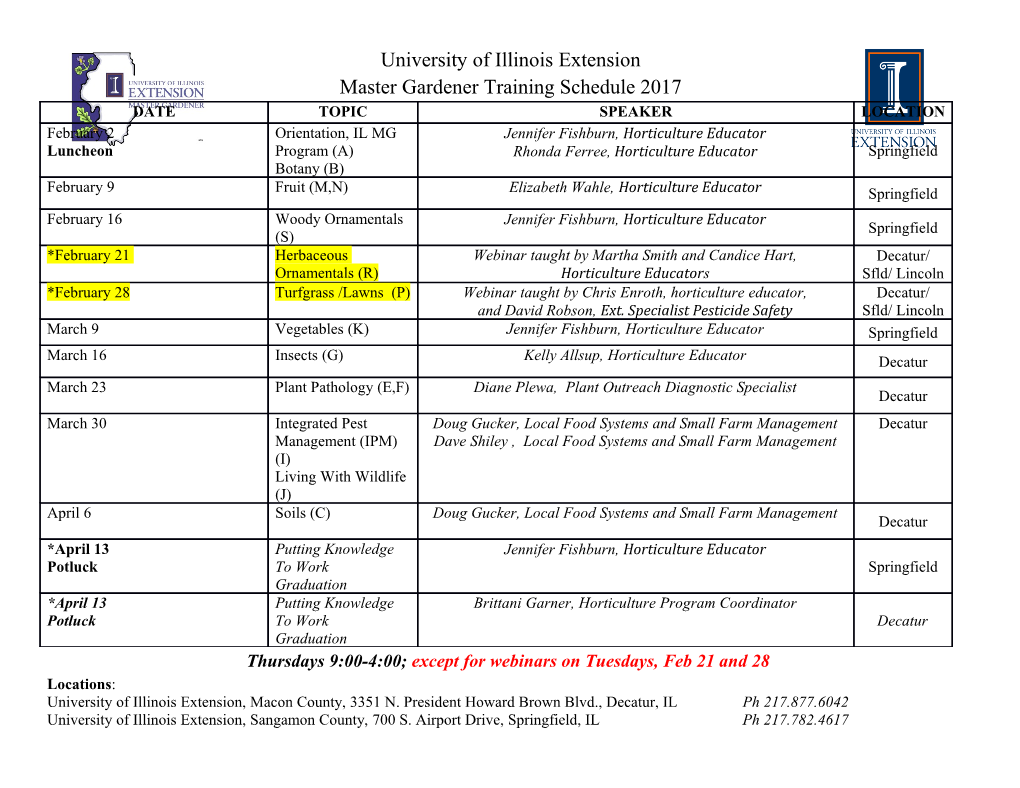
Transfer entropy in continuous time, with applications to jump and neural spiking processes Dr. Joseph T. Lizier ARC DECRA Fellow / Senior Lecturer Centre for Complex Systems The University of Sydney December 2016 Outline TE Cont’-time Spike trains Examples Estimator Close 2 Manuscripts � Richard E. Spinney, Mikhail Prokopenko and Joseph T. Lizier, “Transfer entropy in continuous time, with applications to jump and neural spiking processes”, in review, 2016. arXiv:1610.08192 � Terry Bossomaier, Lionel Barnett, Michael Harr´eand Joseph T. Lizier, “An Introduction to Transfer Entropy: Information Flow in Complex Systems”, Springer, 2016. � Joseph T. Lizier, Richard E. Spinney, Mikail Rubinov, Michael Wibral and Viola Priesemann, “A nearest-neighbours based estimator for transfer entropy between spike trains”, in preparation, 2016 J.T. Lizier TE in spike trains December 2016 Outline TE Cont’-time Spike trains Examples Estimator Close 3 Motivation Measuring directed information transmission in neural recordings allows us to: � characterise neural computation in terms of information storage, transfer and modification (Wibral et al., 2015); � detect informationflows in space and time (Wibral et al., 2014a); � investigate why critical dynamics are computationally important (Barnett et al., 2013; Boedecker et al., 2012; Priesemann et al., 2015); � infer effective information networks (Lizier et al., 2011; Vicente et al., 2011; Wibral et al., 2011) – see e.g. TRENTOOL (Lindner et al., 2011). J.T. Lizier TE in spike trains December 2016 Outline TE Cont’-time Spike trains Examples Estimator Close 4 Motivation � Transfer entropy is the tool of choice for measuring directed information transmission in neural recordings. � It has been used across all modalities, and for multiple purposes J.T. Lizier TE in spike trains December 2016 Outline TE Cont’-time Spike trains Examples Estimator Close 4 Motivation � Transfer entropy is the tool of choice for measuring directed information transmission in neural recordings. � It has been used across all modalities, and for multiple purposes � Yet precise method of application to spike trains remains unclear: � With time-binning, how to set bin sizes / history length appropriately, capturing all relationships and avoiding undersampling? � Can we remain in continuous time, and is this more accurate? J.T. Lizier TE in spike trains December 2016 Outline TE Cont’-time Spike trains Examples Estimator Close 5 Outline 1 Transfer entropy 2 Continuous-time TE 3 Transfer entropy in spike trains 4 TE examples for spike trains 5 TE estimator for spike trains 6 Summary J.T. Lizier TE in spike trains December 2016 Outline TE Cont’-time Spike trains Examples Estimator Close 6 Information dynamics Key question: how is the next state of a variable in a complex system computed? Q: Where does the information inx n+1 come from, and how can we measure it? Q: How much was stored, how much was transferred, can we partition