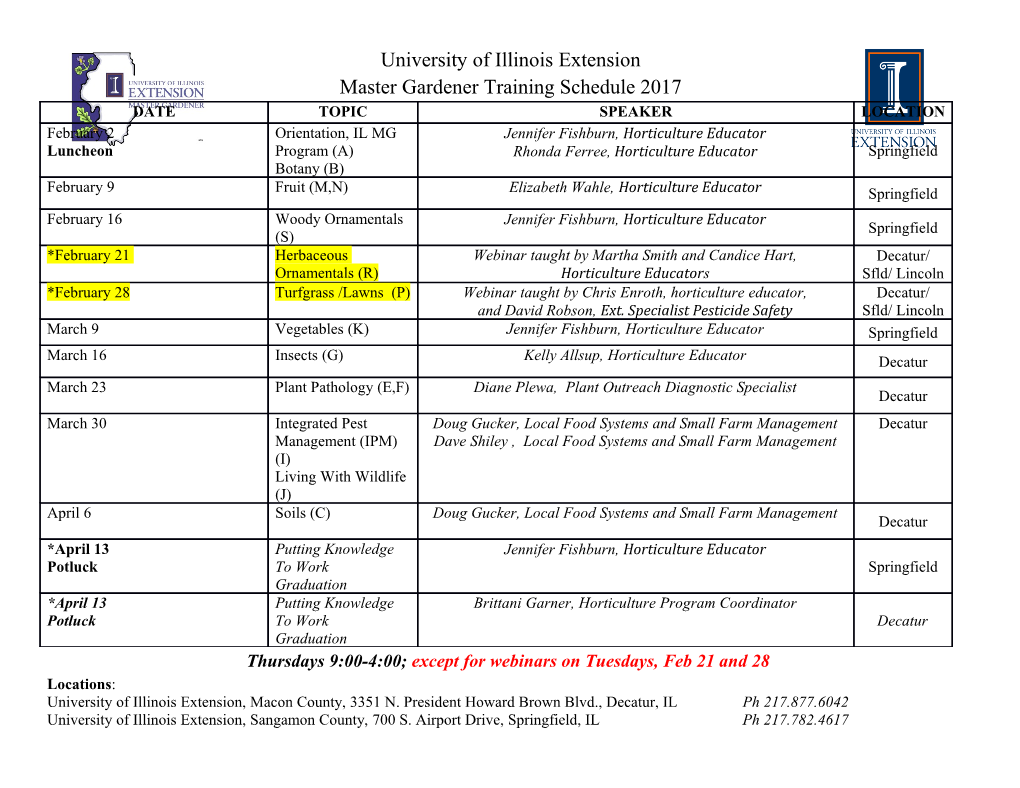
WIN : An Efficient Data Placement Strategy for Parallel XML Databases Nan Tang† Guoren Wang‡ Jeffrey Xu Yu† Kam-Fai Wong† Ge Yu‡ † Department of SE & EM, The Chinese University of Hong Kong, Hong Kong, China ‡ College of Information Science and Engineering, Northeastern University, Shenyang, China {ntang, yu, kfwong}se.cuhk.edu.hk {wanggr, yuge}@mail.neu.edu.cn Abstract graph-based. Distinct from them, XML employs a tree struc- The basic idea behind parallel database systems is to ture. Conventional data placement strategies in perform operations in parallel to reduce the response RDBMSs and OODBMSs cannot serve XML data well. time and improve the system throughput. Data place- Semistructured data involve nested structures; there ment is a key factor on the overall performance of are much intricacy and redundancy when represent- parallel systems. XML is semistructured data, tradi- ing XML as relations. Data placement strategies in tional data placement strategies cannot serve it well. RDBMSs cannot, therefore, well solve the data place- In this paper, we present the concept of intermediary ment problem of XML data. Although graph partition node INode, and propose a novel workload-aware data algorithms in OODBMSs can be applied to tree parti- placement WIN to effectively decluster XML data, to tion problems, the key relationships between tree nodes obtain high intra query parallelism. The extensive ex- such as ancestor-descendant or sibling cannot be clearly periments show that the speedup and scaleup perfor- denoted on graph. Hence data placement strategies mance of WIN outperforms the previous strategies. for OODBMSs cannot well adapt to XML data either. Furthermore, the path based XML query languages such as XPath and XQuery enhance the hardness and 1 Introduction intricacy of tree data placement problem. Data place- ment strategies in both parallel relational and object- XML has become the de facto standard for data oriented database systems are helpful to the data place- representation and exchange over the Internet. Nev- ment for XML documents. They cannot, nevertheless, ertheless, along with the increasing size of XML doc- solve the problem which XML faces. Efficient data ument and complexity of evaluating XML queries, ex- placement strategies for XML DBMSs are, therefore, isting query processing performance in concentrative meaningful and challenging. environment will get a limitation. Parallelism is a vi- [13] proposed two data placement strategies for able solution to this problem. XML data tree: NSNRR and PSPIB. NSNRR is pro- In parallel DBMSs, data placement has a signifi- posed to exploit pipeline query parallelism. With cant impact on the overall system performance. Sev- NSNRR elements having different tag names are al- eral earlier studies have shown that data placement is located to different sites in a round-robin way. Previ- closely relevant to the performance of a shared-nothing ous experiments show that when the document is small parallel architecture. Many classical data placement NSNRR has good speedup and scaleup performance; strategies are thereby proposed in the literature. [2,11] but as the document grows, both the speedup and proposed many single dimension and multiple dimen- scaleup performance of NSNRR behave badly. PSPIB sion data placement strategies for structured data in is a data placement strategy based on path instances. RDBMSs. In OODBMSs otherwise, since the objects Path instance is informally defined as a sequence of can be arbitrarily referenced by one another, the re- node identifiers from root to leaf node, while path lationship of objects is regarded as a graph on topol- schema is an abstract of path instance. The main ogy. Thus most of the object-placement strategies are idea of PSPIB is to decluster the path instances having 1 the same path schema evenly across all sites. Because And yet these nodes are the crux of many XML query PSPIB and WIN have the similar idea to partition the expressions. Therefore, we should duplicate these XML data tree to form sub-trees, we compare WIN nodes to all sites; the tiny storage space consumption with PSPIB in the performance evaluation. We give a can trade off between high intra query parallelism and simple example of data distribution of PSPIB to make low communication overhead. We will partition the re- it more concrete. Given a lightweight XML data tree maining sub-trees to different sites, and then in each in Fig. 1, Fig. 2 shows the data placement under the site, combine the sub-trees and duplicated nodes to case