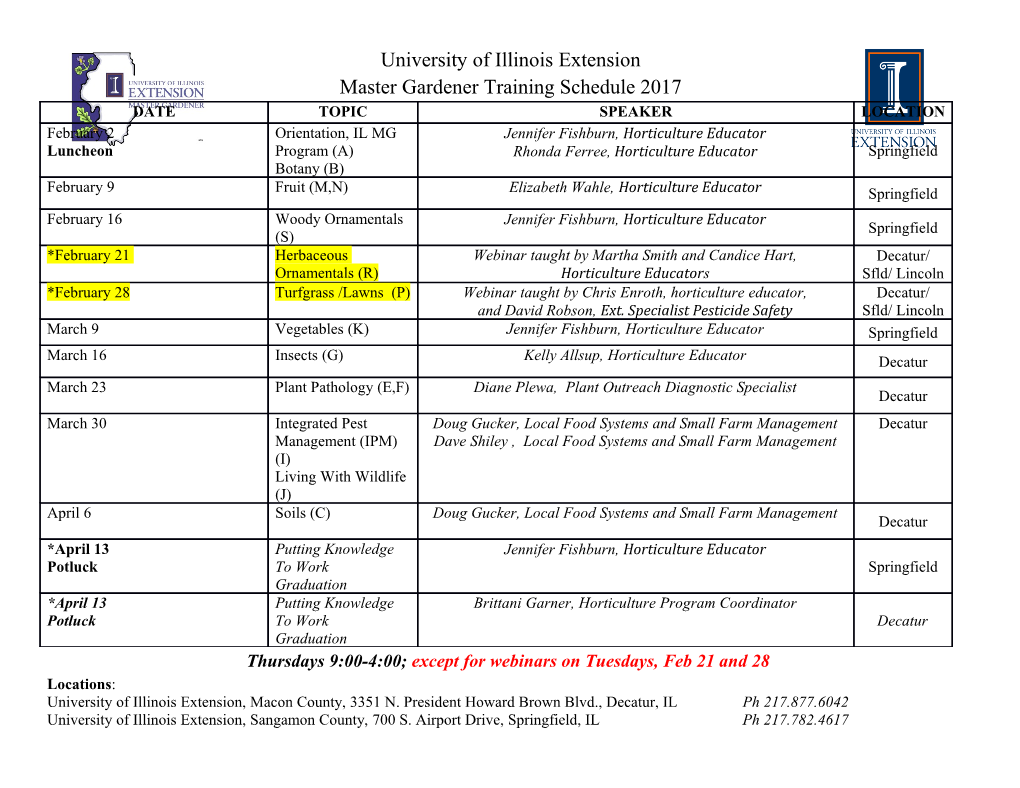
Advances in Research 11(5): 1-13, 2017; Article no.AIR.35391 ISSN: 2348-0394, NLM ID: 101666096 Evaluation of Variation in Macronutrients of Soils in Harda District, Madhya Pradesh, India - A Geostatistical Approach Subhash 1,2* , G. S. Tagore 1, Debabrata Nath 2, M. Mohanty 2 and Nishant K. Sinha 2 1Department of Soil Science and Agricultural Chemistry, Jawaharlal Nehru Krishi Vishwa Vidyalaya, Jabalpur - 482004 (M.P.), India. 2Indian Institute of Soil Science, Bhopal, 462001, India. Authors’ contributions This work was carried out in collaboration between all authors. Author GST designed the study, performed the statistical analysis, wrote the protocol and wrote the first draft of the manuscript. Authors Subhash and GST managed the analyses of the study. Authors Subhash and DN managed the literature searches and communicate with journal. Authors MM and NKS evaluated and edited the manuscript. All authors read and approved the final manuscript. Article Information DOI: 10.9734/AIR/2017/35391 Editor(s): (1) S. Srinivasa Rao, Department of Chemistry, V. R. Siddhartha Engineering College, Andhra Pradesh, India. Reviewers: (1) Sukhwinder Bali, University of Maine Cooperative Extension, USA. (2) Seref Kilic, Ardahan University, Turkey. (3) Ahmed Raissouni, University of Abdelmalek Essaâdi, Morocco. (4) Stanley U. Onwudike, Federal University of Technology, Nigeria. Complete Peer review History: http://www.sciencedomain.org/review-history/21013 Received 11 th July 2017 st Original Research Article Accepted 1 September 2017 Published 15th September 2017 ABSTRACT GPS based three hundred and three surface soil samples (0-15 cm) were collected from dominant cropping system and analyzed for different soil characteristics in laboratory using standard procedures. The results were statistically interpreted that the N, P, K, and S were found to be deficient in 56.77, 31.68, 6.6 and 52.48 percent soil samples, respectively. Geo-statistical results revealed that the exponential model was found best fit for available N, P, K, and S. Spatial distribution maps showed that soil pH, EC, organic carbon, calcium carbonate, N, P, K, and S spatially varied and were deficient in Hundia, Timarani, Khirkiya and Sirrali. These maps will be helpful for farmer’s to decide the quantity of fertilizer to be added to soil to improve fertility status for sustainable crop production and environmental protection. _____________________________________________________________________________________________________ *Corresponding author: E-mail: [email protected]; Subhash et al.; AIR, 11(5): 1-13, 2017; Article no.AIR.35391 Keywords: GIS; GPS; macronutrients; mapping; semi-variogram; variability. 1. INTRODUCTION minimum up to 12°C and an average annual rainfall of 1021.84 mm. The major crops grown in Increase in human population and disturbance the study area are soybean, wheat, summer on the earth’s ecosystem to produce food and mungbean, chickpea, sugarcane and vegetables. fiber will place greater demand on soils to supply The soil map of NBSSLUP Nagpur showed the essential nutrients. In India, continuous cropping highest area is dominated by Vertisols followed for enhanced yield removes substantial by Inceptisols and Entisols. amounts