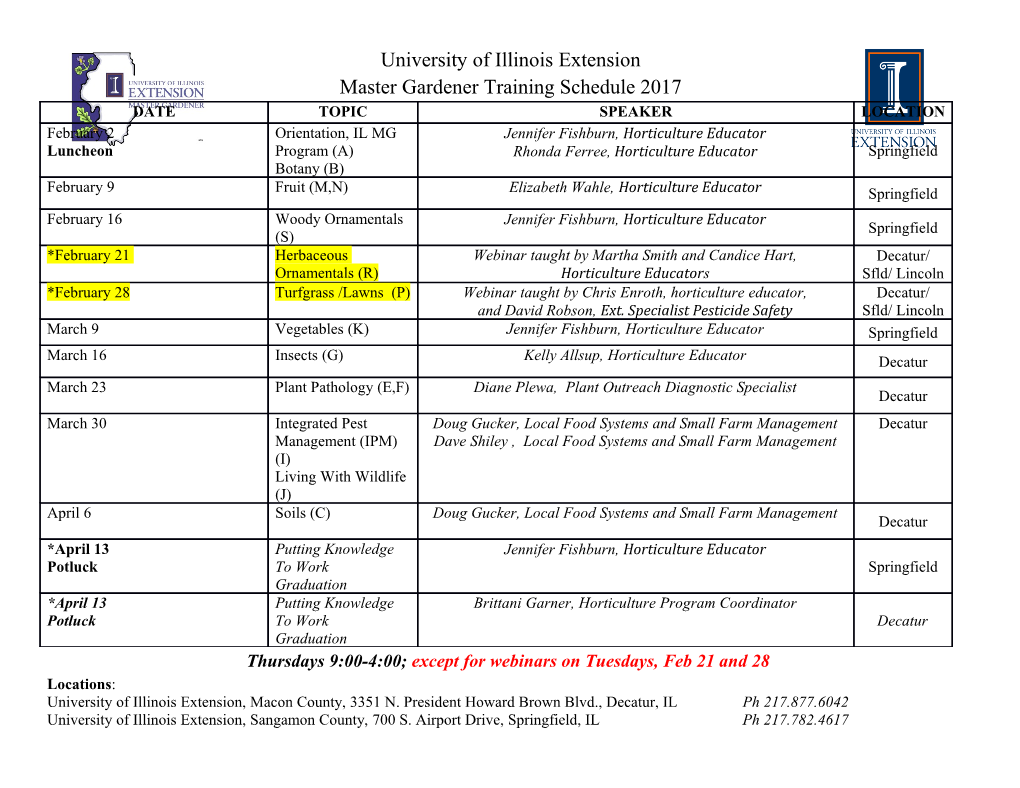
Theoretical Properties of a New Weighted Likelihood Estimator for Right Censored Data Adhidev Biswas, Pratim Guha Niyogi, Suman Majumder, Subir K. Bhandari, Abhik Ghosh and Ayanendranath Basu TECHNICAL REPORT NO. ISRU/2018/2 INTERDISCIPLINARY STATISTICAL RESEARCH UNIT INDIAN STATISTICAL INSTITUTE 203, Barrackpore Trunk Road Kolkata - 700108 INDIA Theoretical Properties of a New Weighted Likelihood Estimator for Right Censored Data Adhidev Biswas, Pratim Guha Niyogi, Suman Majumder, Subir K. Bhandari, Abhik Ghosh and Ayanendranath Basu Abstract As in all cases involving randomly generated real data, the robustness issue is a matter of real concern in the context of right censored survival data. In the present manuscript we consider the weighted likelihood approach considered in Biswas et al.(2015) and Majumder et al.(2016) and apply it in the right censored survival analysis scenario. Important theoretical properties of the weighted likelihood estimator (WLE), such as the influence function (IF) and asymptotic normality, are derived. The resulting function coincides with the IF of the maximum likelihood estimator (MLE) under the true model, thereby indicating the first order efficiency of the proposed method at the model. The same has been reflected in the asymptotic distribution of the estimator, where the asymptotic variance of the WLE coincides with that of the MLE. Taken in conjunction with the strong robustness properties of the WLE, the above results demonstrate the immense potential and utility of the WLE in case of right censored survival data. Keywords: Asymptotic normality, Censoring, Consistency, Influence function, Maximum likelihood estimator, Sub-distribution functions, Weighted likelihood es- timator. 1 Introduction In many scientific experiments, one needs to model real data to understand the laws of nature and to predict the behaviour of the system in future. Right censored data are commonly encountered in many medical and industrial experiments. As in any random dataset generated through a stochastic mechanism, right censored data (in survival or other contexts) may also be prone to (legitimately occurring or spurious) outliers and model misspecifications. The scale and nature of such problems has in- creased and become more complicated in the present age of big data. Sometimes, the 1 problem is further aggravated by incorrect measurements and faulty data recording. Classical procedures often produce questionable estimators and incorrect inference in such cases. This manuscript considers a non-trivial modification of the weighted likelihood estimation scheme as considered in Biswas et al.(2015) and Majumder et al.