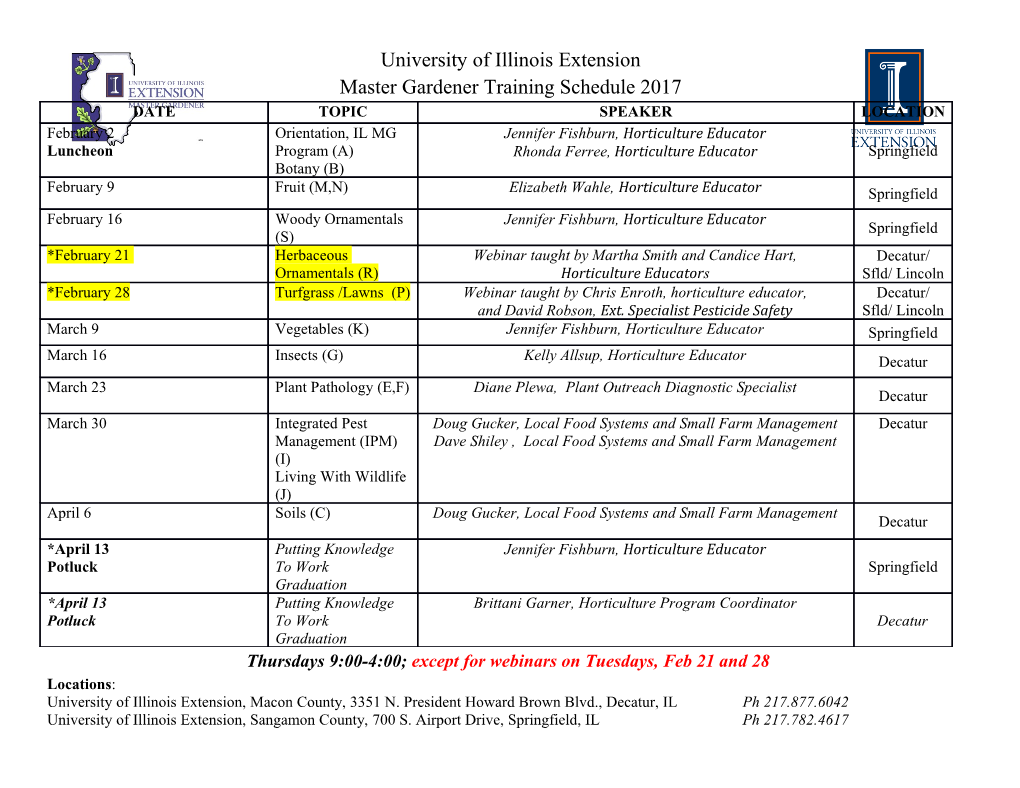
EXPERIMENTAL AND THERAPEUTIC MEDICINE 21: 61, 2021 Interaction network of immune‑associated genes affecting the prognosis of patients with glioblastoma XIAOHONG HOU1*, JIALIN CHEN2*, QIANG ZHANG1, YINCHUN FAN1, CHENGMING XIANG1, GUIYIN ZHOU1, FANG CAO1 and SHENGTAO YAO1 1Department of Cerebrovascular Disease, Affiliated Hospital of Zunyi Medical University;2 Department of Neonatology, The First People' s Hospital of Zunyi Affiliated to Zunyi Medical University, Zunyi, Guizhou 563000, P.R. China Received October 15, 2019; Accepted October 6, 2020 DOI: 10.3892/etm.2020.9493 Abstract. Glioblastoma multiforme (GBM) is a common and immune genes of interest. The interaction network of malignant tumor type of the nervous system. The purpose immune‑regulatory genes constructed in the present study of the present study was to establish a regulatory network of enhances the current understanding of mechanisms associated immune‑associated genes affecting the prognosis of patients with poor prognosis of patients with GBM. The risk score with GBM. The GSE4290, GSE50161 and GSE2223 datasets model established in the present study may be used to evaluate from the Gene Expression Omnibus database were screened the prognosis of patients with GBM. to identify common differentially expressed genes (co‑DEGs). A functional enrichment analysis indicated that the co‑DEGs Introduction were mainly enriched in cell communication, regulation of enzyme activity, immune response, nervous system, cytokine Glioblastoma multiforme (GBM) is one of the most malig‑ signaling in immune system and the AKT signaling pathway. nant tumor types of the central nervous system, with short The co‑DEGs accumulated in immune response were then median survival and poor prognosis. The major treatment is further investigated. For this, the intersection of those combined therapy, including surgery, radiotherapy and chemo‑ co‑DEGs and currently known immune‑regulatory genes therapy (1‑3). In spite of these combinations, the efficacy of was obtained and a differential expression analysis of these GBM treatments remains unsatisfactory, placing a serious overlapping immune‑associated genes was performed. A risk burden on society and affected families (4). model was established using immune‑regulatory genes that In recent years, the significant role of the immune micro‑ affect the prognosis of patients with GBM. The risk score was environment in tumors has been increasingly elucidated. significantly associated with the prognosis of patients with The immune microenvironment has an important role in the GBM and had a significant independent predictive value. The prognosis of patients with GBM (5‑7). In the immune micro‑ risk model had high accuracy in predicting the prognosis of environment of tumors, immune cells and stromal cells have patients with GBM [area under the receiver operating charac‑ an important role and affect the prognosis of patients (8‑13). teristic curve (AUC)=0.764], which was higher than that of a An algorithm called ESTIMATE was designed to predict previously reported model of prognosis‑associated biomarkers immune infiltration by estimating immune cells and stromal (AUC=0.667). Furthermore, an