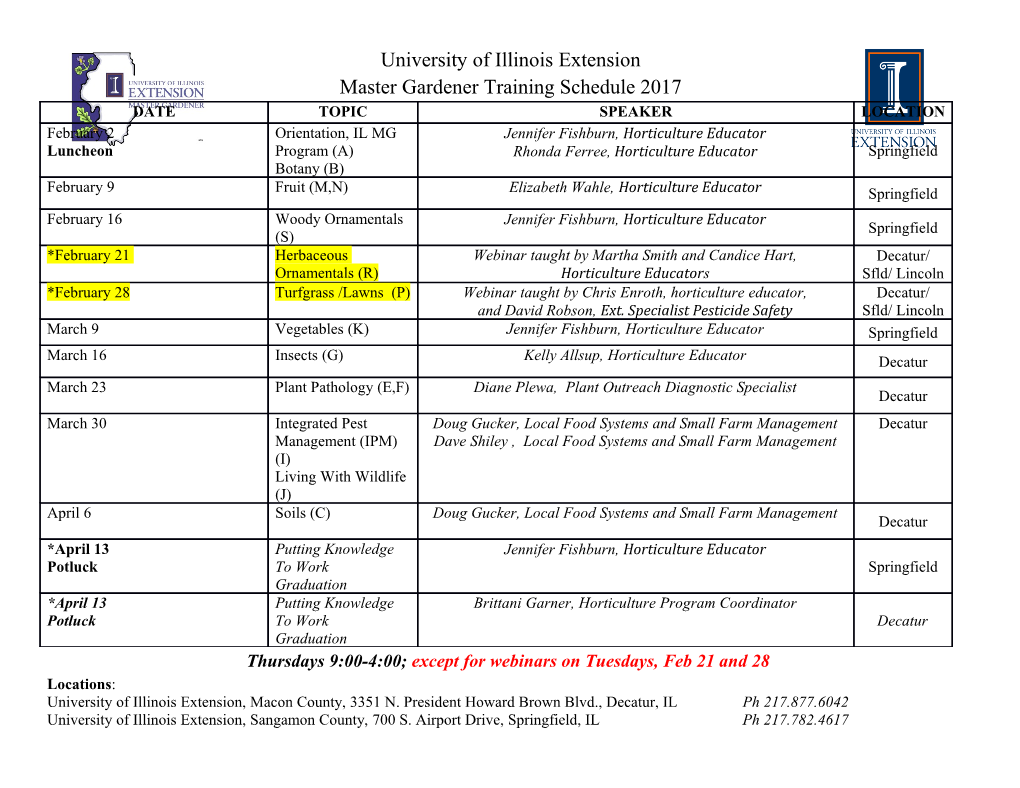
medRxiv preprint doi: https://doi.org/10.1101/2021.05.22.21257482; this version posted May 23, 2021. The copyright holder for this preprint (which was not certified by peer review) is the author/funder, who has granted medRxiv a license to display the preprint in perpetuity. It is made available under a CC-BY-ND 4.0 International license . 1 Multi-omics analysis reveals the impact of microbiota on host metabolism in hepatic steatosis Mujdat Zeybel,1,2,3,# Muhammad Arif,4,# Xiangyu Li,4,# Ozlem Altay,4 Mengnan Shi,4 Murat Akyildiz,1 Burcin Saglam,1 Mehmet Gokhan Gonenli,1 Buket Yigit,1 Burge Ulukan,1 Dilek Ural,5 Saeed Shoaie,4,7 Hasan Turkez,8 Jens Nielsen,9 Cheng Zhang,4,6 Mathias Uhlén,4 Jan Borén,10,* Adil Mardinoglu4,7,* 1Department of Gastroenterology and Hepatology, School of Medicine, Koç University, Istanbul, Turkey 2NIHR Nottingham Biomedical Research Centre, Nottingham University Hospitals NHS Trust & University of Nottingham, Nottingham, UK 3Nottingham Digestive Diseases Centre, School of Medicine, University of Nottingham, Nottingham, UK 4Science for Life Laboratory, KTH - Royal Institute of Technology, Stockholm, Sweden 5School of Medicine, Koç University, Istanbul, Turkey 6 Key Laboratory of Advanced Drug Preparation Technologies, Ministry of Education, School of Pharmaceutical Sciences, Zhengzhou University, Zhengzhou, Henan Province, 450001, China 7Centre for Host-Microbiome Interactions, Faculty of Dentistry, Oral & Craniofacial Sciences, King's College London, London, United Kingdom 8Department of Medical Biology, Faculty of Medicine, Atatürk University, Erzurum, Turkey 9Department of Biology and Biological Engineering, Chalmers University of Technology, Gothenburg, Sweden 10Department of Molecular and Clinical Medicine, University of Gothenburg and Sahlgrenska University Hospital Gothenburg, Sweden *Correspondence: [email protected]; [email protected] Emails: [email protected]; [email protected]; [email protected]; [email protected]; [email protected]; [email protected]; [email protected]; [email protected]; [email protected]; [email protected]; [email protected]; [email protected]; [email protected]; [email protected]; [email protected]; [email protected]; [email protected]; [email protected] NOTE: This preprint reports new research that has not been certified by peer review and should not be used to guide clinical practice. medRxiv preprint doi: https://doi.org/10.1101/2021.05.22.21257482; this version posted May 23, 2021. The copyright holder for this preprint (which was not certified by peer review) is the author/funder, who has granted medRxiv a license to display the preprint in perpetuity. It is made available under a CC-BY-ND 4.0 International license . 