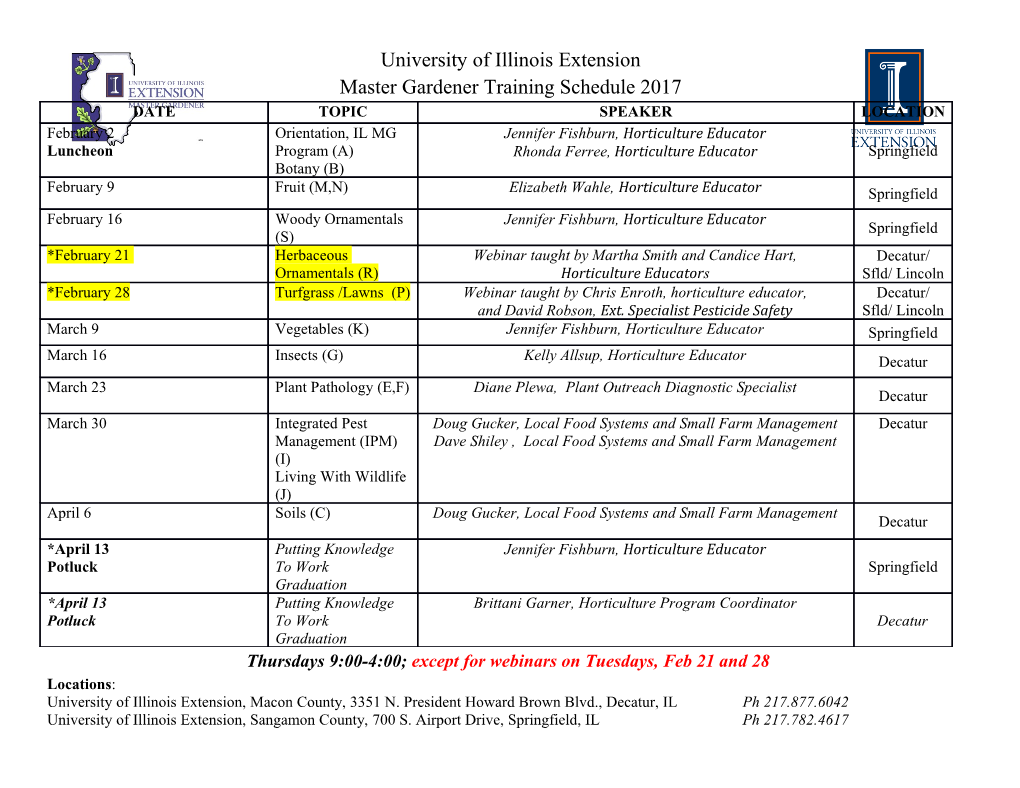
Searching for Snake-in-the-Box Codes with Evolved Pruning Models Daniel R. Tuohy Walter D. Potter and Darren A. Casella Stottler Henke Associates, Inc. Artficial Intelligence Center San Mateo, CA University of Georgia Athens, GA 0111 Abstract We present a method for searching for 0101 achordal open paths (snakes) in n-dimensional hyper- cube graphs (the box). Our technique first obtains a set 0011 0001 of exemplary snakes using an evolutionary algorithm. 1101 1111 These snakes are then analyzed to define a pruning 1001 1011 model that constrains the search space. A depth-first search of the constrained solution space has established 1100 1110 new lower bounds for the length of the longest snakes in 1000 1010 the 9 and 10 dimensional hypercube graphs. 0100 0110 Keywords: Search, Genetic Algorithms, Mathematics 0000 0010 Figure 1: A maximal length snake in Q4 1 Introduction The snake-in-the-box problem is that of discovering 4. We perform a depth first search of all snakes, the longest path in a hypercube graph such that pruning and backtracking whenever any at- the path is not adjacent to itself at any node. The tribute of the current snake escapes the model. longest such path for the dimension-4 hypercube is illustrated in Figure 1. The reader should note that we are not con- cerned with time efficiency. We are interested only For dimensions one through seven, longest max- in obtaining snakes which are longer than the cur- imal snakes have been found by exhaustive search rent theoretical lower bound, and therefore have techniques [7][15]. In higher dimensions, those not yet been discovered either through computa- greater than seven, the solution space is intractable. tional search or mathematical construction. We Several non-exhaustive computational search tech- have achieved this objective for the 9 and 10 di- niques have been employed to discover lengthy mensional hypercube graphs. snakes in these dimensions, including genetic algo- rithms [16], distributed computing [11], neural net- works [4], and other evolutionary algorithms [6]. 2 Applications We have developed a hybrid method described by this sequence of steps: The snake-in-the-box problem was first described in a paper by Kautz in the late 1950s, and was noted 1. An evolutionary algorithm is used to generate for its relevance to coding theory [12]. Snake-in- a set of very long snakes. the-box codes are useful because they are “spread 2” gray codes. This means that any two codewords 2. We compute several attributes of each snake are at least two entries apart in the list of codewords at each node in the snake. or that they differ in at least two positions. Conse- quently, errors at only one position (the most com- 3. The upper and lower bounds of these at- mon case) are easily detectable because they will tributes define a pruning model. reference code words at positions in the list which are more obviously inappropriate [10]. Snakes have Dimension n-snake n-coil been put to use in disjunctive normal form simplifi- cation, electronic combination locking mechanisms, Q1 1 2 disk sector encoding, and analog-to-digital conver- Q2 2 4 sion [14][13][5][15][8]. Q3 4 6 The specific use of snake-in-the-box codes in Q4 7 8 these domains is often error-detection and correc- Q5 13 14 tion, and the longest snakes are the most useful for Q6 26 26 this purpose. Consequently, methods for discov- Q7 50 48 ering the longest snakes in dimensions eight and Q8 97 96 above have been the subject of much research in Q9 186 180 both mathematics and computer science (See refer- Q10 358 344 ences). Q11 680 630 Q12 1260 1238 3 Background and Terminol- Table 1: Longest snakes and coils in Qn. For n≥8, ogy solutions are only the current best-known. Following standard convention, we use Qn to de- “n-coil” to coils of maximal length in Qn. The note the n-dimensional hypercube, which is defined terms “snake” and “n-snake” are used in exactly inductively as the Cartesian product of Q1 and the same way to designate induced open paths. Qn−1. It is useful to think of Q1 as a line (two The lengths of n-snakes and n-coils up to n = 6 constituent nodes), Q2 as a square (four), Q3 as were computed via exhaustive search by Davies [7]. a cube (eight), and Q4 as the sixteen-node graph Those for n = 7 were determined by the Genetic > in Figure 1. For n 4, meaningful visualisation is Algorithm of Potter [16] and later the exhaustive tricky. n technique of Kochut [15], which we will be building There are 2 nodes in Qn that can be represented upon. Table 1 shows the lengths of the n-snakes as vectors of binary digits. The nodes are labeled and n-coils for n≤7, as well as current best-known in such a way that the binary vectors of adjacent lengths of longest snakes and coils in dimensions 8 nodes always differ by exactly one bit, as in Figure through 12 [2][6][7][9][15][16]. 