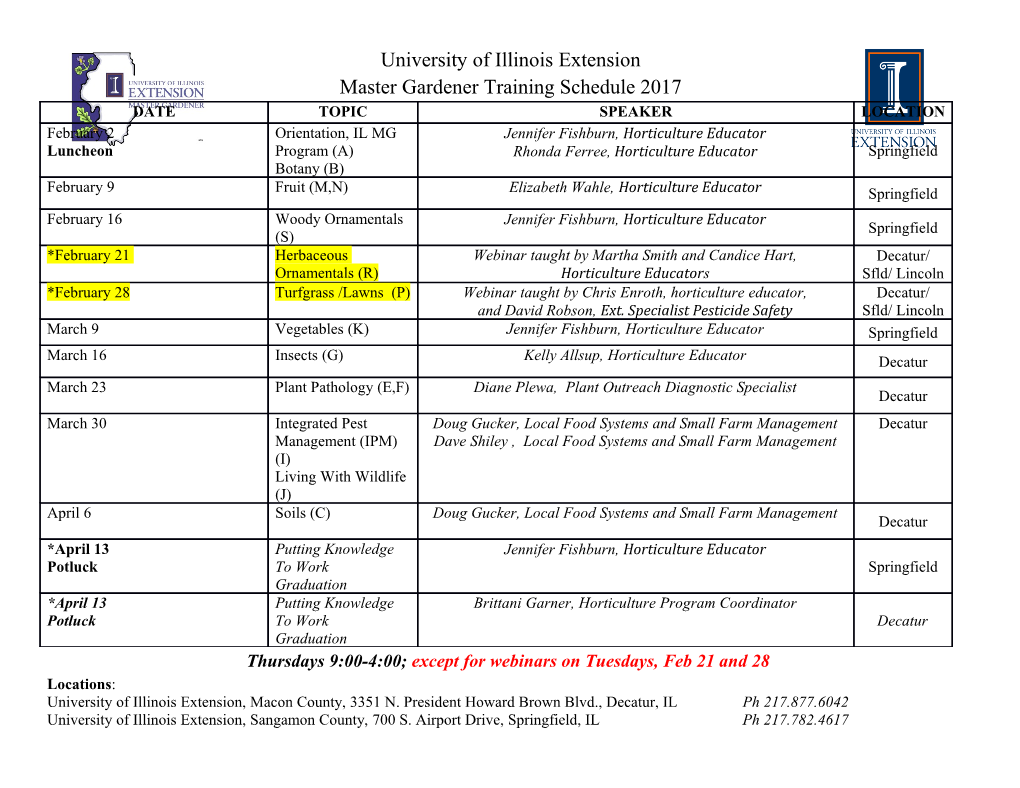
Supplementary Notes on Linear Algebra Mariusz Wodzicki May 3, 2015 1 Vector spaces 1.1 Coordinatization of a vector space 1.1.1 Given a basis B = fb1,..., bng in a vector space V , any vector v 2 V can be represented as a linear combination v = b1b1 + ··· + bnbn (1) and this representation is unique, i.e., there is only one sequence of coeffi- cients b1, ··· , bn for which (1) holds. 1.1.2 The correspondence between vectors in V and the coefficients in the ex- pansion (1) defines n real valued functions on V , ∗ ∗ bi : V ! R, bi (v) ˜ bi, (i = 1, . , n).(2) 1.1.3 If 0 0 0 v = b1b1 + ··· + bnbn (3) is another vector, then 0 0 0 v + v = (b1 + b1)b1 + ··· + (bn + bn)bn which shows that ∗ 0 0 ∗ ∗ 0 bi (v + v ) = bi + bi = bi (v) + bi (v ), (i = 1, . , n). Similarly, for any number a, one has av = (ab1)b1 + ··· + (abn)bn which shows that ∗ ∗ bi (av) = abi (v), (i = 1, . , n). 3 ∗ In other words, each function bi : V ! R is a linear transformation from the vector space V to the one-dimensional vector space R, and the corre- spondence 2 3 b1 ˜ 6 . 7 v 7−! [v]B 4 . 5 (4) bn is a linear transformation from V to the n-dimensional vecor space of column vectors Rn . 1.1.4 The coordinatization isomorphism of V with Rn The kernel of (4) is f0g since vectors b1,..., bn are linearly independent. n The range of (4) is the whole R since vectors b1,..., bn span V . Thus, the n correspondence v 7! [v]B identifies V with the vector space R . We shall refer to (4) as the coordinatization of the vector space V in basis B. _ 1.2 The dual space V 1.2.1 Linear transformations V ! R are referred to as (linear) functionals on V (they are also called linear forms on V ). Linear functionals form a vector _ space of their own which is called the dual of V . We will denote it V (pronounce it “V dual” or “V check”). 1.2.2 An example: the trace of a matrix The trace of an n × n matrix A = aij is the sum of the diagonal entries, tr A ˜ a11 + ··· + ann.(5) The correspondence A 7! tr A is a linear functional on the vector space Matn(R) of n × n matrices. Exercise 1 Calculate both tr AB and tr BA and show that tr AB = tr BA (6) where 2 3 a11 ... a1n 6 . 7 A = 4 . 5 (7) am1 ... amn 4 denotes an arbitrary m × n matrix and 2 3 b11 ... b1m 6 . 7 B = 4 . 5 (8) bn1 ... bnm denotes an arbitrary n × m matrix. 1.2.3 An example: the dual of the space of m × n matrices For any n × m matrix (8), let us consider the linear functional on the space of m × n matrices: fB : A 7−! tr AB (A 2 Matmn(R)).(9) t t Exercise 2 Calculate fB(B ), where B denotes the transpose of B, and show that it vanishes if and only if B = 0. Deduce that fB = 0 if and only if B = 0. 