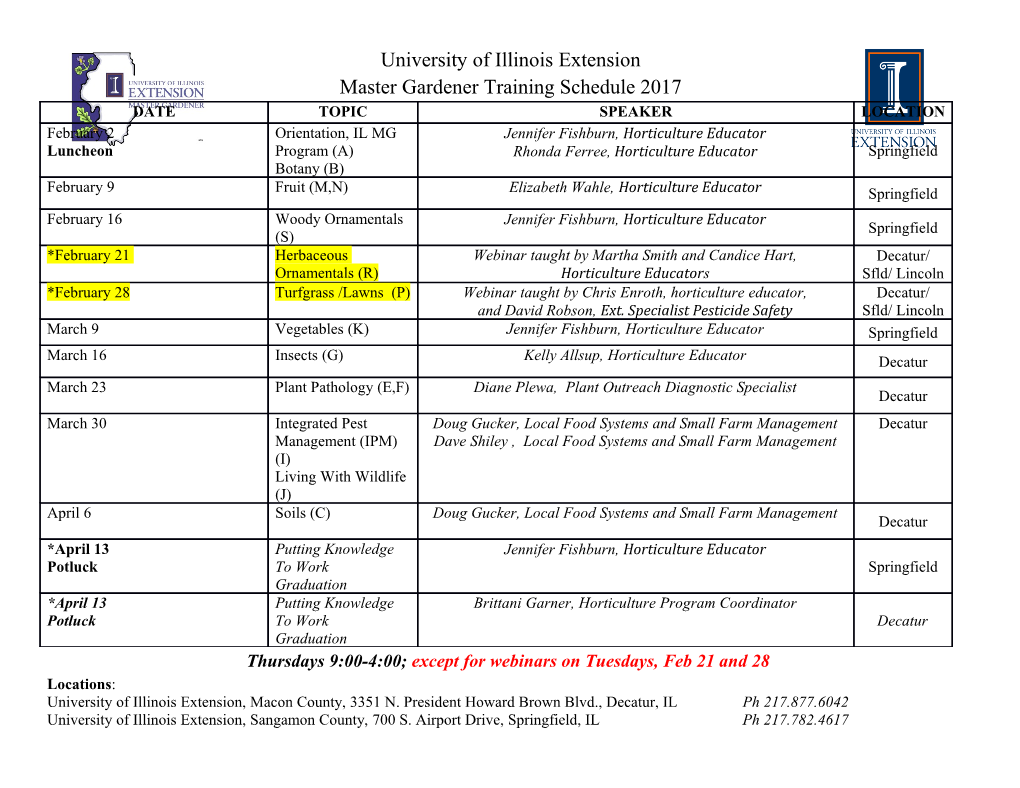
Photos placed in horizontal position with even amount of white space between photos and header Machine Learning Tutorial Danny Dunlavy, 01461 Sandia National Laboratories is a multimission laboratory managed and operated by National Technology and Engineering Solutions of Sandia, LLC, a wholly owned subsidiary of Honeywell International, Inc., for the U.S. Department of Energy’s National Nuclear Security Administration under contract DE-NA0003525. SAND2018-7925 TR Goals for this Tutorial § Introduction to main concepts in Machine Learning § Preparation for participation in MLDL Workshop Caveats § Awareness stressed over education § Neural Networks/Deep Learning mostly avoided § Deep Learning Tutorial: Thursday, July 19, 2018 7/18/18 ML Tutorial 2 Machine Learning A computer program is said to learn from experience E with respect to some class of tasks T and performance measure P, if its performance at tasks in T, as measured by P, improves with experience E. --Tom Mitchell, Machine Learning, 1997 7/18/18 ML Tutorial 3 Example: Handwriting Recognition § Task (T): § recognizing and classifying handwritten numbers within images § Performance measure (P): § percent of numbers correctly classified § Experience (E): § a database of handwritten numbers with given classifications Example adapted from Tom Mitchell, Machine Learning, 1997 Data from MNIST database, http://yann.lecun.com/exdb/mnist/ 7/18/18 ML Tutorial 4 Example ML Workflow Data Features Model Solution Evaluation Instance Label Label Correct 5 0 87% 0 1 96% 4 2 84% . 1 3 82% . 3 0 4 1 7/18/18 ML Tutorial 5 Feature Engineering § Feature engineering is the process of using domain knowledge to create feature § Often manual, time-consuming process § Many machine learning algorithms take vectors as inputs § Raw data often is not in vector format § For many data types, there are existing conventions for creating feature vectors Pedro Domingos. 2012. A few useful things to know about machine learning. Communications of the ACM, 55(10), 78-87. 