interaction network was cells with specific expression values (14). In addition, tran‑ constructed by using immune‑regulatory genes and transcrip‑ scription factors are able to bind to specific sequences on the tion factors affecting the prognosis of patients with GBM and 5' end of the gene, thereby regulating gene expression (15,16). the University of California Santa Cruz database was used to Transcription factors have a key role in the development of perform a preliminary analysis of the transcription factors GBM (5,17‑19). The era of excessive data has provided a convenient plat‑ form to explore the molecular mechanisms of various diseases. Microarray analysis is of great research and application value and has an important role in the discovery of molecular Correspondence to: Dr Shengtao Yao, Department of Cerebrovas‑ markers for diagnosis and prognosis, as well as novel drug cular Disease, Affiliated Hospital of Zunyi Medical University, 201 Dalian Road, Zunyi, Guizhou 563000, P.R. China targets (20). In the present study, to further investigate the E‑mail: [email protected] association between transcription factors and immune‑regu‑ latory genes, data from the Assay for Transposase‑Accessible *Contributed equally Chromatin (ATAC) database from patients with GBM were analyzed and peak coverage on chromosomes was determined. Key words: immune gene, poor prognosis, glioblastoma, Gene Finally, an interaction network of the prognosis‑associated Expression Omnibus, The Cancer Genome Atlas immune‑regulatory genes and transcription factors was estab‑ lished, providing a possible immune gene regulatory network for genes associated with poor prognosis in GBM. 2 HOU et al: USE OF IMMUNE INTERACTION NETWORK FOR GLIOBLASTOMA PROGNOSIS Materials and methods between the differentially expressed immune genes and tran‑ scription factors with the prognosis of GBM was then performed Data sources. Gene Expression Omnibus (GEO) is a public with a correlation coefficient of the absolute value >0.4 and functional genomic database of integrated gene expres‑ P<0.05 considered to indicate statistical significance. sion data, gene chips and microarrays. The gene expression datasets used in the present study were downloaded from the Construction of Cox risk model. First, the association of immune GEO online platform (https://www.ncbi.nlm.nih.gov/geo/) (21). genes with the prognosis of patients GBM was determined using The selected datasets were GSE2223, including 4 normal univariate Cox regression analysis and a total of 17 prognostic glioma samples and 27 GBM samples, GSE50161, including genes were obtained. Subsequently, multivariate Cox regression 13 normal samples and 34 GBM samples, and GSE4290, analysis was used to construct a best‑fitting prognostic model including 23 normal glioma samples and 81 GBM samples. and a risk score formula was then established by including each To further verify the association between immune‑regulatory of these selected genes [in total, seven key genes were selected genes and GBM, the level‑3 gene expression profile and clinical according to the minimum criteria, these genes were calcium data of patients with GBM were downloaded from The Cancer binding protein a10 (S100A10), mitogen‑activated