1.2.4 The correspondence _ f : Matnm(R) −! Matmn(R) , B 7−! fB,(10) is a natural linear transformation from the space of n × m matrices into the dual of the space of m × n matrices. In view of Exercise 2 it is injective. By considering bases in V , in the next sections we will show that _ the dimension of V equals the dimension of V if the latter is finite. In _ particular, this will imply that dim Matmn(R) = dim Matmn(R). Since the transposition of matrices, t A 7−! A (A 2 Matmn(R)), is an isomorphism of vector spaces, it will follow that _ dim Matnm(R) = dim Matmn(R) . A corollary of this is that the dual space Matmn(R) is naturally identified with the vector space of n × m matrices, via identification (10). 5 1.2.5 The duality between the spaces of row and column vectors n In particular, the space of column vectors R = Matn1(R) is naturaly identified with the dual of the space of row vectors Mat1n(R) and, vice- versa, the space of row vectors Mat1n(R) is naturally identified with the n dual of the space of column vectors R = Matn1(R). 1.2.6 The coordinate functionals The coordinatization isomorphism of V with Rn is made up of n coordi- _ nate functionals, cf. (2). They span V . Indeed, given a linear functional f : V ! R, let a1 ˜ f(b1),..., an ˜ f(bn). Then, for any vector v 2 V , one has f(v) = f(b1b1 + ··· + bnbn) = b1f(b1) + ··· + bnf(bn) = b1a1 + ··· + bnan = a1b1 + ··· + anbn ∗ ∗ = a1b1 (v) + ··· + anbn(v) ∗ ∗ = a1b1 + ··· + anbn (v) which shows that the linear functional f is a linear combination of func- ∗ ∗ tionals b1,..., bn , ∗ ∗ f = a1b1 + ··· + anbn. 1.2.7 ∗ ∗ The coordinate functionals b1,..., bn are linearly independent. Indeed, if a linear combination ∗ ∗ a1b1 + ··· anbn is the zero functional, then its values on v = b1,..., bn are all zero. But those values are: a1,..., an, since ( ∗ 1 if i = j bi (bj) = .(11) 0 if i , j 6 1.2.8 The dual basis B∗ ∗ ˜ ∗ ∗ Thus, B fb1,..., bng forms a basis of the dual space. Note, that _ dim V = dim V. 1.3 Scalar products 1.3.1 Bilinear pairings A function of two vector arguments h , i : V × V ! R (12) is said to be a bilinear pairing on V if it is a linear functional in each argument. (Bilinear pairings are also called bilinear forms on V .) 1.3.2 We say that the bilinear pairing is nondegenerate if, for any nonzero vector v 2 V , there exists v0 2 V , such that hv, v0i , 0. 1.3.3 We say that the bilinear pairing is symmetric if, for any vectors v, v0 2 V , one has hv0, vi = hv, v0i. 1.3.4 Orthogonality We say that vectors v and v0 are orthogonal if hv, v0i = 0. We denote this fact by v ? v0 . 1.3.5 If X is a subset of V , the set of vectors orthogonal to every element of X is denoted X? ˜ fv 2 V j v ? x for all x 2 X g (13) Exercise 3 Show that X? is a vector subspace of V and X ⊆ X??.