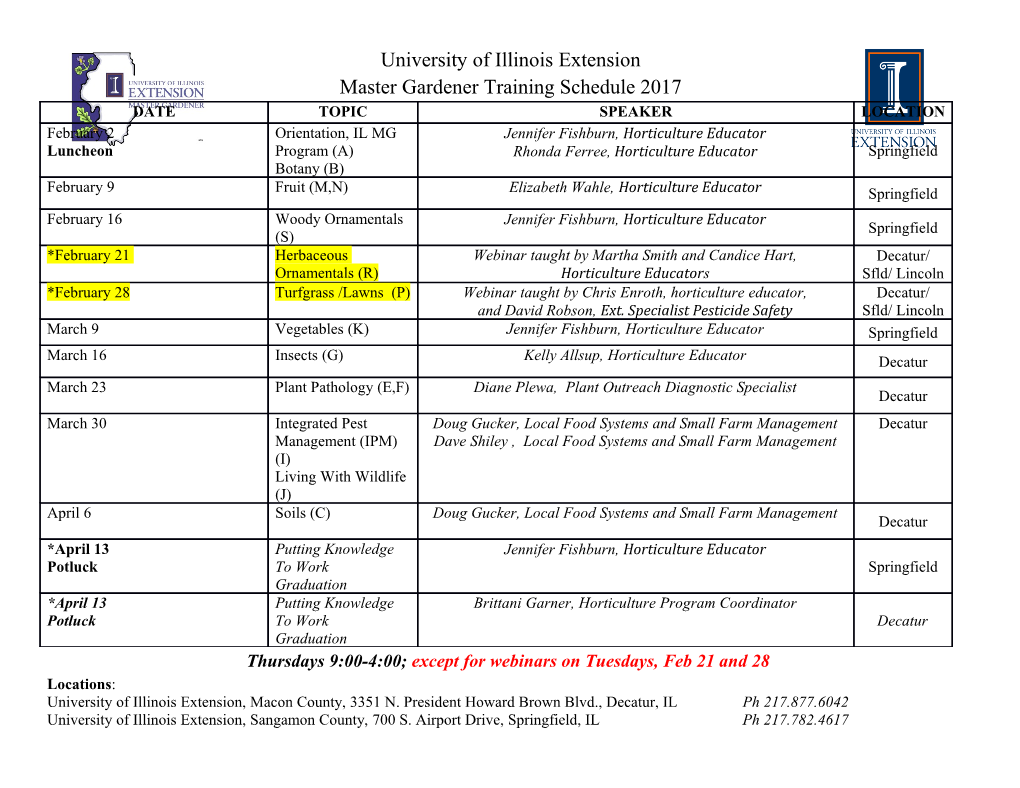
A BLOCK ORTHOGONALIZATION PROCEDURE WITH CONSTANT SYNCHRONIZATION REQUIREMENTS ࣿ y ANDREAS STATHOPOULOS AND KESHENG WU Abstract. We prop ose an alternative orthonormalization metho d that computes the orthonor- mal basis from the right singular vectors of a matrix. Its advantage are: aङ all op erations are matrix-matrix multiplications and thus cache-ecient, bङ only one synchronization p oint is required in parallel implementations, cङ could b e more stable than Gram-Schmidt. In addition, we consider the problem of incremental orthonormalization where a blo ckofvectors is orthonormalized against a previously orthonormal set of vectors and among itself. We solve this problem by alternating itera- tively b etween a phase of Gram-Schmidt and a phase of the new metho d. We provide error analysis and use it to derive b ounds on how accurately the two successive orthonormalization phases should b e p erformed to minimize total work p erformed. Our exp eriments con rm the favorable numerical b ehavior of the new metho d and its e ectiveness on mo dern parallel computers. Key words. Gram-Schmidt, orthogonalization, Householder, QR factorization, singular value decomp osition, Poincare AMS Sub ject Classi cation. 65F15 1. Intro duction. Computing an orthonormal basis from a given set of vectors is a basic computation, common in most scienti c applications. Often, it is also one of the most computationally demanding pro cedures b ecause the vectors are of large dimension, and b ecause the computation scales as the square of the number of vectors involved. Further, among several orthonormalization techniques the ones that ensure high accuracy are the more exp ensive ones. In many applications, orthonormalization o ccurs in an incremental fashion, where a new set of vectors घwe call this internal setङ is orthogonalized against a previously orthonormal set of vectors घwe call this externalङ, and then among themselves. This computation is typical in blo ck Krylov metho ds, where the Krylov basis is expanded by a blo ckofvectors [12, 11]. It is also typical when certain external orthogonalization constraints have to be applied to the vectors of an iterative metho d. Lo cking of converged eigenvectors in eigenvalue iterative metho ds is such an example [19,22]. This problem di ers from the classical QR factorization in that the external set of vectors should not be mo di ed. Therefore, atwo phase pro cess is required; rst orthogonalizing the internal vectors against the external, and second the internal among themselves. Usually, the numb er of the internal vectors is much smaller than the external ones, and signi cantly smaller than their dimension. Another imp ortant di erence is that the accuracy of the R matrix of the QR factorization is not of pri- mary interest, but rather the orthonormalityof the pro duced vectors Q. A variety of orthogonalization techniques exist for b oth phases. For the external phase, Gram- Schmidt घGSङ and its mo di ed version घMGSङ are the most comp etitivechoices. For the internal phase, QR factorization using Householder transformations is the most stable, alb eit more exp ensive metho d [11]. When the number of vectors is signi - cantly smaller than their dimension, MGS or GS with reorthogonalization are usually preferred. Computationally, MGS, GS and Housholder transformations are based on level 1 or level 2 BLAS op erations [15,9,8]. These basic kernel computations, dot pro d- ucts, vector up dates and sometimes matrix-vector op erations, cannot fully utilize the ࣿ Department of Computer Science, College of William and Mary, Williamsburg, Virginia 23187- 8795, घ[email protected]ङ. y NERSC, Lawrence Berkeley National Lab oratory, Berkeley, California 94720, घ[email protected]ङ. 1 pro cessor cache, with signi cant p erformance shortcomings on most of to days com- puters. In addition, these metho ds have high synchronization requirements when implemented on parallel computers. The numb er of synchronization p oints of the GS is linear to the number of vectors, while for the rest metho ds it is quadratic [14]. When the parallel platform involves a high latency network but fast pro cessors, as in the case of the increasingly p opular clusters of workstations [21], the number of synchronization p oints can haveanadverse e ect in the scalability of the metho d. Most of the previous e orts to address these problems considered blo cks of vectors, and used hybrids of the more scalable GS for orthogonalization b etween blo cks, and the more accurate MGS for orthogonalization within blo cks [14, 2]. By adjusting the blo ck size appropriately, these techniques can pro duce accurate orthonormal sets while reducing the synchronization requirements of MGS. However, the number of synchronization p oints is still linear to the number of vectors, and