them or do they overlap? Complex system as a multivariate time-series of states J.T. Lizier TE in spike trains December 2016 Outline TE Cont’-time Spike trains Examples Estimator Close 7 Information dynamics Studies computation of the next state of a target variable in terms of information storage, transfer and modification: (Lizier et al., 2008, 2010, 2012) The measures examine: � State updates of a target variable; � Dynamics of the measures in space and time. J.T. Lizier TE in spike trains December 2016 Outline TE Cont’-time Spike trains Examples Estimator Close 8 Active information storage (Lizier et al., 2012) How much information about the next observationX n+1 of process (k) X can be found in its past state Xn = X n k+1 ...X n 1,X n ? { − − } Active information storage: (k) (k) AX =I(X n+1;X n ) p(x x(k)) = log n+1| n 2 p(xn+1) Average� information from� past state that is in use in predicting the next value. http://jlizier.github.io/jidt J.T. Lizier TE in spike trains December 2016 Outline TE Cont’-time Spike trains Examples Estimator Close 9 Information transfer (k) How much information about the state transition Xn X n+1 of (l) → X can be found in the past state Yn of a source processY? Transfer entropy: (Schreiber, 2000) (k,l) (l) (k) TY X =I(Y n ;X n+1 X n ) → p(x x(k)|,y(l))) = log n+1| n n 2 p(x x(k))) n+1| n Average� info from source that� helps predict next value in context of past. http://jlizier.github.io/jidt J.T. Lizier TE in spike trains December 2016 Outline TE Cont’-time Spike trains Examples Estimator Close 9 Information transfer (k) How much information about the state transition Xn X n+1 of (l) → X can be found in the past state Yn of a source processY? Transfer entropy: (Schreiber, 2000) (k,l) (l) (k) TY X =I(Y n ;X n+1 X n ) → p(x x(k)|,y(l))) = log n+1| n n 2 p(x x(k))) n+1| n Average� info from source that� helps predict next value in context of past. Storage and transfer are complementary: http://jlizier.github.io/jidt HX =A X +T Y X + higher order terms → J.T. Lizier TE in spike trains December 2016 Outline TE Cont’-time Spike trains Examples Estimator Close 9 Information transfer (k) How much information about the state transition Xn X n+1 of (l) → X can be found in the past state Yn of a source processY? Transfer entropy: (Schreiber, 2000) (k,l) (l) (k) TY X =I(Y n ;X n+1 X n ) → p(x x(k)|,y(l))) = log n+1| n n 2 p(x x(k))) n+1| n Average� info from source that� helps predict next value in context of past. Local transfer entropy: (Lizier et al., 2008) (k,l) (l) (k) tY X =i(y n ;x n+1 x n ) → p(x x(k|),y(l)) = log n+1| n n 2 p(x x(k)) n+1| n Storage and transfer are complementary: http://jlizier.github.io/jidt HX =A X +T Y X + higher order terms → J.T. Lizier TE in spike trains December 2016 Outline TE Cont’-time Spike trains Examples Estimator Close 10 Information dynamics in CAs cells 1 + 1 γ+ γ- γ α 5 5 α γ+ 0.5 0.8 γ- 10 10 0 -0.5 15 0.6 15 β γ- Domains and blinkers time γ- -1 20 20 α 0.4 α -1.5 are the dominant 25 25 - + γ - γ -2 0.2 γ 30 30 -2.5 information storage - α + γ γ -3 35 0 35 5 10 15 20 25 30 35 5 10 15 20 25 30 35 entities. (a) Raw CA (b) LAIS + + + + γ γ- γ 3 γ γ- γ 3 5 + 5 + - γ 2.5 γ- γ 2.5 Gliders are the γ 10 10 2 2 15 - 1.5 15 - 1.5 dominant information - γ - γ γ γ 1 20 1 20 transfer entities. - 0.5 - 0.5 25 γ - + 25 γ - + γ γ 0 γ γ 0 30 30 - γ+ -0.5 - γ+ -0.5 (Lizier et al., 2014). 