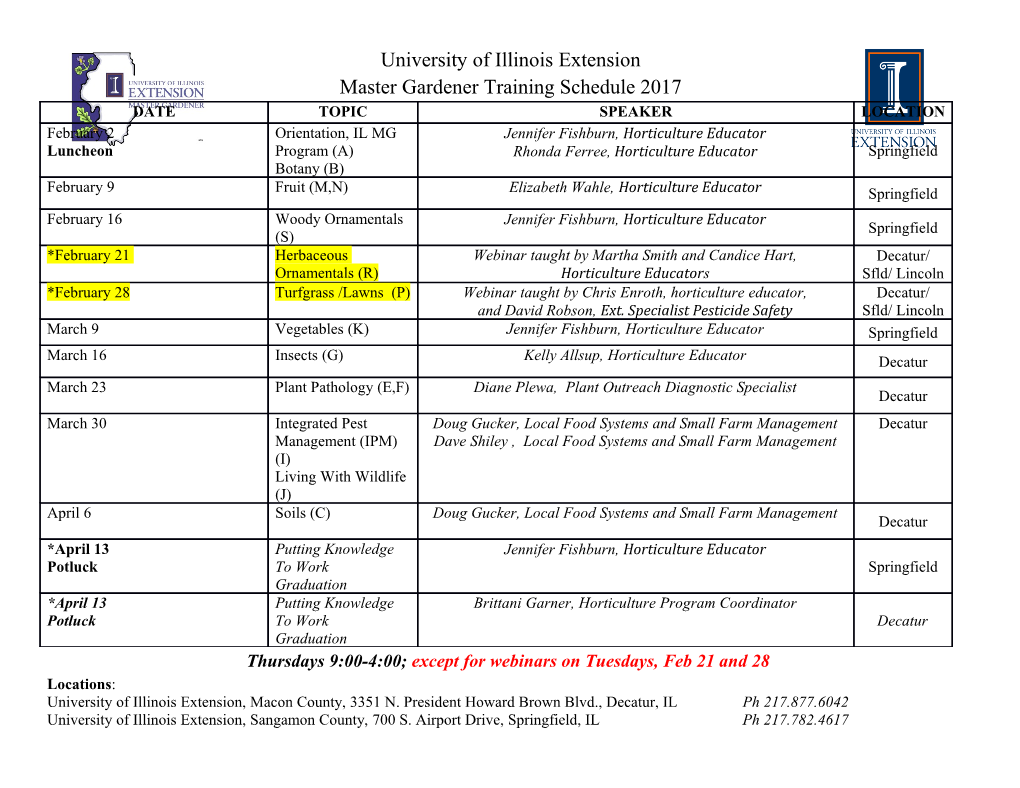
Kinetic profiling of metabolic specialists demonstrates stability and consistency of in vivo enzyme turnover numbers David Heckmanna,1, Anaamika Campeaub, Colton J. Lloyda, Patrick V. Phaneufa, Ying Hefnera, Marvic Carrillo-Terrazasb, Adam M. Feista,c, David J. Gonzalezb, and Bernhard O. Palssona,c,1 aDepartment of Bioengineering, University of California San Diego, La Jolla, CA 92093; bDepartment of Pharmacology, Skaggs School of Pharmacy and Pharmaceutical Sciences, University of California San Diego, La Jolla, CA 92093; and cThe Novo Nordisk Foundation Center for Biosustainability, Technical University of Denmark, 2800 Lyngby, Denmark Edited by Athel Cornish-Bowden, Institut de Microbiologie de la Méditerranée, CNRS, Marseille, France, and accepted by Editorial Board Member Alan R. Fersht July 28, 2020 (received for review February 11, 2020) Enzyme turnover numbers (kcats) are essential for a quantitative proteomic quantification techniques are still suffering from high k understanding of cells. Because cats are traditionally measured in variation, and previous estimates of kcat were based on in silico 13 low-throughput assays, they can be inconsistent, labor-intensive to flux predictions rather than C fluxomics data. Furthermore, kcat obtain, and can miss in vivo effects. We use a data-driven approach is expected to scale with growth rate (4). As many experimental k Escherichia coli to estimate in vivo cats using metabolic specialist conditions in the literature data used in Davidi et al. (3) resulted in strains that resulted from gene knockouts in central metabolism low growth rates, the effective number of datasets contributing to followed by metabolic optimization via laboratory evolution. By kapp,max is low. Finally, if kapp,max is a useful estimator of in vivo combining absolute proteomics with fluxomics data, we find that k k cat, it should improve the predictive capability of metabolic in vivo cats are robust against genetic perturbations, suggesting models on data that was not used to obtain k ,thatis,the that metabolic adaptation to gene loss is mostly achieved through app,max performance on a test set. other mechanisms, like gene-regulatory changes. Combining ma- Here, we present an approach for estimating k in vivo chine learning and genome-scale metabolic models, we show that cat (Fig. 1). We combined proteomic profiling with fluxomics data to the obtained in vivo kcats predict unseen proteomics data with much higher precision than in vitro k s. The results demonstrate that estimate in vivo kcatsinE. coli strains that have undergone strong cat physiological perturbations via knockout (KO) of metabolic in vivo kcats can solve the problem of inconsistent and low- coverage parameterizations of genome-scale cellular models. genes. To obtain strains with high growth rates for which kapp approaches kapp,max, adaptive laboratory evolution (ALE) (5) in vivo | turnover number | proteomics | kcat | gene knockout was used on the metabolic KO strains. We profiled 21 strains, representing metabolic specialists with diverse flux profiles that – nzyme catalytic rates are crucial for understanding many are able to obtain high growth rates (6 9). With this data-driven Eproperties of living systems like growth, proteome allocation, approach, we show that in vivo kcats are stable and robust to stress, and dynamic responses to perturbation. The turnover genetic perturbations, and