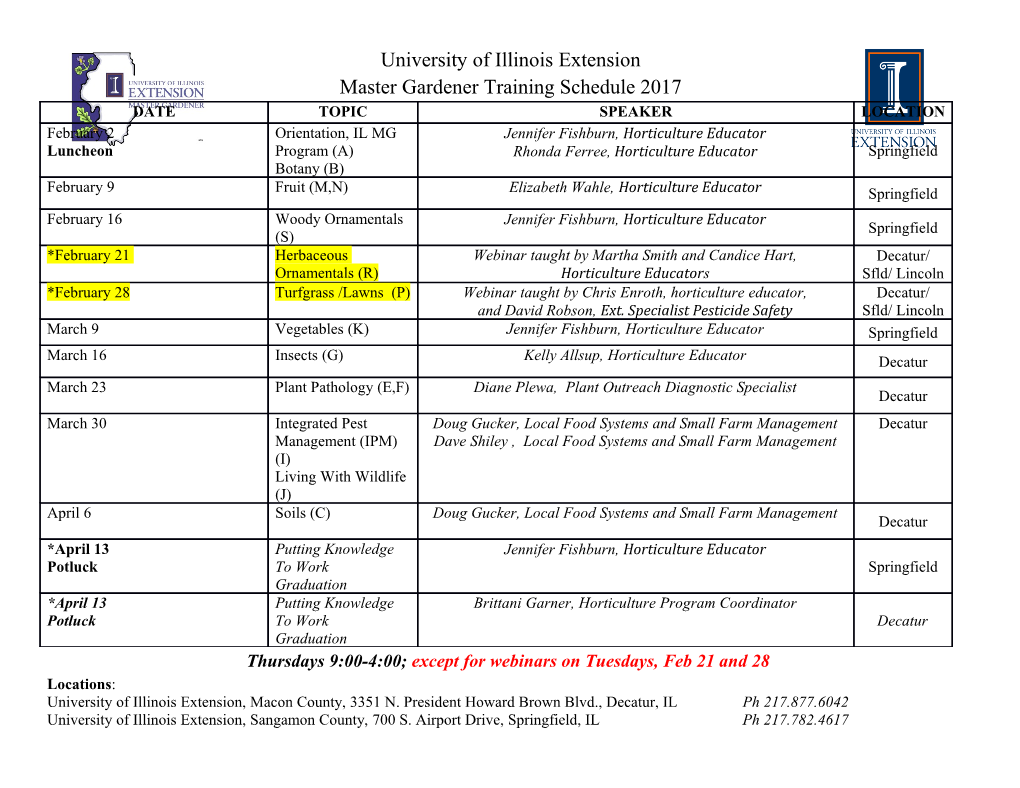
Supporting Long-Running Applications in Shared Compute Clusters Panagiotis Garefalakis Department of Computing Imperial College London This dissertation is submitted for the degree of Doctor of Philosophy June 2020 Abstract Clusters that handle data-intensive workloads at a data-centre scale have become commonplace. In this setting, clusters are typically shared across several users and applications, and consolidate workloads that range from traditional analytics applications to critical services, stream processing, machine learning, and complex data processing applications. This constitutes a growing class of applications called long-running, consisting of containers that are used for durations ranging from hours to months. Even though long-running applications occupy a significant amount of resources in shared compute clusters today, there is currently rudimentary systems support that not only hinders application performance and resilience but also decreases cluster resource efficiency i.e., the effective utility extracted from cluster resources. In this thesis, we describe two main areas that lack support for long-running applications in traditional system designs. First, the way modern data processing frameworks execute complex computation tasks as part of shared long-running containers is broken. Even though these frameworks enable users to combine different types of computation as part of the same application using high-level programming interfaces, they ignore their diverse latency and throughput requirements during execution. Second, existing systems that allocate resources for long-running applications in the form of containers lack an expressive interface that can capture their placement requirements in shared compute clusters. Such placements can be expressed by means of complex constraints and are critical for both the performance and resilience of long-running applications. To target the aforementioned mismatch, we introduce our contribution of unified dataflows with placement constraints, an abstraction that enables the efficient execution and the effective placement of long-running applications in shared compute clusters. Our abstraction is realised as part of a novel execution framework and a new cluster manager following a two-scheduler design. We first present NEPTUNE, a data processing framework that captures application execution require- ments and employs coroutines as a lightweight mechanism for suspending and resuming tasks within long-running containers with sub-millisecond latency. NEPTUNE introduces scheduling policies that can dynamically prioritise tasks of heterogeneous jobs respecting their requirements in an efficient manner while achieving high resource utilisation. iv We then introduce MEDEA, a cluster scheduler that enables the effective placement of containers in shared compute clusters. It follows an optimisation-based scheduling approach that is used for applications with sustained impact on cluster resources. MEDEA introduces powerful placement constraints with formal semantics that developers can use to capture container interactions within and across applications. MEDEA has been deployed in large production clusters and has been open-sourced as part of Apache Hadoop 3.1 release. Acknowledgements Foremost, I want to express my sincere gratitude to my supervisor Peter Pietzuch for