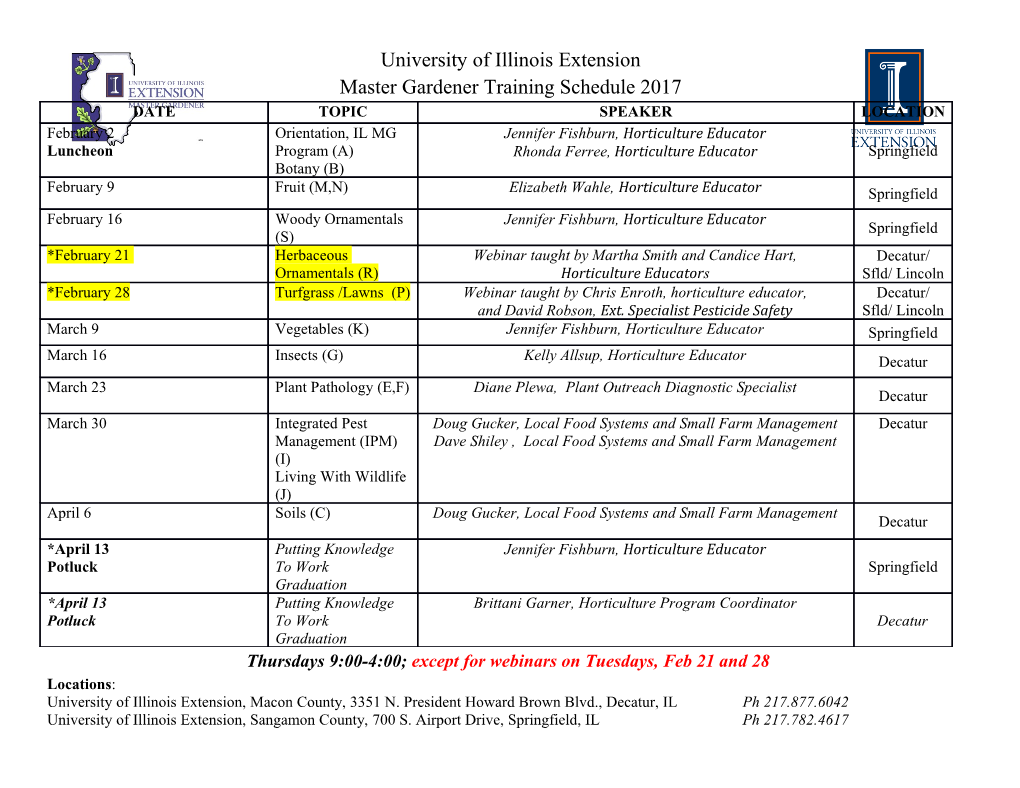
COMPLEXINTERNALREPRESENTATIONS INSENSORIMOTORDECISIONMAKING: A BAYESIAN INVESTIGATION luigi acerbi I V N E R U S E I T H Y T O H F G E R D I N B U Doctor of Philosophy Doctoral Training Centre for Computational Neuroscience Institute of Perception, Action and Behaviour School of Informatics University of Edinburgh 2014 Luigi Acerbi: Complex internal representations in sensorimotor decision making: A Bayesian investigation Doctor of Philosophy, 2014 supervisors: Prof. Sethu Vijayakumar, Ph.D., FRSE Prof. Daniel M. Wolpert, D.Phil., FRS, FMedSci ABSTRACT The past twenty years have seen a successful formalization of the idea that percep- tion is a form of probabilistic inference. Bayesian Decision Theory (BDT) provides a neat mathematical framework for describing how an ideal observer and actor should interpret incoming sensory stimuli and act in the face of uncertainty. The predictions of BDT, however, crucially depend on the observer’s internal models, represented in the Bayesian framework by priors, likelihoods, and the loss function. Arguably, only in the simplest scenarios (e.g., with a few Gaussian variables) we can expect a real observer’s internal representations to perfectly match the true statistics of the task at hand, and to conform to exact Bayesian computations, but how humans systemati- cally deviate from BDT in more complex cases is yet to be understood. In this thesis we theoretically and experimentally investigate how people represent and perform probabilistic inference with complex (beyond Gaussian) one-dimensional distributions of stimuli in the context of sensorimotor decision making. The goal is to reconstruct the observers’ internal representations and details of their decision- making process from the behavioural data – by employing Bayesian inference to un- cover properties of a system, the ideal observer, that is believed to perform Bayesian inference itself. This “inverse problem” is not unique: in principle, distinct Bayesian observer models can produce very similar behaviours. We circumvented this issue by means of experimental constraints and independent validation of the results. To understand how people represent complex distributions of stimuli in the spe- cific domain of time perception, we conducted a series of psychophysical experiments where participants were asked to reproduce the time interval between a mouse click and a flash, drawn from a session-dependent distribution of intervals. We found that participants could learn smooth approximations of the non-Gaussian experimental distributions, but seemed to have trouble with learning some complex statistical fea- tures such as bimodality. To investigate whether this difficulty arose from learning complex distributions or computing with them, we conducted a target estimation experiment in which “priors” where explicitly displayed on screen and therefore did not need to be learnt. Lack of difference in performance between the Gaussian and bimodal conditions in this task suggests that acquiring a bimodal prior, rather than computing with it, is the major difficulty. Model comparison on a large number of Bayesian observer models, representing different assumptions about the noise sources and details of the decision iii process, revealed a further source of variability in decision making that was modelled as a “stochastic posterior”. Finally, prompted by a secondary finding of the previous experiment, we tested the effect of decision uncertainty on the capacity of the participants to correct for added perturbations in the visual feedback in a centre of mass estimation task. Participants almost completely compensated for the injected error in low uncertainty trials, but only partially so in the high uncertainty ones, even when allowed sufficient time to adjust their response. Surprisingly, though, their overall performance was not signif- icantly affected. This finding is consistent with the behaviour of a Bayesian observer with an additional term in the loss function that represents “effort” – a component of optimal control usually thought to be negligible in sensorimotor estimation tasks. Together, these studies provide new insight into the capacity and limitations people have in learning and performing probabilistic inference with distributions beyond Gaussian. This work also introduces several tools and techniques that can help in the systematic exploration of suboptimal behaviour. Developing a language to describe suboptimality, mismatching representations and approximate inference, as opposed to optimality and exact inference, is a fundamental step to link behavioural studies to actual neural computations. iv L AY S U M M A RY A successful unifying hypothesis in neuroscience is that the nervous system attempts to choose a ‘statistically optimal’ course of action by accounting for