(2016), tailored to the additional requirement of dealing with the censored observations. The modification is facilitated by the availability of the product-limit survival function estimate as presented in Kaplan and Meier(1958). The rest of the manuscript is organized as follows. Section2 introduces the problem by describing the setup and presents suitable mathematical models. In Section3 we inspect the maximum likelihood estimating equation, which leads to the development of the proposed weighted likelihood scheme in Section4. In this section we also obtain the influence function of the weighted likelihood estimator. Section5 presents the proof of the asymptotic distribution of the weighted likelihood estimator under some standard regularity conditions. 2 The Mathematical Setup We consider data generated in the survival context where each observation is either fully observed or censored randomly (independently of the failure time of the object) to the right. Let us introduce the necessary mathematical structure involved in what follows. Let T1;T2; ··· ;Tn denote the failure times of n objects, coming from the cumu- lative distribution function (CDF) G having a probability density function (PDF) g. The true density g is modelled by some suitable parametric family of densities ffθ : θ 2 Θg. But, in the right-censored context, we also have to deal with the censor- ing variable C which is assumed to have CDF FC and PDF fC . Let C1;C2; ··· ;Cn denote the independent and identically distributed (i.i.d.) observations from fC . Here we assume that the censoring distribution C does not contain any information about the model parameter θ. So, we essentially observe the variables Xi = minfTi;Cig and ∆i = 1fTi6Cig; i 2 f1; 2; ··· ; ng; (1) where 1A is the indicator function for the event A. We now have i.i.d. pairs of observations f(Xi; ∆i); i 2 f1; 2; ··· ; ngg, on the basis of which we wish to obtain a robust weighted likelihood estimator of the parameter θ. For the sake of complete- ness, we start with the description of the maximum likelihood estimator (MLE) in the next section. 3 The Likelihood Equation We shall first calculate the following quantities required for the expression of the likelihood function. For the ith observation, we consider two quantities, termed as 2 sub-distribution functions (Reid, 1981), as defined by u F (x) = P[Xi 6 x; ∆i = 1] = P[Ti 6 x; Ti 6 Ci] Z x Z x = P[Ci > t]g(t)dt = SC (t)g(t)dt; (2) 0 0 and c F (x) = P[Xi 6 x; ∆i = 0] = P[Ci 6 x; Ti > Ci] Z x Z x ¯ = P[Ti > t]fC (t)dt = G(t)fC (t)dt: (3) 0 0 ¯ Here, G = 1 − G and SC = 1 − FC denote the survival functions of T and C, respectively. Moreover, note that Z 1 Z 1 P[∆i = 1] = P[Ti 6 Ci] = P[Ci > t]g(t)dt = SC (t)g(t)dt; (4) 0 0 and Z 1 Z 1 ¯ P[∆i = 0] = P[Ti > Ci] = P[Ti > t]fC (t)dt = G(t)fC (t)dt: (5) 0 0 Combining the above results, we obtain the conditional distribution of Xi given ∆i as S (x )dG(x ) δi G¯(x )dF (x ) 1−δi dF (x jδ ) = C i i × i C i ; δ 2 f0; 1g: (6) Xij∆i i i R 1 R 1 ¯ i 0 SC (t)dG(t) 0 G(t)dFC (t) The likelihood function of θ, given the observations x = (x1; x2; ··· ; xn) and δ = (δ1; δ2; ··· ; δn), is evaluated by substituting Fθ in place of G, and has the form n Y δi 1−δi L(θjx; δ) = (SC (xi)fθ(xi)) × (Sθ(xi)fC (xi)) ; (7) i=1 where Sθ = 1 − Fθ with Fθ being the model CDF corresponding to the density