that they can be used in genome-scale number of an enzyme, kcat, describes the maximal rate at which an enzyme’s catalytic site can catalyze a reaction. Knowledge of kcat Significance has traditionally been a bottleneck in the quantitative under- standing of cells, mainly because kcats have historically been Enzyme kinetic parameters are crucial for a quantitative under- obtained in labor-intensive, low-throughput in vitro assays. The standing of metabolism, but traditionally have to be measured substantial effort required for in vitro assays is likely the reason in laborious low-throughput assays. To solve this problem, the why, even in model organisms, only a small fraction of cellular enzyme turnover number, kcat, can be estimated in vivo, but it is enzymes has a measured kcat (1). Furthermore, in vitro kcat esti- unclear whether in vivo estimates represent stable systems pa- mates for the same enzyme that are found in databases are fre- rameters that can be used for metabolic modeling. We present quently very inconsistent when different literature sources are the data-driven estimation of in vivo kcats using proteomics and compared (2), probably because different experimental protocols flux data of metabolic knock out strains of Escherichia coli.Our are used. Furthermore, in vitro conditions can miss important results show that in vivo kcats are stable parameters that can be in vivo effects like posttranslational modifications, cellular used for metabolic modeling. We use the estimated in vivo kcats crowding, or metabolite concentrations. The latter points hamper to parameterize metabolic models and show that model per- the utilization of in vitro kcats in genome-scale metabolic models. formance for gene expression predictions increases drastically In order to address the problems of low-throughput acquisition compared to in vitro parameters. and in vivo−in vitro discrepancies, Davidi et al. (3) combined proteomics data and flux predictions to estimate in vivo turnover Author contributions: D.H., A.C., D.J.G., and B.O.P. designed research; D.H., A.C., C.J.L., P.V.P., Y.H., M.C.-T., and A.M.F. performed research; D.H., C.J.L., P.V.P., and A.M.F. ana- numbers based on apparent catalytic rate (kapp). Davidi et al. (3) lyzed data; and D.H. and B.O.P. wrote the paper. integrated published Escherichia coli proteomics datasets with The authors declare no competing interest. in silico flux predictions in multiple growth conditions and showed This article is a PNAS Direct Submission. A.C-B. is a guest editor invited by the that the resulting maximum apparent catalytic rate (kapp,max) Editorial Board. across growth conditions is significantly correlated with in vitro This open access article is distributed under Creative Commons Attribution-NonCommercial- kcats. Thus, kapp,max has the potential to overcome the problem of NoDerivatives License 4.0 (CC BY-NC-ND). − inconsistent conditions, low coverage, and in vitro in vivo dis- 1To whom correspondence may be addressed. Email: [email protected] or crepancies that hampers the use of in vitro kcats in large models of [email protected]. metabolism. However, it is unclear whether kapp,max is a stable This article contains supporting information online at https://www.pnas.org/lookup/suppl/ system parameter that is robust to perturbation, and how much doi:10.1073/pnas.2001562117/-/DCSupplemental. experimental procedures bias the estimation of kapp,max:Absolute First published September 1, 2020. 