sensory uncer- tainty and previous experience while serving the goals of the task at hand. Such optimal behaviour requires an ideal observer to record the pattern of past events and build an accurate internal model of the environment. Arguably, the performance of a real observer will match that of an ideal observer only in the simplest scenarios (e.g., those following simple statistical regularities) as we would not expect the brain to be able to recall and assess arbitrarily complex patterns of past events. However, we have yet to ascertain which statistical features of the environment induce suboptimal behaviour, and why. In this thesis, we investigate people’s performance in perceptual and motor tasks in the presence of events whose patterns feature varying levels of regularity and com- plexity. The goal is to understand what people learn about these patterns, and how the learnt patterns are employed in people’s decision making process. We start by asking how people represent complex patterns of stimuli in the specific case of time perception. We conducted a series of psychophysical experiments where participants were asked to reproduce the time interval between a mouse click and a flash, drawn from a session-dependent pattern of intervals. We found that participants could learn, through practice, generic statistical features of the presented patterns, but had trou- ble with some specific aspects of those. In fact, in a separate psychophysical study, we found evidence that remembering the patterns of past events constitutes more of a challenge to decision making than manipulating the present complex statistical information. Finally, we asked whether a major source of suboptimality can be iden- tified in the mental or physical effort required by the nervous system to correct for mistakes. We found that people are ‘optimally lazy’; that is, they correct just enough to preserve their average performance in the task. Together, these studies provide new insight into the human capacity for, and limita- tions in, learning and making statistically optimal decisions when dealing with prior events with complex patterns. This work also introduces several tools and techniques that can help in the systematic exploration of suboptimal behaviour. Developing a lan- guage to describe suboptimality, mismatching representations, and approximations – as opposed to optimality – is a fundamental step to link behavioural studies to actual neural computations in the brain. v PREFACE acknowledgements Here we are. I would like to thank my first supervisor, Sethu Vijayakumar, for giving me the freedom to pursue my research interests, for providing timely guidance and insight, and above all for reminding me to always be clear about the bigger picture, and resist the charm of mathematical minutiae. I am grateful to my second supervisor, Daniel Wolpert, for the many helpful Skype calls (recently, Google hangouts), for backing my several working visits to his lab during the course of my PhD, and for imbuing me with an understanding of the intricate psychophysical process whereby a tentative draft of a manuscript is transmuted into a scientific paper. I also thank Sae Franklin and James Ingram for their invaluable practical and technical support during my lab visits in Cambridge, and Peggy Seriès for introducing me to the wonders of the Bayesian brain. The work presented in Chapter 3 received constructive feedback from Michael S. Landy and two anonymous reviewers. At various stages of its development, the study reported in Chapter 4 has benefited from helpful suggestions from Jeff Beck, Sophie Denève, Jan Drugowitsch, Wei Ji Ma, Paul R. Schrater, Angela J. Yu, and one or two anonymous reviewers. I would like to thank several other people and fellow travellers who have directly or indirectly contributed to this thesis, by virtue of stimulating discussions or reciprocal encouragement: Paolo Puggioni, Lukas Solanka, and the rest of the DTC09 crew; then Matteo Colombo, Megan Peters, David Reichert, and Michael Schartner. I also thank the friends of a lifetime, old and new, who kept my stay in Scotland between bearable and amazing. I am grateful to my family beyond words. And, finally, I thank Maryam Babaei for carefully reading this thesis, fixing com- mas, typos, and Italianisms, for her thoughtful comments, and above all for her un- wavering support for almost the full extent of my PhD. Thank you. vii publications and contributions The work reported in Chapters 3, 4 and 5 is the result of my collaboration with Prof. Sethu Vijayakumar (University of Edinburgh, Edinburgh, UK) and Prof. Daniel Wolpert (University of Cambridge, Cambridge, UK). Chapter 3 is largely based on a paper published in PLoS Computational
Details
-
File Typepdf
-
Upload Time-
-
Content LanguagesEnglish
-
Upload UserAnonymous/Not logged-in
-
File Pages267 Page
-
File Size-