of nutrients from the soil. Amongst, macronutrients are required in large quantities for 2.2 Land Use and Cropping Pattern growth, increase plant productivity and yield, but these nutrients were deficient in the majority of The Land use map was prepared by using Indian soybean and chickpea growing areas and where remote-sensing satellite-P6, linear imaging self- sulphur free fertilizer has been used. Soil fertility scanning satellite-III (IRS-P6, LISS-III) satellite maps are meant for highlighting the nutrient imagery. The Satellite data has the needs, based on fertility status of soils to realize characteristics of 23.5 m spatial resolution, four good crop yields. spectral channels green (0.52 µ-0.59 µ), red (0.62 µ-0.68 µ), NIR (0.77 µ-0.86 µ), and SWIR Information related to spatial variability and (1.55 µ-1.70 µ) and five days temporal resolution distribution of soil properties is critical for farmers with 141 km swath. attempting to increase the efficiency of fertilizers and crop productivity [1]. Geo-statistics has been The survey of India topographical maps used extensively to characterize the spatial (1:50000), the digital map of soil and satellite variability of soil attributes due to its ability to imagery as a secondary data was used from quantify and reducing sampling uncertainties and internet. For image processing/analysis ERDAS minimizing investigation costs [2]. [3] and [4] Imagine 9.3.1 was used. On the basis of the characterized the spatial variability of soil information obtained by the identification of the physical, [5] studied the chemical properties and physical characteristics from the imagery and microbiological properties [6]. their verification in the field was done. Classic statistic method is needed for extensive The major land-use/land-cover categories were sampling to decline variation correlation. Despite identified and mapped (Fig. 2). From the maps, it high costs spent in this method, the results are is evident that the major area 2082.20 sq km, limited only on mean value for specific classes. which was accounted to 62.52%, is occupied by Some studies were undertaken in traditional cultivated land. On the basis of information survey, mapping of soil fertility and large obtained from every sampling site and local numbers of samples analyzed, but the latitude agriculture department, the soybean based and longitude of sampling sites are often time cropping pattern is dominant existing cropping missing. Hence, an attempt was made to use system viz., soybean-wheat, soybean-wheat- GPS receivers to map the exact location of summer mungbean, soybean-chickpea and sampling sites which can be used as input data soybean-fallow. Sugarcane and horticultural in GIS and is used to run the queries to know crop/orchards-spices crop/vegetables land uses various properties of soil. were observed in study area. Based on the interpretation of classified image and calculated 2. MATERIALS AND METHODS area statistics the forest was classified in two categories; dense 20.0% (666.0 sq km) and open 2.1 Description Study Area 6.96% (231.90 sq km). Geographically, Harda district situated between Other land use categories are built-up (52.83 sq 21° 53’ to 22° 36’ North latitude and 76° 47’ to km) which accounted by 1.59 percent 