7/18/18 ML Tutorial 6 Feature Vectors: Images Pixel Values (vectorized) Image Processing Features (Feature Detectors) § Edge, corner, blob, ridge detection § Histogram of Oriented Gradients (HoG) § Hu’s Invariant Moments § Local binary patterns (LBP) § Hough transform . Example Software: Python: scikit-image Matlab: Image Processing Toolbox Julia: JuliaImages (ImageFeatures) R: https://github.com/bnosac/image Reference: Image Feature Detectors and Descriptors. Eds. Awad Image from Matlab 2018a demo: street1.jpg and Hassaballah, Springer, 2016. 7/18/18 ML Tutorial 7 Works generally with Feature Vectors: Text counts of observations on discrete domains § Vector Space Model § Variations (Bag of Words Model) § Stop words (high frequency) § Document 1 § the, a , and The quick brown fox jumped over the lazy dog. § Stemming § Document 2 § jumps, jumped -> jump The brown dog jumped over the dog fence. § N-grams Doc 1 Doc 2 § quicK brown quick 1 0 § brown fox brown 1 1 § fox jump fox 1 0 § jump 1 1 Weighting § over 1 1 TF-IDF (term frequency- Document Matrix Document - inverse doc frequency) dog 1 2 Term fence 0 1 Salton, et al., 1975. A vector space model for automatic Manning and Schutze, Foundations of Statistical indexing. Communications of the ACM, 18(11), 613-620. Natural Language Processing. MIT Press. 1999. 7/18/18 ML Tutorial 8 ! Sequential Data ! § Natural Language Processing (NLP) Part of speech at np-tl nn-tl jj-tl nn-tl vbd nr at Word The Fulton County Grand Jury said Friday an !! !! W. Nelson Francis and Henry Kucera, 1979. The Brown Corpus: A Standard Corpus of Present-Day Edited American English. YG! MZOMC!@MH3;N*CMBJN3B* § Computer NetworkYG! MZOMC!@MH3;N*CMBJN3B* Traffic Analysis YGF! M[9#6&1#$%*B#%09* YGF! M[9#6&1#$%*B#%09* g)*!*9;)S.'8').!89383,!49!*9;)*797!HHC!A'79)!39]&9.;93!;).8#'.'.S!='A9!$'.73!)=!0&2#.!#;8').3W!4#<$!Z84)!$'.73[,! *&.!Z84)!$'.73[!#.7!3$'K!K9*=)*297!5%!GGg)*!*9;)S.'8').!89383,!49!*9;)*797!HHC!A'79)!39]&9.;93!;).8!&.'A9*3'8%!38&79.83!#8!#S93!)=!BREQQN!#'.'.S!='A9!$'.73!)=!0&2#.!#;8').3W!4#<$!Z84)!$'.73[,!?9!8))$!9#;0!#;8').!3;9.9!e!8'293!ZG!=)*! *&.!Z84)!$'.73[!#.7!3$'K!K9*=)*297!5%!GG7#8#5#39!#.7!B!=)*!*9;)S.'8').[N!!?9!&397!