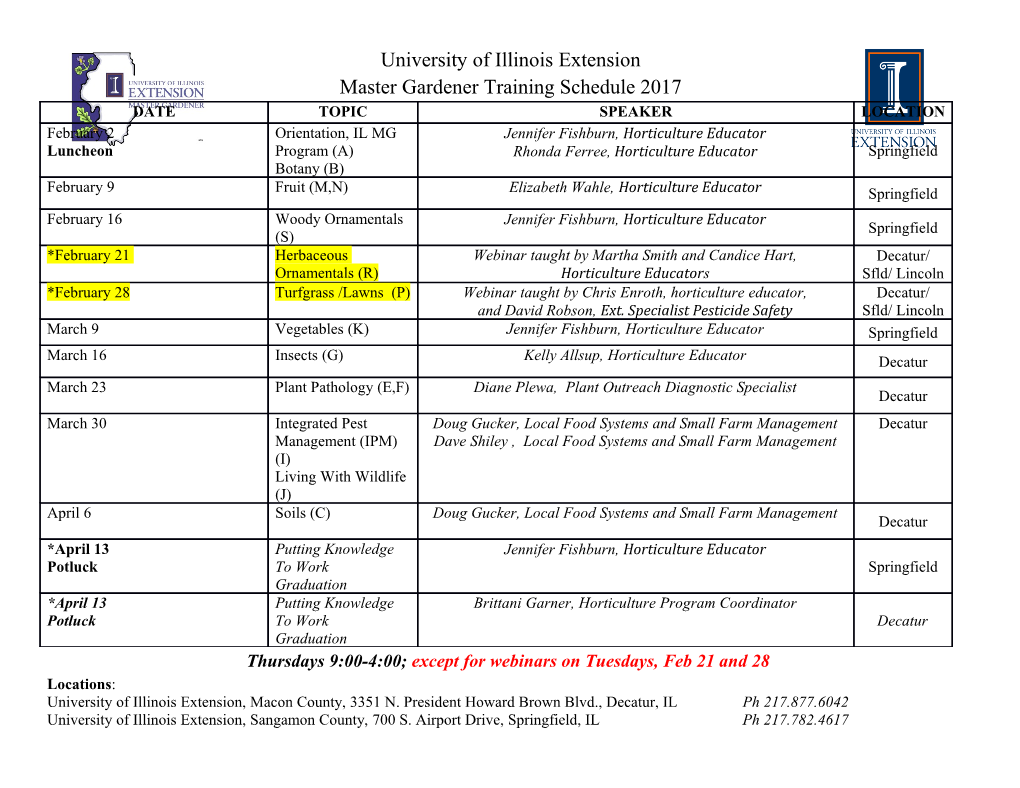
Using Prole Information to Assist Classic Co de Optimizations Pohua P Chang Scott A Mahlke and Wenmei W Hwu Center for Reliable and Highp erformance Computing University of Illinois UrbanaChampaign hwucrhcuiucedu SUMMARY This pap er describ es the design and implementation of an optimizing compiler that automati cally generates prole information to assist classic co de optimizations This compiler contains two new comp onents an execution proler and a prolebased co de optimizer which are not commonly found in traditional optimizing compilers The execution proler inserts prob es into the input pro gram executes the input program for several inputs accumulates prole information and supplies this information to the optimizer The prolebased co de optimizer uses the prole information to exp ose new optimization opp ortunities that are not visible to traditional global optimization metho ds Exp erimental results show that the prolebased co de optimizer signicantly improves the p erformance of pro duction C programs that have already b een optimized by a highquality global co de optimizer Key Words C co de optimization compiler prolebased co de optimization proler INTRODUCTION The ma jor ob jective of co de optimizations is to reduce the execution time Some classic co de optimizations such as dead co de elimination common sub expression elimination and copy propa gation reduce the execution time byremoving redundant computation Other co de optimizations suchasloopinvariant co de removal and lo op induction variable elimination reduce the execution time bymoving instructions from frequently executed program regions to infrequently executed program regions This pap er describ es an optimizing compiler that accurately identies frequently executed program paths and optimizes them To app ear Software Practice Exp erience Static analysis such as lo op detection can estimate execution counts but the estimates are imprecise outcome of conditional statements lo op iteration counts and recursion depth are rarely predictable using static techniques For example a lo op nested within a conditional statementdoes not contribute to the execution time if the condition for its evaluation is never true Optimizing such a lo op may degrade the overall program p erformance if it increases the execution time of other parts of the program Classic co de optimizations use other static analysis metho ds suchaslivevariable analysis reaching denitions and denitionuse chain to ensure the correctness of co de transformations These static analysis metho ds do not distinguish b etween frequently and infrequently executed program paths However there are often instances where a value is destroyed on an infrequently executed path which exists to handle rare events As a result one cannot apply optimizations to the frequently executed paths unless the infrequently executed paths are systematically excluded from the analysis This requires an accurate estimate of the program runtime b ehavior Proling is the pro cess of selecting a set of inputs for a program executing the program with these inputs and recording the runtime b ehavior of the program By carefully selecting inputs one can derive accurate estimate of program runtime b ehavior with proling The motivation to integrate a proler into a C compiler is to guide the co de optimizations with prole information We refer to this scheme as prolebasedcode optimization In this pap er we present a new metho d for using prole information to assist classic co de optimizations The idea is to transform the control ow graph according to the prole information so that the optimizations are not hindered byrare conditions Because prolebased co de optimizations demand less work from the user than hand tuning of a program do es prolebased co de optimizations can b e applied to very large application In this pap er we assume that the reader is familiar with the static analysis metho ds To app ear Software Practice Exp erience programs With prolebased co de optimizations much of the tedious work can b e eliminated from the handtuning pro cess The programmers can concentrate on more intellectual work suchas algorithm tuning The contribution of this pap er is a description of our exp erience with the generation and use of prole information in an optimizing C compiler The prototyp e proler that wehave constructed is robust and tested with large C programs Wehave mo died many classic co de optimizations to use prole information Exp erimental data show that these co de optimizations can substantially sp eedup realistic nonnumeric C application programs We also provide insightinto why these co de optimizations are eective The intended audience of this pap er is optimizing compiler designers and pro duction software develop ers Compiler designers can repro duce