77° 30’ East longitude with an area of 3330 sq represented to Harda city and some village’s km. It is located in the Narmada river valley and settlements. Water bodies were occupied (68.25 the Narmada forms the northern district sq km) 2.05% of total geographical area. The boundary. Administratively, the district divided classified data showed the wasteland in four into six blocks, Rahatgaon, Harda, Khirkiya, category i.e., gullied/ravenous land 0.05% (1.82 Hundia, Sirrali and Timarani (Fig. 1). The district sq km), sandy area-riverine,0.10% (3.17sq km), feels maximum temperature up to 47°C and dense scrub1.28% (42.72 sq km) and open scrub 2 Subhash et al.; AIR, 11(5): 1-13, 2017; Article no.AIR.35391 1.80%(59.89 sq km) and minimum area covered classified as low (<1.67), medium (1.67 to 2.33) by mining 0.01% (0.17 sq km) of the TGA. and high (>2.33). 2.3 Soil Sampling, Processing and Their 2.4 Geo-statistical Analysis Analysis It was necessary to normalize the data prior to Sampling sites were randomly distributed over the geo-statistical analysis because of high agricultural land by considering of topography skewness and the presence of outliers. and heterogeneity of the soil type. GPS based Logarithmic transformations were selected to three hundred and three soil samples (0 to 15 normalize the dataset. When skewness cm) were collected from farmer’s field during the coefficient is lower than 0.5 there is no need to off season of 2014. Soil samples were dried and convert data, but if this coefficient is between 0.5 crushed with the help of wooden rod and passed and 1, and more than 1 for normalizing data through 2 mm sieve and then used for analysis of square root and logarithm must be used, soil pH, EC, OC and CaCO 3. The available N, P, respectively [12]. When this ratio is smaller than and K were analyzed using [7,8] and [9] 0.25 the concerned parameter has a strong respectively. The available S was determined by spatial steal structure, between 0.25 ‐0.75 spatial the turbidimetric method [10]. The nutrient index structure is middle, and when it is greater than (NI) was calculated according to by [11] and 0.75 spatial structures is weak [13]. Fig. 1. Location map of study area 3 Subhash et al.; AIR, 11(5): 1-13, 2017; Article no.AIR.35391 Fig. 2. Land use and its spatial distribution in Harda district If the data distributions are largely deviate from a ʚ#ʛ normal distribution, data transformations are ² 1 often performed to reduce the influence of ͭʚℎʛ = ȕʞzʚxΗʛ − zʚxΗ + hʛ ʟ , extreme values on spatial analysis [14]. 2͈ʚℎʛ $Ͱͥ Next, this was fitted with a theoretical model, f(x) =ln(x) λ=0, such as Exponential, Spherical and Gaussian models [15]. Choice of the best-fitted model was Where f(x) is the transformed value and x is the based on the lowest residual sum of square value to be transformed. For a given data set (x 1, (RSS) and the largest coefficient of determination 2 x2,…..x n), the parameter is estimated based on (R ). The model provided information about the the assumption that the transformed values spatial structure as well as the input parameters (y 1,y 2,…..y n) are normally distributed.
Details
-
File Typepdf
-
Upload Time-
-
Content LanguagesEnglish
-
Upload UserAnonymous/Not logged-in
-
File Pages13 Page
-
File Size-