his continuous support over the past five years. Peter’s passion for research, inspiring counsel, and high standards have been crucial in shaping the core of this thesis. I want to specially thank Konstantinos Karanasos, my industrial mentor, who guided and helped me in countless ways. Our research and non-research conversations challenged and motivated me while ultimately helped me grow both as a person and as a researcher. I am also grateful to my examiners, Seif Haridi and Giuliano Casale, for their insightful comments and suggestions that helped me improve this document after a lively discussion. Next, I would like to thank Imperial College London and the High-Performance and Embedded Distributed Systems (HiPEDS) Centre for Doctoral Training (CDT) programme for supporting my studies. I also want to thank the fantastic members of Large-Scale Distributed Systems (LSDS) group at Imperial with whom I shared office all these years. Special thanks to Alexandros Koliousis, George Theodorakis, Jana Giceva, Holger Pirk, Christian Priebe, Joshua Lind, and Raul Castro Fernandez for all work done together, the technical discussions, and the off-topic conversations that made the past years such a pleasant experience. The ideas for both the systems presented in this dissertation are heavily influenced by my internships at Microsoft back in 2016 and 2017 where I worked with the CISL group. Konstantinos Karanasos, Arun Suresh, Sriram Rao, Virajith Jalaparti, Avrilia Floratou, Ashvin Agrawal, Carlo Curino, Subru Krishnan all helped me with their invaluable advice and their technical expertise. Working with distinguished researchers from the industry helped me realise several opportunities for innovative systems targeting large-scale shared compute clusters. Thanks to Ioanna, Christos, Salvatore, and the rest of my wonderful friends around the world for being by my side in the good days and the bad days. I will always be grateful to Nayia for making everything easier. Even though we met halfway through this journey, it wouldn’t be the same without her love and support. Finally and above all, I am profoundly grateful to my family who supported me through this difficult journey. I thank my wonderful parents Georgia and Giannis, and my sister Eirini for their endless love, unconditional support, and for their encouragement to pursue my dreams. Declaration This thesis presents my work in the Department of Computing at Imperial College London for the period between October 2015 and October 2019. Parts of the work described were done in collaboration with other researchers: • Chapter 4: I proposed, implemented and evaluated the design of NEPTUNE execution frame- work. The metric collection and windowing mechanism was contributed to NEPTUNE by Christo Lolov as part of his MEng project at Imperial College London during the academic year 2018-2019. • Chapter 5: I also led the development and evaluation of MEDEA cluster scheduler based on several production use-cases and requirements. The design of the system was result of many fruitful discussions with Arun Suresh, Konstantinos Karanasos and the open-source community as part of my collaboration with Microsoft’s Cloud and Information Services Lab where I interned for the summers of 2016 and 2017. I declare that the work presented in this thesis is my own, except where declared above. Panagiotis Garefalakis June 2020 viii © The