2 Keywords: Non-alcoholic fatty liver disease: Multi-omics analysis; Metabolomics, Proteomics; gut and oral metagenomics; Systems Biology; Systems Medicine Abstract Non-alcoholic fatty liver disease (NAFLD) is a complex disease involving alterations in multiple biological processes regulated by the interactions between obesity, genetic background and environmental factors including the microbiome. To decipher hepatic steatosis (HS) pathogenesis by excluding critical confounding factors including genetic variants, obesity and diabetes, we characterized 56 heterogeneous NAFLD patients by generating multi-omics data including oral and gut metagenomics as well as plasma metabolomics and inflammatory proteomics data. We explored the dysbiosis in the oral and gut microbiome and revealed host-microbiome interactions based on global metabolic and inflammatory processes. We integrated this multi-omics data using the biological network and identified HS's key features using multi-omics data. We finally predicted HS using these key features and validated our findings in a validation dataset, where we characterized 22 subjects with varying degree of HS. Significance statement The oral and gut microbiota alterations have been linked to NAFLD. There is a lack of data on multi-omics characteristics of hepatic steatosis by exclusion of major confounding factors of obesity and metabolic syndrome. We observed that the oral and gut microbiota remodelling starts at early stages of the NAFLD spectrum, independent of obesity and metabolic syndrome. Our analysis suggested that the bacterial diversity is correlated with multi-omics signatures in NAFLD and our predictive model created based on multi-omics variables can successfully predict hepatic steatosis. The components of the multi-omics signatures may serve as biomarkers and can be pharmaceutically targeted. Future clinical trials with microbiota manipulation could consider intervention at early stages of NAFLD. medRxiv preprint doi: https://doi.org/10.1101/2021.05.22.21257482; this version posted May 23, 2021. The copyright holder for this preprint (which was not certified by peer review) is the author/funder, who has granted medRxiv a license to display the preprint in perpetuity. It is made available under a CC-BY-ND 4.0 International license . 3 Introduction Non-alcoholic fatty liver disease (NAFLD) is characterized by deposition of lipid droplets in the liver without significant alcohol consumption and secondary causes. NAFLD constitutes a wide range of the clinical spectrum, including hepatic steatosis (HS) and non-alcoholic steatohepatitis (NASH), which may ultimately lead to advanced fibrosis and cirrhosis. In parallel to ongoing epidemics of obesity, NAFLD incidence is increasing globally, affecting almost one-fourth of the population (Abeysekera et al, 2020; Bugianesi, 2020). Even though NAFLD is becoming a leading aetiology of chronic liver disease, therapeutic approaches for NAFLD are currently limited with lifestyle modifications encompassing dietary intervention and physical activity (Alferink et al, 2019; Romero-Gómez et al, 2017; Sanyal, 2019) Given that NAFLD is closely linked to metabolic syndrome, obesity and type 2 diabetes (T2D), its pathogenesis is remarkably complex. Discoveries of PNPLA3 and TM6SF2 variants have highlighted the pathological processes leading to metabolic disturbances in HS. However, only 10- 20% of NAFLD susceptibility could be attributed to known genetic variants (Eslam & George, 2020). Exploring the connections between the hepatic and extra-hepatic metabolic factors through the generation of the multi-omics data may reveal the underlying molecular mechanisms