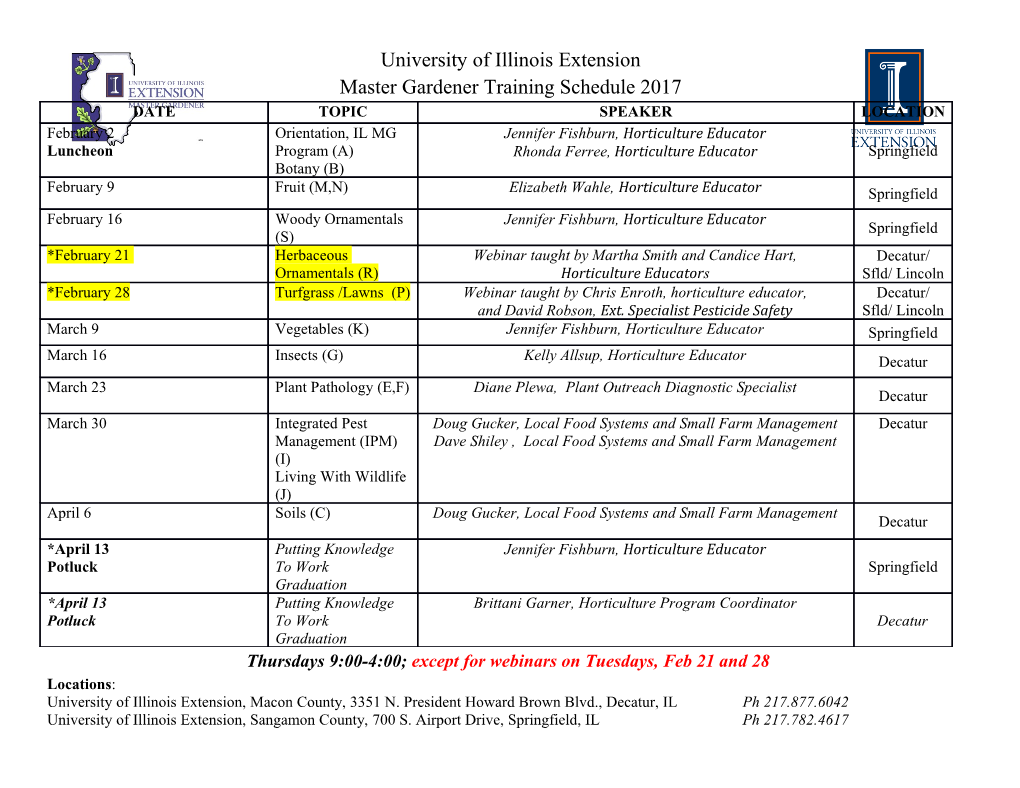
PERSPECTIVES Is there any particular situation where such complex structures emerge? The Criticality and scaling in answer is yes. In physics, fractal struc- tures in space and time were known to evolutionary ecology emerge in the proximity of some types of phase transition10,11. The classic example is a magnetic material. A small piece of Ricard V. Solé iron can tug on a paper clip at room tem- Susanna C. Manrubia perature, but if we heat it to a high tem- perature T, no magnetic power is ob- Michael Benton served. We say the magnetization M is Stuart Kauffman zero. The atoms that form the iron are themselves like small magnets. Each atom Per Bak only interacts with its nearest neighbors and their natural tendency is to align Fluctuations in ecological systems are known to involve a wide range of spatial and spontaneously into small domains with temporal scales, often displaying self-similar (fractal) properties. Recent theoretical the same orientation. At high T the cou- approaches are trying to shed light on the nature of these complex dynamics. The pling between nearest atoms breaks results suggest that complexity in ecology and evolution comes from the network-like down because of thermal perturbations structure of multispecies communities that are close to instability. If true, these ideas and, therefore, the atoms can have any might change our understanding of how complexity emerges in the biosphere and how polarity (up or down) and M 5 0. But sud- macroevolutionary events could be decoupled from microevolutionary ones. denly, when the material is cooled down, order spontaneously shows up. There is a critical temperature at which global Ricard Solé is at the CSRG-Dept of Physics, FEN, Universitat Politècnica de Catalunya, M. Campus Nord Mòdul B4, 08034 Barcelona, Spain ([email protected]; magnetization appears ( 0) and both Susanna Manrubia is at the Fritz-Haber Institut der Max-Planck Gesellschaft, Faradeyweg 4–6, fractal-spatial and fractal-temporal fea- 14195 Berlin, Germany ([email protected]); Michael Benton is at the tures arise. These transitions are de- Dept of Geology, University of Bristol, Bristol, UK BS8 1RJ ([email protected]); scribed by an ‘order parameter’ (here M), Stuart Kauffman is at the Santa Fe Institute, 1399 Hyde Park Road, Santa Fe, NM 87501, USA which is zero at the disordered phase ([email protected]); Per Bak is at the Bohr Institute, Biegdamsvej 17, DK-2100 Copenhagen, and positive otherwise. Denmark ([email protected]). Self-similarity is a defining character- istic of the critical state. The surprise came when physicists realized that very ractals are widespread in nature1,2 and t.0 is a given exponent, often called the different systems behaved exactly the Fhave features that look the same when critical exponent. The reason why these same when close to critical transition there is a change in scale: they are called laws are characteristic of fractal objects points6,10,11. Surprisingly, extremely sim- ‘self-similar’. In biology, self-similar pat- is that they are the only functions dis- ple models of these systems provided an terns are known to occur at many levels1–4 playing invariance under scale change. If exact description. This is a consequence (Box 1). But fractals are also present in we look at a larger or smaller scale – that of the so-called ‘universality’. Universal- time: the fluctuations of a given quantity is, if we take s9 5 gs – it is not difficult to ity means that systems sharing a small can appear the same when observed at see that N(s9) 5 C9N(s) or, in other words, number of basic features behave identi- different temporal resolutions (Fig. 1a). a change of scale does not modify the cally at the critical point. Are there also This is the case for heartbeat intervals5, basic statistical behavior. universal principles behind apparently epidemics in small islands6, breeding bird The same argument can be employed different biological phenomena? populations7 or the fossil record8. for a time series (Fig. 1b). If self-similar Because fractals involve long-range behavior is present, then the time corre- From RNA viruses to epidemics correlations, they also reflect some key lations should decay in a power-law fash- Starting at the molecular level, an features of how living systems are or- ion9. This is something that has been example of a critical point in biological ganized and how they evolve in time. The widely observed and is known as ‘1/f- systems can be exemplified by the dy- implications for evolution are very im- noise’6,8,9. A 1/f-like signal looks like a namics of RNA viruses12. The under- portant, because cooperative effects mountain