35 γ 35 γ 5 10 15 20 25 30 35 5 10 15 20 25 30 35 (c) LTE right (d) LTE left J.T. Lizier TE in spike trains December 2016 Outline TE Cont’-time Spike trains Examples Estimator Close 11 TE in computational neuroscience Measuring directed information transmission using transfer entropy in neural recordings allows us to (Wibral et al., 2014a): � characterise neural computation in terms of information storage, transfer and modification (Wibral et al., 2015); � detect informationflows in space and time (Wibral et al., 2014a); � investigate why critical dynamics are computationally important (Barnett et al., 2013; Boedecker et al., 2012; Priesemann et al., 2015); � infer effective information networks (Lizier et al., 2011; Vicente et al., 2011; Wibral et al., 2011). J.T. Lizier TE in spike trains December 2016 Outline TE Cont’-time Spike trains Examples Estimator Close 12 TE applied to spike trains TE has been applied to spike trains, by time-binning to create a binary time series. J.T. Lizier TE in spike trains December 2016 Outline TE Cont’-time Spike trains Examples Estimator Close 12 TE applied to spike trains TE has been applied to spike trains, by time-binning to create a binary time series. For example by: � Ito et al. (2011), over multiple delays for effective network inference. See also Timme et al. (2014, 2016). � Priesemann et al. (2015), to investigate relationship to criticality. J.T. Lizier TE in spike trains December 2016 Outline TE Cont’-time Spike trains Examples Estimator Close 13 TE applied to spike trains But: how do we choose bin size and history, to capture full subtleties of relationship, but avoid undersampling? J.T. Lizier TE in spike trains December 2016 Outline TE Cont’-time Spike trains Examples Estimator Close 13 TE applied to spike trains But: how do we choose bin size and history, to capture full subtleties of relationship, but avoid undersampling? 1 Bin for max entropy – but this puts multiple spikes in one bin, misses timing subtleties. J.T. Lizier TE in spike trains December 2016 Outline TE Cont’-time Spike trains Examples Estimator Close 13 TE applied to spike trains But: how do we choose bin size and history, to capture full subtleties of relationship, but avoid undersampling? 1 Bin for max entropy – but this puts multiple spikes in one bin, misses timing subtleties. 2 Aim for one spike / bin – Ito et al. (2011) found performance increase with small bin sizes. But scale of bins becomes much smaller than relevant history period k .
Details
-
File Typepdf
-
Upload Time-
-
Content LanguagesEnglish
-
Upload UserAnonymous/Not logged-in
-
File Pages77 Page
-
File Size-