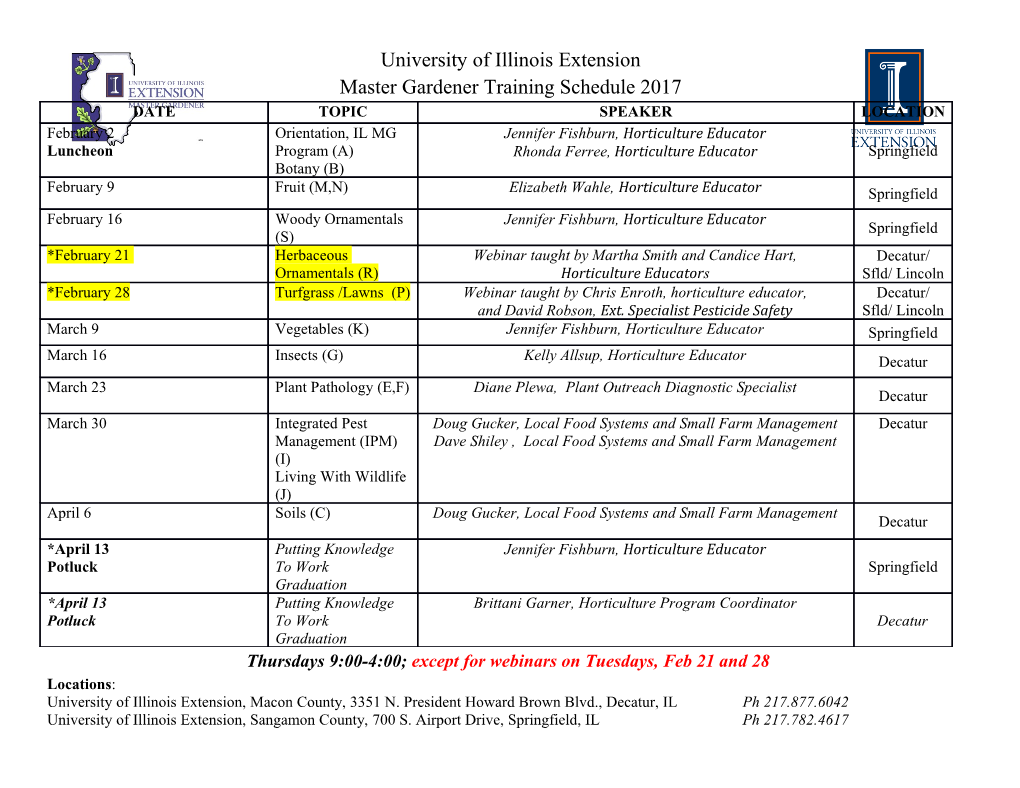
Biometrics & Biostatistics International Journal Research Article Open Access Size-biased discrete-lindley distribution and its applications to model distribution of freely-forming small group size Abstract Volume 7 Issue 2 - 2018 A size-biased discrete-Lindley distribution (SBDLD) has been proposed by size- biasing the discrete Lindley distribution (DLD).The moments about origin and Simon Sium, Rama Shanker moments about mean have been obtained and hence expressions for coefficient of Department of Statistics, College of Science, Eritrea Institute of variation (C.V.), skewness, kurtosis and index of dispersion have been given. The Technology, Asmara, Eritrea estimate of the parameter of SBDLD by both the method of moment and the method of maximum likelihood are the same. Applications of SBDLD have been discussed with Correspondence: Rama Shanker, Department of Statistics, four examples of observed real datasets relating to freely-forming small group size College of Science, Eritrea Institute of Technology, Asmara, at public places. The goodness of fit of SBDLD shows quite satisfactory fit over size Eritrea, Email [email protected] biased Poisson and size-biased Poisson-Lindley Distributions. Received: March 03, 2018 | Published: April 03, 2018 Keywords: Size-biased distribution, Discrete-Lindley distribution, Moments and moments based measures, Estimation of parameter, Goodness of fit Introduction Lindley7 introduced one parameter Lindley distribution having probability density function (pdf) and cumulative distribution function Let a random variable X has probability distribution (cdf). θ 2 Px0 ( ;θθ) ; x= 0,1, 2,..., > 0 . If sample units are weighted or −θ x α f( x;θθ) =(1 +xe) ; x >> 0, 0 (1.2) selected with probability proportional to x , then the corresponding θ + 1 size-biased distribution of order α is given by its probability mass function (pmf) θ x −θ x α Fx( ;θθ )=−+ 1 1 e, x> 0, > 0 (1.3) x⋅ Px0 ( ;θ ) θ = θ + 1 Px1 ( ; ) (1.1) µ′ α Ghitany et al.8 have detailed study on various statistical and ∞ αα mathematical properties, estimation of parameter and application of µθ′ = = ∑ α = Where α E( X) xP0 ( x; ) . When 1, the x=0 Lindley distribution and it has benn showed that Lindley distribution distribution is known as simple size-biased distribution and is gives better fit over exponential distribution to model waiting time applicable for size-biased sampling and forα = 2 , the distribution is data in a bank. Shanker et al.9 have detailed comparative study on known as area-biased distribution and is applicable for area-biased modeling of lifetimes data from engineering and medical science using sampling. In many statistical sampling situations care must be taken both Lindley and exponential distributions and showed that both are so that one does not inadvertently sample from size-biased distribution competing and in majority of datasets exponential distribution gives in place of the one intended. Size-biased distributions are a particular better fit over Lindley distribution. case of weighted distributions which arise naturally in practice Recently, Berhane & Shanker10 introduced discrete-Lindley when observations from a sample are recorded with probability distribution (DLD), a discrete version of