associated with the disease's occurrence, discovering novel biomarkers and drug targets, and eventually provide further insight for the development of efficient treatment strategies. The dysbiosis and diversity in the oral and gut microbiome have been associated with NAFLD (Boursier et al, 2016; Mardinoglu et al, 2018c) as well as metabolic syndrome (Vrieze et al, 2012; Yuan et al, 2019), type 2 diabetes (Mardinoglu et al, 2019) and obesity (Leung et al, 2016). Studies indicated that microbiome composition changes are associated with advanced hepatic fibrosis (Loomba et al, 2017) and cirrhosis (Bajaj et al, 2014; Lelouvier et al, 2016). Moreover, several multi-omics studies integrated complementary –omics data across various environmental states through systems biology. These studies demonstrated that a multi-omics approach is a powerful tool for understanding the dynamics of biological functions in liver diseases and other associated metabolic conditions (Altay et al, 2019; Mardinoglu et al, 2018a). Here, we performed an in-depth characterization of 56 subjects to decipher NAFLD pathogenesis by excluding critical confounding factors including obesity, diabetes and genetic variant- associated HS (Figure 1A). We collected saliva and faeces samples for studying the dysbiosis in the oral and gut microbiome through the generation of shotgun metagenomics data and identified the key species involved in various stages of HS. Moreover, we performed plasma metabolomics and inflammatory proteomics analysis to explore the host-microbiome interactions. We studied the altered global metabolic and inflammatory processes, and its connections with the species' abundances in the oral and gut microbiome. We integrated this multi-omics data using biological networks and identified key features of HS using multi-omics data. We finally predicted HS using these key features and validated our findings in an independent validation dataset, where we characterized 22 subjects with varying HS. medRxiv preprint doi: https://doi.org/10.1101/2021.05.22.21257482; this version posted May 23, 2021. The copyright holder for this preprint (which was not certified by peer review) is the author/funder, who has granted medRxiv a license to display the preprint in perpetuity. It is made available under a CC-BY-ND 4.0 International license . 4 Results The associations between HS and clinical and physical variables We generated data for 56 overweight and obese subjects (BMI>28.8) with a varying degree of HS. We excluded patients if they carry PNPLA3 I148M (homozygous for I148M). We determined HS by the proton density fat fraction, measured by magnetic resonance imaging (MRI-PDFF). We classified the subjects into four different groups: i) 10 subjects with no steatosis (HS<5.5%), ii) 14 subjects with mild steatosis (5.5%≤HS<8%), ii) 20 subjects with moderate steatosis (8.0%≤HS<16.5%) and iv) 12 subjects with severe steatosis (HS ≥16.5%) (Figure 1A-B). We carefully phenotyped these subjects by measuring clinical and physical variables (Dataset S1A- C). We also collected saliva and
Details
-
File Typepdf
-
Upload Time-
-
Content LanguagesEnglish
-
Upload UserAnonymous/Not logged-in
-
File Pages35 Page
-
File Size-