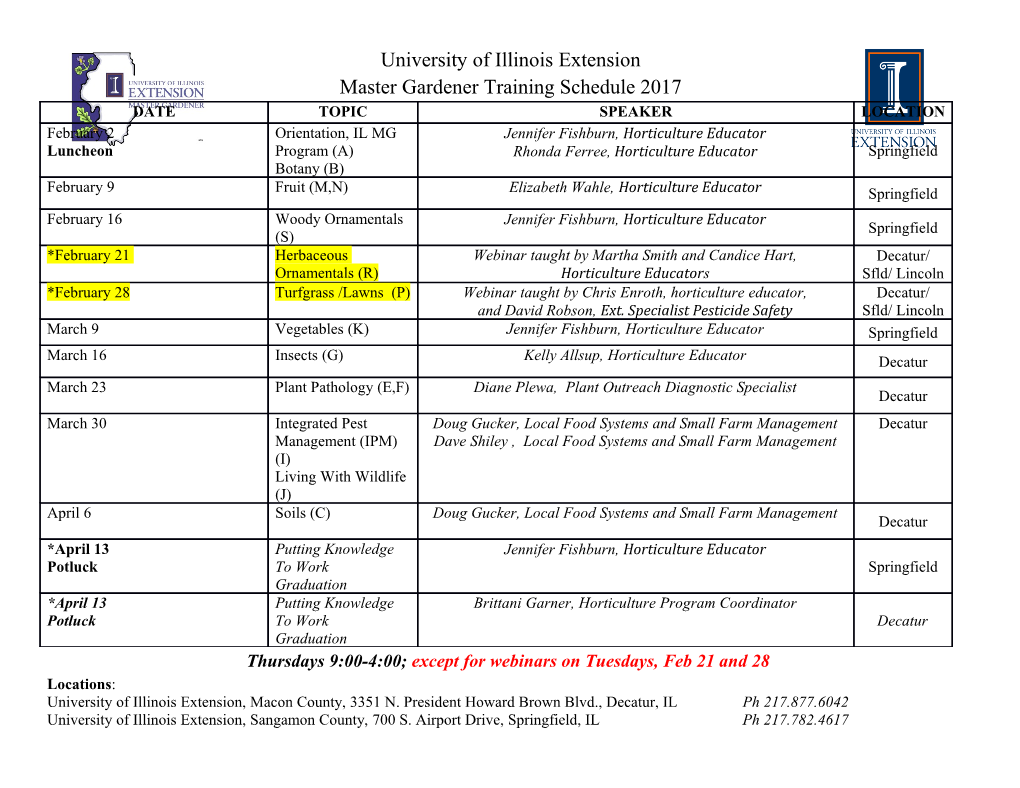
An Experimental Approach to Causal Identification of Spillover Effects under General Interference Alexander Coppock∗ and Neelanjan Sircar† Columbia University July 18, 2013 Abstract This paper extends the Rubin Causal Model to a general interference framework. In par- ticular, we develop a framework to define estimands in a general way using "reference assign- ments." This framework allows for the flexible stipulation of the non-interference assumptions required for estimation. We apply this approach to two common estimands, the average di- rect treatment effect and the average indirect exposure effect, recasting the framework in the language of network analysis. We use the network context to demonstrate the relationship between the potential outcomes and edges in a network, as well as to define intuitive non- interference assumptions. Finally, we derive unbiased and consistent estimators of average causal effects under interference and demonstrate their properties with simulated data. ∗[email protected] †[email protected] We would like to thank Peter Aronow, Albert Fang, Donald Green, Dominik Hangartner, Macartan Humphreys, Ryan Moore and participants at European Political Science Association Annual Meeting 2013, the 6th Annual Meeting of the Political Networks Section of the American Political Science Association, and the Columbia University Methods Workshop for useful comments and advice. All errors are our own. 1 1 Introduction Estimation of causal effects has typically relied on a strong assumption of non-interference be- tween experimental units. Many treatments, such as information, monitoring, or transfers, vi- olate this assumption in ways that are of great social scientific relevance, i.e., average spillover effects themselves are estimands of interest. We address the special estimation challenges asso- ciated with interference by extending the Rubin Causal Model to a general interference frame- work. In this paper, we derive a principled approach to the analysis of experiments under interference. We define estimands of interest with respect to "reference assignments," which are treatment assignment vectors that guarantee that we will observe the outcome of interest. The benefit of this approach is that estimands can be decoupled from assumptions about non-interference. Once an experiment has been conducted, we can flexibly state assumptions of non-interference, which allow for unbiased and consistent estimation of relevant estimands. We apply this approach to two common estimands, the average direct treatment effect and the average indirect exposure effect. In doing so, we recast a typical experiment involving spillovers in the language of network analysis. This allows us to comment on the relationship between the edges of a network and the potential outcomes for indirect exposure to a treatment. Furthermore, the network exposition allows us to frame plausible non-interference conditions in an intuitive way. Section 2 discusses relevant literature and a motivation for our approach. Section 3 derives our general framework using set-theoretic and probability measure-theoretic principles. Section 4 explores techniques to define non-interference conditions, as well as the bias and efficiency of estimation strategies, by applying the framework to a network context. Section 5 concludes the paper. 2 Related Literature and Motivation The assumption of "no interference between units" (Cox, 1958) is commonly made in experi- ments and is one component of the popularly assumed stable unit treatment value assumption, or SUTVA (Rubin, 1980). Non-interference, however, may not always hold between experimental units, as with treatments that are likely to spillover such as persuasion, monitoring, or educa- tion. Spillovers, or indirect exposure effects, bias naïve treatment effect estimates and may be of substantive relevance to researchers in their own right. In the past decade, researchers have begun seriously investigating indirect exposure effects through experimental methods. Estimands of interest from such studies include, for instance, the effects of vaccination on infection rates in surrounding areas, the effects of get-out-the-vote campaigns on neighbors’ voting rates, and the effects of educational interventions for students on their classmates. Such studies have often been conducted through multilevel experimentation or double randomization (e.g., Duflo and Saez (2003), Giné and Mansuri (2011), Sinclair, McConnell and Green (2012)). 