Lindley distribution using proportional to some measure of unit size. In field applications, size- infinite series approach, having pmf. biased distributions can arise either because individuals are sampled θ 2 with unequal probability by design or because of unequal detection − (e 1) −θ probability. Size-biased distributions come into play when organisms θθ= +=x > P0 ( x; ) 2θ (1xe) ; x 0,1, 2, 3,...., 0 (1.4) occur in groups, and group size influences the probability of detection. e Fisher1 firstly introduced these distributions to model ascertainment Various statistical properties of DLD, estimation of parameter and biases which were later formalized by Rao2 in a unifying theory applications to model count data have been studied by Berhane & for problems where the observations fall in non-experimental, non- Shanker10 and it has been observed that it gives better fit than both replicated and non-random categories. Size-biased distributions Poisson distribution and Poisson-Lindley distribution, a Poisson have applications in environmental science, econometrics, social mixture of Lindley7 distribution and introduced by Sankaran.11 The science, biomedical science, human demography, ecology, geology, first four moments about origin and the variance of DLD obtained by forestry etc. Further, size-biasing occurs in many unexpected context Berhane & Shanker10 are given by such as statistical estimation, renewal theory, infinite divisibility of 2 µ ′ = distributions and number theory. Many researchers have done work 1 θ , on size-biased distributions including Patil & ord,3 Patil & Rao,4,5 (e − 1) Patil,6 are some among others. Submit Manuscript | http://medcraveonline.com Biom Biostat Int J. 2018;7(2):131–136. 131 © 2018 Sium et al. This is an open access article distributed under the terms of the Creative Commons Attribution License, which permits unrestricted use, distribution, and build upon your work non-commercially. Copyright: Size-biased discrete-lindley distribution and its applications to model distribution of freely-forming small 132 group size ©2018 Sium et al. θ parameterθ has been drawn in Figure 1. It would be recalled that 22(e + ) ′ the pmf of size-biased Poisson-Lindley distribution (SBPLD) having µ2 = , θ 2 parameter θ > 0 given by (e − 1) θ 3 xx( ++θ 2) 2θθ Px;θθ= ;x= 1, 2, 3,..., ;> 0 (2.5) 2(ee++ 74) 3 ( ) x+2 ′ θ + 2 θ + 1 µ3 = ( ) θ 3 (e − 1) 13 has been introduced by Ghitany & Mutairi, which is a size- 32θ θθ biased version of Poisson-Lindley distribution (PLD) introduced 2(eee+ 18 ++ 33 8) by Sankaran.11 Ghitany & Mutairi13 have discussed its various ′ µ4 = , θ 4 mathematical and statistical properties, estimation of the parameter (e − 1) using maximum likelihood estimation and the method of moments, and goodness of fit. Shanker et al.14 has critical study on the θ 2 2e applications of SBPLD for modeling data on thunderstorms and found µσ2 = = , that SBPLD is a better model for thunderstorms than size-biased θ 2 (e − 1) Poisson distribution (SBPD). θθ 21ee( + ) µ3 = , θ 3 (e − 1) θθ2 θ 2ee( ++ 10 e 1) µ4 = θ 4 (e − 1) In this paper, size- biased discrete Lindley distribution has been Figure 1 Behavior of pmf of SBDLD for varying values of the parameterθ . proposed and its moments about origin and moments about mean have been