copyright of this thesis rests with the author. Unless otherwise indicated, its contents are licensed under a Creative Commons Attribution 4.0 International Licence (CC BY). Under this licence, you may copy and redistribute the material in any medium or format for both commercial and non-commercial purposes. You may also create and distribute modified versions of the work. This on the condition that you credit the author. When reusing or sharing this work, ensure you make the licence terms clear to others by naming the licence and linking to the licence text. Where a work has been adapted, you should indicate that the work has been changed and describe those changes. Please seek permission from the copyright holder for uses of this work that are not included in this licence or permitted under UK Copyright Law. Table of contents 1 Introduction1 1.1 The evolution of Big Data processing . .2 1.2 Research motivation . .4 1.3 Contributions . .5 1.3.1 Unified dataflows . .5 1.3.2 Placement constraints . .6 1.4 Related publications . .6 1.5 Outline . .7 2 Background9 2.1 Cluster workloads . 10 2.1.1 Workload types . 12 2.1.2 Workload scheduling . 13 2.2 Data processing systems . 15 2.2.1 Dataflow model . 17 2.2.2 Programming interface . 18 2.2.3 Execution model . 21 2.2.4 Evolution of the execution model . 22 2.2.5 Scheduling hybrid dataflow applications . 24 2.2.6 Data processing summary . 27 2.3 Cluster scheduling . 27 x Table of contents 2.3.1 Long-running application performance . 28 2.3.2 Long-running application resilience . 31 2.3.3 Global cluster objectives . 33 2.3.4 Cluster scheduling requirements . 33 2.3.5 Cluster scheduler architectures . 36 2.3.6 Cluster scheduling summary . 40 2.4 Chapter summary . 40 3 Unified Dataflows with Placement Constraints 41 3.1 Overview . 42 3.2 Unified dataflows . 43 3.2.1 Execution model . 44 3.2.2 API . 45 3.3 Placement constraints . 46 3.3.1 Expressing constraints . 47 3.3.2 Container tags . 48 3.3.3 Node groups . 49 3.4 Discussion . 49 3.5 Summary . 50 4 Neptune: An Execution Framework for Suspendable Tasks 53 4.1 Overview . 55 4.2 Unified dataflow applications . 56 4.2.1 Expressing stream/batch applications . 56 4.2.2 Scheduling stream/batch applications . 57 4.2.3 Executing stream/batch tasks . 58 4.3 Suspendable tasks . 59 4.4 Locality- and memory-driven scheduling . 62 Table of contents xi 4.4.1 Metric windowing . 63 4.4.2 Scheduling policy . 64 4.5 Implementation . 66 4.6 Evaluation . 69 4.6.1 Experimental set-up . 70 4.6.2 Application performance . 72 4.6.3 Varying resource demands . 76 4.6.4 Task suspension . 79 4.7 Limitations and discussion . 81 4.8 Summary . 83 5 Medea: A Cluster Scheduler with Rich Placement Constraints 85 5.1 Overview . 87 5.2 Expressing placement constraints . 89 5.2.1 Discussion . 92 5.3 Scheduling long-running applications . 92 5.3.1 Overview . 92 5.3.2 ILP-based scheduling . 93 5.3.3 Heuristic-based scheduling . 95 5.4 Implementation . 96 5.5 Evaluation . 99 5.5.1 Experimental set-up . 99 5.5.2 Application performance . 102 5.5.3 Application resilience . 104 5.5.4 Global cluster objectives . 105 5.5.5 Scheduling latency . 108 5.6 Limitations and discussion . 111 5.7 Summary . 112 xii Table of contents 6 Conclusions and future work 115 6.1 Thesis summary .
Details
-
File Typepdf
-
Upload Time-
-
Content LanguagesEnglish
-
Upload UserAnonymous/Not logged-in
-
File Pages148 Page
-
File Size-