2 In multilevel experiments, clusters are selected randomly from the universe of clusters of units in the population, e.g. neighborhoods or counties. For a random subset of selected clusters, a fixed percentage of units are assigned to treatment, and in the other clusters no units are assigned to treatment. Crucially, non-interference is assumed to hold across clusters but not within clusters. This yields three types of experimental groups, consisting of: (a) those units that are directly treated; (b) those units that are not directly treated but are located in clusters where units were treated (thus experiencing spillovers); and (c) those units that are not directly treated and are in clusters where no other unit was treated (serving as a control group). The average indirect effect is estimated as the average outcome in group (b) minus the average outcome in group (c). The statistical foundations of estimation via multilevel experimentation are discussed in detail in Hudgens and Halloran (2008). However, multilevel experiments may not always be feasible. The interference structure may not resemble clusters, as is often the case in the study of peer effects and spatial spillovers. In such cases, it is useful to stipulate a network of nodes and edges that describes the paths along which spillovers can occur. Experiments conducted in this more general network setting include Angelucci, de Giorgi, Rangel and Rasul (2010), Chen, Humphreys and Modi (2010), and Ichino and Schuendeln (2012). The framework we develop in this paper is a generalization of the classic Rubin Causal Model (Rubin (1974)) and its extension to multilevel experimentation (Hudgens and Halloran, 2008). In particular, we follow the insights of Rubin (1990) and Sobel (2006) and explicitly define potential outcomes as a function of the vector of treatment assignments resulting from the randomization. We use this idea to define "reference assignments," which are assignment vectors that guaran- tee that we an observe the potential outcome of interest. For example, we are guaranteed to see the control potential outcome for each unit under the assignment vector where no unit re- ceives treatment. Following Sobel (2006), we then define the estimand of interest by dividing differences in the appropriate potential outcomes by the number of reference assignments. The benefit of this approach is that it defines estimands of interest independently of non-interference assumptions. The stable unit treatment value assumption entails one of a set of many possible non-interference assumptions that may be appropriate for a given network structure. A common non-interference assumption is that units’ potential outcomes do not vary with the treatment assignments of those units that they are not connected to over some network. A more complex version of this assumption is often implicit: units’ potential outcomes may vary with the treatment assignments of direct neighbors but they do not vary with the assignments of indirect neighbors. For instance, we might allow a voter’s turnout decision to be affected when a friend is treated with a get-out- the-vote message, but assume that the voter’s decision is not affected when a friend of a friend is treated. Defining a non-interference assumption entails classifying the set of assignment vectors that would yield the same potential outcomes as a reference assignment. We may then use the known randomization distribution from the experiment and a stipulated non-interference assumption as to construct the probability of assignment to particular treatment conditions. We use inverse probability weighting to construct unbiased or consistent estimates of our estimands of interest. The inverse probability weighting approach, with application, is discussed in Chen, Humphreys 3 and Modi (2010). We refer readers to Aronow and Samii (2013b), who discuss the statistical and mathematical underpinnings of the inverse probability approach, including variance estimation, using “exposure mappings." 3 General Framework In this section, we develop a principled approach to constructing estimands of interest under interference. In particular, following Rubin (1990) and Sobel (2006), we define general poten- tial outcomes as a function of each possible vector of assignments to treatment statuses. Our estimands of interest are defined with respect to reference assignments over which we are guar- anteed to observe the relevant potential outcomes. After a set of estimands has been defined, we can flexibly stipulate non-interference assumptions and corresponding estimators. Many of the studies discussed above are interested in two estimands in particular: the direct and indirect effects of treatment. The average effect of direct exposure to a treatment is the average of all unit treatment effects in the absence of any interference. The average effect of (first-order) indirect exposure is the average of all spillover treatment effects, which are defined with respect to individual edges with receiver i and sender j. These quantities can be estimated
Details
-
File Typepdf
-
Upload Time-
-
Content LanguagesEnglish
-
Upload UserAnonymous/Not logged-in
-
File Pages18 Page
-
File Size-