obtained. Behaviors of coefficient of variation, Skewness, Moments kurtosis, and index of dispersion have been discussed graphically for varying values of parameter. The method of moment and the method of The probability generating function (G(t)) and the moment maximum likelihood give the same estimate of the parameter. Finally generating function (M(t)) of SBDLD can be obtained as applications of SBDLD have been discussed with four examples of θ 3 observed real datasets relating to distribution of freely-forming small te(− 1) θ Gt( ) = for t≠ e, (3.1) group size at various public places and the fit by SBDLD has been θ 3 (et− ) observed to be quite satisfactory. and Size-biased discrete-lindley distribution θθ3 2(−t ) (ee− 1) Using (1.1) and (1.4) and the expression for the mean of DLD, = ≠ θ Mt( ) 3 for t . (3.2) θ −t a size-biased discrete-Lindley distribution (SBDLD) with parameter (e − 1) θ > 0 can be defined by its pmf. It can be easily verified that the function in (3.2) is infinitely θ 3 differentiable with respect to t , since it involves exponential terms xP⋅ 0 ( x;θ ) (e − 1) 2 −θ x Px;θ = =+=(xxe ) ; x 1, 2, 3,.., (2.1) ′ 2 ( ) 2θ of its argument. This means that all moments about origin µr ,1r ≥ µ′ 2e th 1 of SBDLD can be obtained. The r moment about origin of SBDLD It can be easily verified that SBDLD is unimodal and have (2.1) can be obtained as θ 3 increasing failure rate. Since e − 1 ∞ '2rr( ) −θ x µ =EX() = ∑ x() x+ x e Px2 ( + 1; θ ) 12 r 2θ = + x=1 θ 1 2e Px( ;θ ) e x 2 θ 3 − ∞∞ (e 1) +−θθ+ − = ∑∑rx12+ r x 2θ ((xe xe 2e xx=11= is a deceasing function of x , Px2 ( ;θ ) is log-concave. Therefore, SBDLD is unimodal, has an increasing failure rate (IFR), and hence Taking r = 1, 2, 3 and 4 and simplifying the complicated and increasing failure rate average (IFRA). It is new better than used in tedious algebraic expression, the first four raw moments (moments expectation (NBUE) and has decreasing mean residual life (DMRL). about the origin) of the SBDLD (2.1) can be obtained as The definitions, concepts and interrelationship between these aging θ 12 e + 2 concepts have been discussed in Barlow & Proschan. µ ' = 1 θ (e − 1) Behavior of the pmf of SBDLD (2.1) for varying values of the Citation: Sium S, Shanker R. Size-biased discrete-lindley distribution and its applications to model distribution of freely-forming small group size. Biom Biostat Int J. 2018;7(2):131–136. DOI: 10.15406/bbij.2018.07.00200 Size-biased discrete-lindley distribution and its applications to model distribution of freely-forming small Copyright: 133 group size ©2018 Sium et al. 2θθ θθ ' ee++74 µ 3ee (+ 1) µ = β =3 = 2 θ 2 1 32 32 (e − 1) µ θ 2 (3e ) 32θ θθ eee+18 ++ 33 8 µ ' = 2θθ 3 θ 3 µ (ee++13 1) (e − 1) β =4 = 2 2 θ θθ θθ 43 2 µ2 3e ' ee++41 171 e ++ 131 e 16 µ = 2 θ 4 θ 4 σ 3e (e − 1) γ = = θθ µ ′ −+ Now, using the relationship between central moments (moments 1 (ee12)( ) about mean) and the raw moments, the central moments of the It can be easily verified that SBDLD is over-dispersed (µσ< 2 ) SBDLD (2.1) can be obtained as θ 3e 2 2 µσ=2 = equi-dispersed (µσ= ) and under-dispersed (µσ> ) for 2 θ 2 (e − 1) ∗ θθ>=<( ) = 1.00505 .
Details
-
File Typepdf
-
Upload Time-
-
Content LanguagesEnglish
-
Upload UserAnonymous/Not logged-in
-
File Pages6 Page
-
File Size-