the techniques that are describ ed in this pap er Pro duction software develop ers can evaluate the costeectiveness of prolebased co de optimizations for improving pro duct p erformance RELATED STUDIES Using prole information to handtune algorithms and programs has b ecome a common practice for serious program develop ers Several UNIX prolers are available suchaspr of g pr of and tcov The pr of output shows the execution time and the invo cation count of each function The gprof output not only shows the execution time and the invo cation countofeach function but also shows the eect of called functions in the prole of each caller The tcov output is an annotated listing of the source program The execution countofeach straigh tline segmentofC It should b e noted that prolebased co de optimizations are not alternatives to conventional optimizations but are meant to b e applied in addition to conventional optimizations UNIX is a Trademark of ATT To app ear Software Practice Exp erience statements is rep orted These proling to ols allow programmers to identify the most imp ortant functions and the most frequently executed regions in the functions Recent studies of prolebased co de optimizations have provided solutions to sp ecic architec tural problems The accuracy of branch prediction is imp ortant to the p erformance of pip elin ed pro cessors that use the squashing branchscheme It has b een shown that prolebased branch prediction at compile time p erforms as well as the b est hardware schemes Trace scheduling is a p opular global micro co de compaction technique For trace scheduling to b e eective the compiler must b e able to identify frequently executed sequences of basic blo cks It has b een shown that proling is an eective metho d to identify frequently executed sequences of basic blo cks in aow graph Instruction placement is a co de optimization that arranges the basic blo cks of a ow graph in a particular linear order to maximize the sequential lo cality and to reduce the numb er of executed branch instructions It has b een shown that proling is an eective metho d to guide instruction placement A C compiler can implementamultiway branch ie a sw itch statement in C as a sequence of branch instructions or as a hash table lo okup jump If most o ccurrences are satised by few case conditions then it is b etter to implement a sequence of branch instructions starting from the most likely case to the least likely case Otherwise it is b etter to implement a hash table lo okup jump Prole information can help a register allo cator to identify the frequently accessed variables Function inline expansion eliminates the overhead of function calls and enlarges the scop e of global co de optimizations Using prole information the compiler can identify the most frequently invoked calls and determine the b est expansion sequence A counterbased execution proler that measures the average execution times and their variance can b e optimized to achieve a run time overhead less than The estimated execution times can b e used to guide program To app ear Software Practice Exp erience partitioning and scheduling for multipro cessors DESIGN OVERVIEW C programs Input data BoxA BoxC Compiler Proler Frontend Intermediate Co de BoxB Co de Co de Generator Optimizer AMDk MIPS SPARC i Host Assemblers Figure A blo ck diagram of our prototyp e C compiler Figure shows the ma jor comp onents of our prototyp e C compiler Box A contains the com piler frontend and the co de generator Box B is the global co de optimizer that op erates on the intermediate form Table lists the lo cal and global co de optimizations that wehave implemented in our prototyp e compiler In order to have prolebased co de optimizations wehave added a new To app ear Software Practice Exp erience Box C to the prototyp e compiler The prole information is then integrated into the intermediate co de Some co de optimizations in Box B are mo died to use the prole information These co de optimizations form a separate pass that is p erformed after the classic global co de optimizations Our prototyp e compiler generates co de for several existing pro cessor architectures MIPS R SPARC Intel i and AMDk local global constant propagation constant propagation copy propagation copy propagation common sub expression elimination common sub expression elimination redundant load elimination redundant load elimination redundant store elimination redundant store elimination constant folding lo op unrolling strength reduction lo op invariantcoderemoval constantcombining lo op induction strength reduction op eration folding lo op induction elimination dead co de removal dead co de removal co de reordering global variable migration Table
Details
-
File Typepdf
-
Upload Time-
-
Content LanguagesEnglish
-
Upload UserAnonymous/Not logged-in
-
File Pages33 Page
-
File Size-