of two sites. Solid circles represent the elements form a local data tree. WIN describes how to find a duplicated at both sites; real circles represent the ele- group of duplicate nodes and a group of sub-trees to ments distributed to site 1; dotted circles represent the tradeoff the duplication cost and high parallelism. The elements distributed to site 2. problem is formally defined as follows: Root Let XT be an XML data tree, Q be the query set a b 1 1 of n XML query expressions Q1,Q2, · · · ,Qn, and fi be c1 c2 c4 c5 c8 c9 c11 c12 the frequency of Qi, let N > 1 be the number of sites. How to partition XT to N sub-trees XT1 ∼ XTN , d1 d2 d3 d5 d6 d7 d9 d11 d12 d13 d15 d16 d18 N XT = XT , to take each Q a high intra query c3 c6 c7 c10 c13 c14 i i i=1 d4 d8 d10 d14 d17 d19 parallelism.S Figure 1. A Lightweight XML Data Tree We will first present the concept of INode, and then give the basic data placement idea based on INode, last Root we give our workload estimation formulae. a1 b1 2.1 Intermediary Node c1 c2 c4 c5 c8 c9 c11 c12 d1 d2 d3 d5 d6 d7 d9 d11 d12 d13 d15 d16 d18 We classify nodes in an XML data tree XT into c3 c6 c7 c10 c13 c14 three classes: Duplicate Node (DNode), Intermediary Node (INode) and Trivial Node (T Node). DNode will d d d d d d 4 8 10 14 17 19 be duplicated at all sites. INode is the essential node Figure 2. Data Placement of PSPIB that decides which nodes will be partitioned to different In this paper, we present the concept of interme- sites. T Node will be partitioned to the site where their diary node INode. Considering that determining the ancestor INode is. We use DN to represent the set of best data placement strategy in parallel DBMSs is an DNodes, IN for INodes and TN for T Nodes. They NP-complete [9] problem, a novel workload-aware data satisfy the following properties: placement strategy WIN is proposed to find the sub- 1. DN 0s ancestor nodes are DN or NULL; optimal INode set for partitioning XML data tree. 2. IN 0s ancestor nodes are DN or NULL; This strategy is not aim at special queries, neverthe- 3. IN 0s descendant nodes are TN or attribute nodes less, as will be shown, this strategy suits each query or text nodes or NULL; expression for good intra query parallelism. Based on 4. DN is duplicated in all sites, IN, TN are unique; an XML database benchmark–XMark [10], comprehen- 5. IN ∩ DN ∩ TN = Ø; sive experimental results show that our new data place- 6. IN ∪ DN ∪ TN = XT . ment strategy WIN has better speedup and scaleup DN forms the duplicated root tree in all sites, and performance than previous work. each INode in IN is the root of a sub-tree to be par- The rest of this paper is organized as follows. Sec- titioned. IN is the pivot of our data placement strat- tion 2 gives the concept of INode and the basic idea for egy. For a certain IN, our partition algorithm will data placement. Section 3 provides a detail statement get one data placement result. Different IN will con- of the novel data placement strategy WIN . Section duct different data placement, the key of our novel data 4 shows experimental results. Our conclusions and fu- placement strategy is how to find the best IN. There ture work are contained in Section 5. are numerous IN in a data tree, as shown in Fig. 3, {b, c} is a possible INode set, and also {b, f, g} and 2 Data Placement using INode {d, e, c}, INode could be leaf node, e.g., {b, l, m, g}, {b, f, n, o}, {b, l, m, n, o}, etc. The extreme case of IN Observe from a common XML data tree, we find is all INodes are leaf nodes such as {h, i, j, k, l, m, n, o}. that the number of upper level nodes is always few. How to choose the best IN is obvious a NP-Complete 2 problem, as discussed in [9] which search the best data at proposing a workload estimation model to meet de- placement strategy in parallel DBMSs. mand for common XML query processing techniques a instead of certain algorithms or specific systems. Now we will give our workload cost model to eval- b c uate the workload of a data tree with root a. Table 1 gives the variables used in this paper. d e f g Variable Description na the number of element a h i j kl mno N the number of sites na b the result number of a b Figure 3. Example for INode Sa the size of element a Qi the i-th query in statistics 2.2 Data Placement based on INode fi query frequency of Qi δa b selectivity of operation a b t avg. disk access time The basic idea of data placement based on INode I/O v net speed is described below.
Details
-
File Typepdf
-
Upload Time-
-
Content LanguagesEnglish
-
Upload UserAnonymous/Not logged-in
-
File Pages7 Page
-
File Size-