(14) 7 1.3.6 We say that the bilinear pairing is positively defined if, for any vector v 2 V , one has hv, vi ≥ 0. Theorem 1.1 (The Cauchy-Schwarz Inequality) Let h , i be a positively de- fined symmetric bilinear pairing on a vector space V . Then, for any vectors v, v0 2 V , one has the following inequality hv, v0i2 ≤ hv, vihv0, v0i.(15) 1.3.7 We shall demonstrate (15) by considering the second degree polynomial p(t) ˜ htv + v0, tv + v0i = hv, vit2 + hv, v0i + hv0, vit + hv0, v0i = at2 + bt + c where a = hv, vi, b = 2hv, v0i and c = hv0, v0i. In view of the hypothesis, p(t) ≥ 0 for all real number t. This is equivalent to the inequality b2 ≤ 4ac which yields inequality (15). 1.3.8 An immediate corollary of the Cauchy-Schwarz Inequality is that a sym- metric bilinear pairing is nondegenerate and positively defined if and only if hv, vi > 0 for any nonzero vector in V . 1.3.9 Scalar products A nondegenerate positively defined symmetric bilinear pairing on V is called a scalar product. Exercise 4 Show that a set of nonzero vectors fv1,..., vng, mutually orthogonal with respect to some scalar product on V , is linearly independent. (Hint: for a linear combination representing the zero vector, a1vq + ··· + anvn = 0 calculate the scalar product of both sides with each vi .) 8 1.3.10 The associated norm For any scalar product, the functional q v 7−! kvk ˜ hv, vi (16) is called the associated norm. Using the norm notation, we can rewrite the Cauchy-Schwarz Inequality as 0 0 hv, v i ≤ kvkkv k.(17) 1.3.11 The Triangle Inequality Note that kv + v0k2 = kvk2 + 2hv, v0i + kv0k2 while 2 kvk + kv0k = kvk2 + 2kvkkv0k + kv0k2. In view of the Cauchy-Schwarz Inequality, the bottom expression is not less than the top expression. Equivalently, kv + v0k ≤ kvk + kv0k,(18) for any pair of vectors v and v0 in V . This is known as the Triangle Inequality. 1.3.12 The associated norm satisfies also the following two conditions kavk = jajkvk,(19) for any real number a and any vector v 2 V , and kvk > 0 (20) for any nonzero vector v 2 V . 1.3.13 Norms on a vector space Any function V −! [0, ¥] that satisfies the Triangle Inequality (18) and conditions (19) and (20) is called a norm on V . 9 1.3.14 Polarization Formula In terms of the associated norm, the scalar product is expressed by means of the identity 1 hv, v0i = kv + v0k2 − kvk2 − kv0k2.(21) 2 known as the Polarization Formula. If a norm k k on a vector space V is associated with a scalar product, then the right-hand-side of (21) must depend on v linearly. If it does not, then that norm is not associated with a scalar product. 1.3.15 Quadratic forms A function q : V ! R is called a quadratic form if the pairing assigning the number 1 hv, v0i ˜ q(v + v0) − q(v) − q(v0) (22) 2 to a pair of vectors v and v0 in V , is bilinear.
Details
-
File Typepdf
-
Upload Time-
-
Content LanguagesEnglish
-
Upload UserAnonymous/Not logged-in
-
File Pages46 Page
-
File Size-