protein Genome Atlas (TCGA) database (Data release/version 17.0; kinase 3 (MAPK3), serpin family A member 3 (SERPINA3), https://portal.gdc.cancer.gov/). The immune score and stromal Fc fragment of IgG receptor IIb (FCGR2B), endothelin receptor score were determined from data obtained from the database type A (EDNRA) and p21 activated kinase 1 (PAK1) and the using the ESTIMATE algorithm (14). From the TCGA data‑ patients were divided into high/low risk score groups]. Patients base, a small number of non‑cancerous control tissues from were classified into high‑ or low risk‑groups with the median subjects without GBM were available. The most common sites risk score as the cutoff. of GBM are the frontal lobe, temporal lobe and parietal lobe. In order to reduce bias, the corresponding 102 normal samples Multivariate analysis. To determine whether the immune risk were downloaded from the University of California Santa score independently affects the survival of patients with GBM, Cruz (UCSC) database (https://xena.ucsc.edu/) and the data univariate and multivariate Cox proportional hazards model were then corrected using the ‘removevBatchEffect’ func‑ analyses of the influence of risk scores and clinical features of tion of the limma package (22). ATAC with high‑throughput patients with GBM on survival were performed. sequencing (ATAC‑seq) data for patients with GBM were downloaded from the ATAC database (https://gdc.cancer. Interaction network. In the present study, the ‘corrplot’ gov/about‑data/publications/ATACseq‑AWG). package of R software was used for interaction network correlation analysis and a correlation coefficient >0.4 and Differentially expressed genes (DEGs). R software P<0.001 were considered to indicate a significant correlation. (version 3.5.1) was used to analyze the three datasets GSE2223, Subsequently, Cytoscape software (24) was used to construct GSE50161 and GSE4290, as well as the TCGA and GTEX an interaction network for the immune genes associated with data. Screening criteria were set to P<0.05 and log|fold change the prognosis of patients with GBM, differentially expressed (FC)| >1, and finally, the common DEGs (co‑DEGs) of the transcription factors and immune cells from the TIMER data‑ datasets were obtained. base (https://cistrome.shinyapps.io/timer/). Retrieval of immune gene and transcription factor data. ATAC‑seq data analysis. ATAC standardized matrix data were The enrichment analysis of co‑DEGS from the three downloaded from the ATAC database and through ‘TxDb. GEO datasets focused on results associated with immuniza‑ Hsapiens’, ‘UCSC.hg38.knownGene, org.Hs.eg.db’, ‘cluster‑ tion. For further study, tumor‑associated immune genes and Profiler’, ‘karyoploteR’ and ‘ChIPseeker’ package analyses, transcription factors were downloaded from the IMMPORT the distribution of ATAC‑seq peaks on chromosomes was database (https://www.immport.org/) and the Cistrome data‑ determined. To further assess whether Snail family transcrip‑ base (http://cistrome.org/). The DEGs among the downloaded tional repressor 2 (SNAI2)/MYC and C‑X‑C motif chemokine immune
Details
-
File Typepdf
-
Upload Time-
-
Content LanguagesEnglish
-
Upload UserAnonymous/Not logged-in
-
File Pages10 Page
-
File Size-