#!38#8';!;)<)*!!&.'A9*3'8%!38&79.83!#8!#S93!)=!BREQQN!PPM!A'79)!;#29*#!4'80!GC=K3!=*#29!*#89,!40';0!4#3!K)3'8').97!?9!8))$!9#;0!#;8').!3;9.9!e!8'293!ZG!=)*! 8)!#!J!2989*3!<).S!4#<$!4#%!8)!8#$9!=&<<!5)7%!2)A929.8!7#8#5#39!#.7!B!=)*!*9;)S.'8').[N!!?9!&397!#!38#8';!;)<)*!PPM!A'79)!;#29*#!4'80!GC=K3!=*#29!*#89,!40';0!4#3!K)3'8').97!)=!J!389K3N!V#;0!A'79)!=*#29!4#3!7)4.!3#2K<97!=*)2!IQC\eRC! K'\9<3!8)!GHCmQeC!K'\9<3N!"09!A'79)!=*#29!*#89!4#3!#<3)!7)4.E3#2K<97!8)!.)*2#<'a9!809!2)8').!3K997!).!9#;0!2)8').N!8)!#!J!2989*3!<).S!4#<$!4#%!8)!8#$9!=&<<!5)7%!2)A929.8!)=!J!389K3N!V#;0!A'79)!=*#29!4#3!7)4.!3#2K<97!=*)2!IQC\eRC! K'\9<3!8)!GHCmQeC!K'\9<3N!"09!A'79)!=*#29!*#89!4#3!#<3)!7)4.E3#2K<97!8)!.)*2#<'a9!809!2)8').!3K997!).!9#;0!2)8').N!!34546G >.!80'3!9\K9*'29.8,!49!79='.9!#!7#8#!398!)!40';0!8#$93!809!=)*2!)=!#!./ 34546 !89.3)*,!409*9!(kGG!Z809!.&259*!)=!K9)K<9[,! >.!80'3!9\K9*'29.8,!49!79='.9!#!7#8#!398!Wylie,!40';0!8#$93!809!=)*2!)=!#! et al., Using NoSQL Databases for Streaming! G !89.3)*,!409*9!(kGG!Z809!.&259*!)=!K9)K<9[,!Network Analysis, LDAV, 2012. VkJ!Z809!.&259*!)=!#;8').!;<#3393[,!#.7!)6kGC!Z809!.&259*!)=!'2#S9!=9#8&*93[N!./ VkJ!Z809!.&259*!)=!#;8').!;<#3393[,!#.7!§ Video Analysis 6kGC!Z809!.&259*!)=!'2#S9!=9#8&*93[N! ?#<$B! ?#<$Q! `&.B! `&.Q! :$'K! ?#<$B! ?#<$Q! `&.B! `&.Q! :$'K! Takayuki Hori, Jun Ohya and Jun Kurumisawa, Computational Imaging, 2010. 7/18/18 ML Tutorial 9 ?#<$! ?#<$! `&.! `&.! :$'K! ?'80!#*23!?#<$! ?'80)&8!#*23!?#<$! h)4!3K997!`&.! ('S0!3K997!`&.! :$'K! ?'80!#*23!4#A'.S! ?'80)&8!#*23!4#A'.S! h)4!3K997! ('S0!3K997! 4#A'.S! 4#A'.S! g'S&*9!IW!g'A9!1'.73!)=!(&2#.!T;8').3!#.7!V\K<#'.!)=!809!2)8').N! g'S&*9!IW!g'A9!1'.73!)=!(&2#.!T;8').3!#.7!V\K<#'.!)=!809!2)8').N! YGK! O#645$*!"#$%&'&8+%&5$*C#40-%4* YGK! O#645$*!"#$%&'&8+%&5$*C#40-%4* g)*!*9;)S.'8').!89383,!49!&397!BHJ!3#2K<93!ZGG!K9)K<9!+!J!#;8').3[N!"09!*9;)S.'8').!*93&<83!#*9!30)4.!'.!g'SN!R,!'.! 40';0!809!*9;)S.'8').!#;;&*#;'93!=)*!809!J!#;8').3!#*9!30)4.Ng)*!*9;)S.'8').!89383,!49!&397!BHJ!3#2K<93!ZGG!K9)K<9!+!J!!")!30)4!809!A#<'7'8%!)=!)&*!K*)K)397!2980)7,!)&*!2980)7!#;8').3[N!"09!*9;)S.'8').!*93&<83!#*9!30)4.!'.!g'SN!R,!'