1. An induced path in Qn, as defined in [9], is a sequence of nodes P such that for any u,v∈P, if u 4 PBSHC: An Evolutionary and v are adjacent in Qn then they are also adjacent in P. Algorithm for Obtaining A path is expressed in node sequence representa- Model Snakes tion if it is a vector of base-10 integers correspond- ing to the binary labels on each node in the path. A Population-Based Stochastic Hill-Climber (PB- The node sequence of the snake in Figure 1 is {0 1 SHC) was used to generate our model snakes. This 376141213}. algorithm is described in more detail in [6]. The A path is expressed in transition sequence rep- PBSHC evolves a population of snakes from a zero resentation if it is a vector of integers in the range or small initial length to some maximum length. 0..n-1 [1]. These integers correspond to the index Each individual in the population consists of a of the bit in the bit string that was flipped to grow sequence of integers that represents the node se- the snake from one node to the next. The index of quence of a snake, or valid path through the hyper- the least significant bit is 0 and that of the most cube, in the dimension being searched. These in- significant bit is n-1. The transition sequence of the dividuals are initialized as either a snake of length snake in Figure 1 is {0120310}. zero, that is consisting of only the zero node, or The term “Snake in the Box” (derived from the seeded with a pre-existing snake of choice. Follow- visualization of a chain as a unit-radius tube) orig- ing initialization, the evolutionary cycle begins its inally designated induced cycles, or closed paths first generation. Each generation begins with a fit- (also called closed chains), in a graph [12]. We ness evaluation. The fitness function used is based adopt the terminology introduced in [9], who as- on both the length and the ‘tightness’ of the snake. sign the term “coil” to simple cycles and the term The tightness of a snake, as defined for the PBSHC, is a measure of how many nodes are left available sion eight, unidirectional growth was chosen for this in the hypercube after subtracting all those nodes implementation to best allocate computational re- that are disqualified either by already being in the sources. This operator can be seen to perform a snake or by being adjacent to another node in the stochastic hill-climbing process on each snake in the snake. The choice of tightness as a component of population as the choice of which adjacent node the fitness function was inspired by the idea that to connect to is based on random selection from tighter snakes, since they make more efficient use the available nodes. A choice was made early to of nodes, might have more room to grow. grow all snakes in the population instead of only Initial attempts of integrating both length and the snake of best fitness (note: enhancing the best tightness into a fitness function met with limited individual in a population is a common approach success. Many combinations of linear and non- in hybrid genetic algorithms). This choice was also linear factors of length and tightness for the fitness based on results of a comparison of these two ap- function were tried. While they each performed proaches in an earlier GA implementation. Grow- well in some periods of evolution, they would in- ing all the individuals within the population also evitably perform worse in others. Once the average works well in conjunction with the fitness function fitness of the population slowed, the diversity of the which requires that all snakes in the population of population would fall and the fitness function would a given generation be of the same length in order to converge to a local optimum. Our first attempt at function properly. The fitness function consists of solving this problem was to develop an adaptive fit- the sum of the snake’s length and normalized tight- ness function that would balance between the im- ness. This results in the fitness function simplifying portance of tightness and length, using the diver- to a function of tightness alone as the length compo- sity of the population as the balancing factor. This nent of the fitness value will dominate for snakes of technique also met with limited success, reacting to different lengths, yet cancel for snakes of the same changes in diversity too quickly in some cases and length.
Details
-
File Typepdf
-
Upload Time-
-
Content LanguagesEnglish
-
Upload UserAnonymous/Not logged-in
-
File Pages7 Page
-
File Size-