23182–23190 | PNAS | September 15, 2020 | vol. 117 | no. 37 www.pnas.org/cgi/doi/10.1073/pnas.2001562117 Downloaded by guest on September 29, 2021 Fig. 1. Approach for obtaining kcat in vivo from metabolic specialists: KO of enzymes in central metabolism was followed by ALE to obtain 21 strains that had diverse flux profiles, while achieving high growth rates (6–9). Fluxomics and proteomics data were then integrated for the evolved strains to obtain the maximum kapp across the 21 strains (kapp,max) for each enzyme that could be mapped uniquely. The obtained kapp,max vector was then extrapolated to genome scale via supervised machine learning and used to parameterize genome-scale metabolic models. The resulting genome-scale models were then validated on unseen proteomics data. SYSTEMS BIOLOGY models to obtain a high predictive performance for unseen Gene KO and ALE Cause Diversity in Enzyme Usage. We integrated protein abundance data. the measured protein abundances with 13C metabolic flux anal- ysis (MFA) fluxomics data (6–9) to calculate apparent catalytic Results rates in the 21 strains as the ratio of flux and protein abundance. Quantifying In Vivo Kinetics in Metabolic Specialists. In theory, Like in Davidi et al. (3), we only calculated kapp for homomeric kapp,max will approach kcat in vivo if a condition is found in which enzymes and reactions that are not catalyzed by multiple isoen- the respective enzyme is utilized at full efficiency. In order to zymes, to allow a specific mapping of proteins to reactions. This achieve strong genetic perturbations of enzyme usage, we used approach resulted in a median number of 258 enzymes per strain gene KO strains for the phosphotransferase system (PTS) for which we were able to calculate kapp. The resulting apparent (ptsHIcrr; ref. 6), the phosphoglucose isomerase (pgi; ref. 8), catalytic rates largely cluster by the genotype of the KO strain triosephosphate isomerase (tpiA; ref. 7), and succinate dehy- (Fig. 2A), confirming that enzyme usage was indeed perturbed by the respective KOs. Across the 21 strains, the maximum ob- drogenase (sdhCB; ref. 9). As kapp increases with growth rate (4), we used KO strains that were optimized for growth on glucose served kapp of an enzyme is, on average, 4.4 times larger than the minimal medium via ALE (6–9) experiments. In addition to smallest observed kapp (Fig. 2B). This result indicates that con- these KO strains, we utilized a wild-type (WT) MG1655 strain siderable variation in enzyme usage was caused by the metabolic that was subjected to ALE (6–10). As evolution is not a deter- gene KO. To exclude the possibility that experimental variation ministic process, ALE endpoints differ in genotype, and we in- causes this apparent diversity in enzyme usage, we compared the SD of kapp in biological replicates (mean on log10 scale = 0.07) to cluded a total of 21 strains that resulted from replicates of ALE = experiments (i.e., four endpoint strains for ptsHIcrr, eight for pgi, the SD measured across the 21 strains (mean on log10 scale 0.18). We found that the variation caused by KOs and ALE is four for tpiA, three for sdhCB, and two WT controls) and that significantly larger than that caused by experimental variation were representative for the respective endpoint population.
Details
-
File Typepdf
-
Upload Time-
-
Content LanguagesEnglish
-
Upload UserAnonymous/Not logged-in
-
File Pages9 Page
-
File Size-