.! '3!;)2K#*97!4'80!D9#*938!D9'S05)*!*&<9!ZDD[!#.7!O*'.;'K#<!P)2K).9.8!T.#<%3'3!ZOPT[!5#397!2980)73N!"09!DDE5#397!40';0!809!*9;)S.'8').!#;;&*#;'93!=)*!809!J!#;8').3!#*9!30)4.N!")!30)4!809!A#<'7'8%!)=!)&*!K*)K)397!2980)7,!)&*!2980)7! '3!;)2K#*97!4'80!D9#*938!D9'S05)*!*&<9!ZDD[!#.7!O*'.;'K#<!P)2K).9.8!T.#<%3'3!ZOPT[!5#397!2980)73N!"09!DDE5#397! 2980)7!;#<;&<#893!809!7'==9*9.;9!598499.!809!'.K&8!7#8#!#.7!9#;0!7#8#!38)*97!'.!809!89.3)*#),!#.7!809!#;8').!80#8!S'A93!809! 2980)7!;#<;&<#893!809!7'==9*9.;9!598499.!809!'.K&8!7#8#!#.7!9#;0!7#8#!38)*97!'.!809!89.3)* ,!#.7!809!#;8').!80#8!S'A93!809! 2'.'2#<!7'==9*9.;9!'3!809!*93&<8!)=!809!*9;)S.'8').N!"09!OPTE5#397!*9;)S.'8').!2980)7!&393!V'S9.!A#<&93!)58#'.97!=*)2!#) 809!A#*'#.;9E;)A#*'#.;9!2#8*'\!'.!809!7#8#5#39,!#.7!39#*;093!2'.'2#<!7'==9*9.;9!'3!809!*93&<8!)=!809!*9;)S.'8').N!"09!OPTE5#397!*9;)S.'8').!2980)7!&393!V'S9.!A#<&93!)58#'.97!=*)2!=)*!809!#;8').!80#8!S'A93!809!2)38!2'.'2#<!7'==9*9.;9!)=!809! V'S9.!A#<&93N!!809!A#*'#.;9E;)A#*'#.;9!2#8*'\!'.!809!7#8#5#39,!#.7!39#*;093!=)*!809!#;8').!80#8!S'A93!809!2)38!2'.'2#<!7'==9*9.;9!)=!809! V'S9.!A#<&93N!! g'S&*9!RW!`9;)S.'8').!`93&<83!=)*!9#;0!#;8').N! ! g'S&*9!RW!`9;)S.'8').!`93&<83!=)*!9#;0!#;8').N! ! @:,AB,@CDQ+."*8+EFGG++EFGGHIBT @:,AB,@CDQ+."*8+EFGG++EFGGHIBT Downloaded from SPIE Digital Library on 30 May 2012 to 198.102.153.2. Terms of Use: http://spiedl.org/terms Downloaded from SPIE Digital Library on 30 May 2012 to 198.102.153.2. Terms of Use: http://spiedl.org/terms ! ! Major Types of Machine Learning § Unsupervised Learning § Supervised Learning § Semi-supervised Learning § Reinforcement Learning 7/18/18 ML Tutorial 10 Unsupervised Learning § Tasks § Clustering (grouping) § Dimensionality reduction § Anomaly detection § Association § Generative modeling § Experience (data) § Instances are unlabeled § Performance measures § Challenging due to lack of labels/known solutions § Validation often leverages labeled data sets (labels only used in testing) Fisher, 1936.
Details
-
File Typepdf
-
Upload Time-
-
Content LanguagesEnglish
-
Upload UserAnonymous/Not logged-in
-
File Pages46 Page
-
File Size-