landscape in time, rather than standing of how these entities evolve and emerging from the interactions can lead to space. The self-similarity is described by adapt must take into account their ex- new, sometimes counterintuitive, results. the power spectrum P(f ), which measures treme variability, caused by the error- A consequence of this is that order could the contribution of each frequency to the prone RNA polymerase activity and the be generated through evolution by a syn- overall time series6. The 1/f noise is defined lack of proofreading mechanisms13. In- ergy between natural selection and self- as P(f ) < f-b being the exponent 0,b,2. stead of a given single sequence, we have organizing processes. Scaling and power laws The common feature of self-similar be- Box 1. Fractals everywhere havior is the presence of scaling laws4 The patterns displayed by many natural systems do not allow for a simple description using Euclidean (also known as power laws). Given the fre- geometry: they present scale-invariance; that is, no characteristic length measure can be obtained from them. Therefore, when observed at different resolutions, they display the same pattern. This is the case quency distribution N(s) of some quantity of river networks and mountains1, tree branching and blood vessels3 or forest spatial structures2,4. Even s (number of species, size, lifetime, etc.) at the molecular level, fractals can be observed: if we analyse the linear distribution of nucleotides in a it is said that it follows a power law if DNA chain, a self-similar pattern can also be detected5. These structures – the so-called fractals – share N(s) 5 Cs2t (Fig. 1a). Let us assume that s the presence of long-range correlations. stands for size. Here C is a constant and 156 0169-5347/99/$ – see front matter © 1999 Elsevier Science. All rights reserved. PII: S0169-5347(98)01518-3 TREE vol. 14, no. 4 April 1999 PERSPECTIVES (a) Box 2. Recipe for a catastrophe: the error catastrophe 4 10 A population of strings is simulated to explore how the ‘master’ sequence population behaves when the mutation rate is changed (a). Here, N 5 100 strings of size n 5 15. Each string is defined by a sequence e S1S2…Sn where Si {0,1}. At each time step, we choose a string and make a copy of it after removing 103 5 5 another randomly chosen string. This replication takes place with probability P 1 if Si 1 for all units (this is the master sequence) and with probability P 5 0.05 for any other string16. The copy process involves a mutation rate m, which means that the probability of error per unit and per replication cycle is 102 m for each string. Starting from a random population, we repeat the same rules over 13104 steps and at Frequency the end we look into the system to see if there is any copy of the master sequence. Here the master sequence is the string 1111…11; that is, the one with the highest replication rate. This is averaged over 101 3 2 1 10 trials and for each mutation rate we compute how many of these replicas (Pm) have at least one master sequence. Although our intuition would expect a smooth, continuous decay of this probability, 100 101 102 there is actually a sharp decay close to a given critical mutation rate (i.e. Pm acts as an order parameter Avalanche size for this transition). This phenomenon is known as the ‘error catastrophe’. It describes the breakdown of heredity and the transition from a localized set of mutant sequences (localized around the master sequence in sequence space) towards a random set of strings17. The inset graphs show the evolution (b) of relative population sizes of strings when (b) m 5 0.05 and (c) m 5 0.12. The master sequence is indicated by the unbroken line in these two graphs. 30 (a) 20 1.0 10 Extinction rate (c) 0 40 0 200 400 600 0.8 Geological time (million years) 30 20 0.6 10 (c) Frequency (b) 60 0 0 100 200 0.4 40 Time Order parameter 20 Frequency 0.2 0 0 100 200 Time 0.0 0.00 0.10 0.20 Mutation rate (Online: Fig. I ) a cloud of mutants around the so-called theoretical19 work show that critical ‘master’ sequence. This cloud is known points could play a very important role in as a quasispecies, a term first coined by the evolution of quasispecies. Manfred Eigen in 1971 (Refs 14,15). These transitions are well known in Fig. 1. Scaling and self-similarity. (a) Power RNA viruses adapt to a changing the dynamics of infectious diseases20 and law: in a log–log plot, a power law distribution, 21 defined as N(s) < s2a gives a straight line. environment by making use of their in some habitat fragmentation models .
Details
-
File Typepdf
-
Upload Time-
-
Content LanguagesEnglish
-
Upload UserAnonymous/Not logged-in
-
File Pages5 Page
-
File Size-