BLAS level 2 kernels are still dominant despite blo cking. Finally, most of such e orts have fo cused on a full QR factorization of a set of vectors, rather than the two phase problem. In this pap er, weintro duce a metho d based on the singular value decomp osition घSVDङ, that uses the right singular vectors to pro duce an orthonormal basis for a given subspace. The idea itself is not new, dating back at least to Poincare, and it is sometimes encountered in chemistry and wavelet literature [5, 20,16,17, 6]. How- ever, it has not received any attention as a computationally viable orthogonalization metho d, and to our knowledge there is no analysis of its numerical prop erties. Beyond theoretical considerations, the metho d, whichwe call SVQB, has some very attractive characteristics. It uses primarily level 3 BLAS kernels, and it has a constantnumber of synchronization p oints. As we show in this pap er, it is not as numerically accurate as MGS, but it is often b etter than GS. More interestingly,we show that more stable alternatives, such as MGS or Householder, are an overkill for our two phase problem. Coupling the SVQB metho d for the internal phase with a blo ck GS with reorthogonal- ization for the external phase results in a metho d also with constant synchronization requirements. The pap er is organized as follows. We rst describ e the basic idea of the SVQB metho d, and we couple it with a blo ck GS for the two phase problem. The numerical stability of the metho d is analyzed in the following section, and some numerical exp er- iments and comparisons with other metho ds verify its e ectiveness. We then analyze the numerical interaction b etween the metho ds used in each of the two phases, and based on this theory we tune the two phases so that they do not p erform unneces- sary reorthogonalizations. Following, we present timings from a series of exp eriments on the Cray T3E, and on the IBM SP-2. These verify that b oth the blo ck computa- tions and the small numb er of synchronizations help the new metho d achieve accurate orthogonality, faster than other comp etitive metho ds. nࣾk 2. Orthogonalization metho ds. Let V 2< b e a set of orthonormal vec- nࣾm tors, and W 2< b e a set of vectors, where k + m ऊ n. In practice, we usually exp ect m < k and m n. The problem is to obtain an orthonormal set of vec- tors Q, such that spanघQङ=spanघW ङ and Q ? V . This problem can be viewed as consisting of two phases: obtaining a Q which is orthogonal to V घexternal phaseङ, and orthonormalizing the Q vectors among themselves घinternal phaseङ. The internal phase problem is most commonly solved through various metho ds for QR factoriza- tion [11,23]. Gram-Schmidt घGSङ is the most well known and also the least computationally exp ensive metho d for QR factorization. In addition, GS is based primarily on level 2 2 BLAS kernels, and it is p ossible to implement it in parallel with a mo dest num- ber of m + 1 synchronization p oints. Although computationally attractive, GS do es not always pro duce numerically orthogonal vectors [2]. However, when used with reorthogonalization, a second GS iteration is typically enough for pro ducing orthog- onality to machine precision [7]. Since a second iteration is not always necessary,GS with reorthogonalization is often preferred over other metho ds. Householder re ections, on the other hand, yield a QR factorization that is nu- merically accurate. By construction, the resulting matrix Q is orthogonal to machine precision घङ, and the computed upp er triangular matrix R di ers from the exact one by less than kAk [11]. Despite its excellentnumerical characteristics, this technique involves twice the arithmetic of the GS. For a slight increase in arithmetic, a blo ck version is also p ossible that facilitates the use of level 3 BLAS kernels. However, when m n, this blo cking is harder to obtain and it is less e ective. Moreover, the number of synchronization p oints it requires grows quadratically with m. Mo di ed Gram-Schmidt घMGSङ improves the numerical stability of the GS by orthogonalizing individual pairs of vectors rather than a vector against a blo ck. For MGS, the error in the orthogonalityof Q can b e b ounded by ऊघW ङ, where ऊघW ङis the condition number of W [1, 3]. The op eration count for MGS is the same with GS, but working on individual vectors allows only for level 1 BLAS kernels, which impairs signi cantly the actual p erformance on cache based computers. Finally, the number of synchronization p oints grows quadratically with m. In the context of the two phase problem, pro ducing an internal set of vectors Q with orthogonality close to machine precision is unnecessary, b ecause of the interde- p endence of the phases.
Details
-
File Typepdf
-
Upload Time-
-
Content LanguagesEnglish
-
Upload UserAnonymous/Not logged-in
-
File Pages20 Page
-
File Size-