fθ. Then, the log-likelihood is given by n X l(θ) ≡ l(θjx; δ) = fδi log fθ(xi) + (1 − δi) log Sθ(xi)g i=1 n X + fδi log SC (xi) + (1 − δi) log fC (xi)g: (8) i=1 We obtain the maximum likelihood equation of θ by maximizing this log-likelihood with respect to θ 2 Θ or, equivalently, solving the estimating equation given by n n X X rl(θ) = fδiu1,θ(xi) + (1 − δi)u2,θ(xi)g = Uθ(xi; δi) = 0; (9) i=1 i=1 3 where r represents derivative with respect to θ, u1,θ(x) = r log fθ(x), u2,θ(x) = r log Sθ(x) and ( u1,θ(x); if δ = 1 Uθ(x; δ) = u2,θ(x); if δ = 0: In order to obtain the IF, we need to consider the likelihood functional based on the CDF’s G and FC . This can be defined as the solution θ to the population estimating equation given by ZZ Uθ(x; δ)dFXj∆(xjδ)dP(δ) = 0; (10) or, Z Z u1,θ(x)dFXj∆(xj1)P(∆ = 1) + u2,θ(x)dFXj∆(xj0)P(∆ = 0) = 0 X X or, Z Z u c u1,θ(x)dF (x) + u2,θ(x)dF (x) = 0: (11) X X It can easily be verified that replacing F u and F c by their respective empirical ^u ^c counterparts Fn and Fn will lead to the likelihood equation (9). 4 Robust Weighted Likelihood Functional: Influ- ence Function Analysis In Section3, we considered the likelihood equation and derived the implicit definition which gives rise to the likelihood functional. In this section, we are going to extend this idea to obtain a robust weighted likelihood functional for the censored data case. Given the setup in Section2, let us again start with the data of the form (1) and follow the construction of the likelihood function in the same line mentioned in Section3. However, in order to control the influence of outlying observations, we introduce the weight function following the idea and rationale of Biswas et al.(2015) and Majumder et al.(2016). While defining the residual function in the present right-censored case, we also need to take into account the possibility of data points being censored (eg. lost to follow-up). As a remedy, we consider the product-limit estimator of the survival function (and likewise, that of the distribution function) introduced in Kaplan and Meier(1958) and define the residual function as follows. 8 0; if p < F (x) < 1 − p > θ > ^ <>FKM(x) − 1; if Fθ(x) 6 p τKM,θ(x) = Fθ(x) (12) > ^∗ >SKM(x) > − 1; if Fθ(x) > 1 − p; : Sθ(x) 4 ^∗ where SKM(x) is the product-limit estimator of P(T > x). In subsequent discussion, 1 the parameter p has been fixed at . 2 Using the same idea as in Biswas et al.(2015) and Majumder et al.(2016), the weight function is defined as a sufficiently smooth, unimodal, positive function H(·) of the residual in (12), with right tail decaying to zero. We now define our weighted likelihood estimating equation by modifying the likelihood equation (9) as below. n X H(τKM,θ(Xi))Uθ(Xi; ∆i) = 0: (13) i=1 ^ Hereafter, by weighted likelihood estimator (to be denoted by θn;WLE), we define any solution of the weighted likelihood estimating equation (13).
Details
-
File Typepdf
-
Upload Time-
-
Content LanguagesEnglish
-
Upload UserAnonymous/Not logged-in
-
File Pages21 Page
-
File Size-