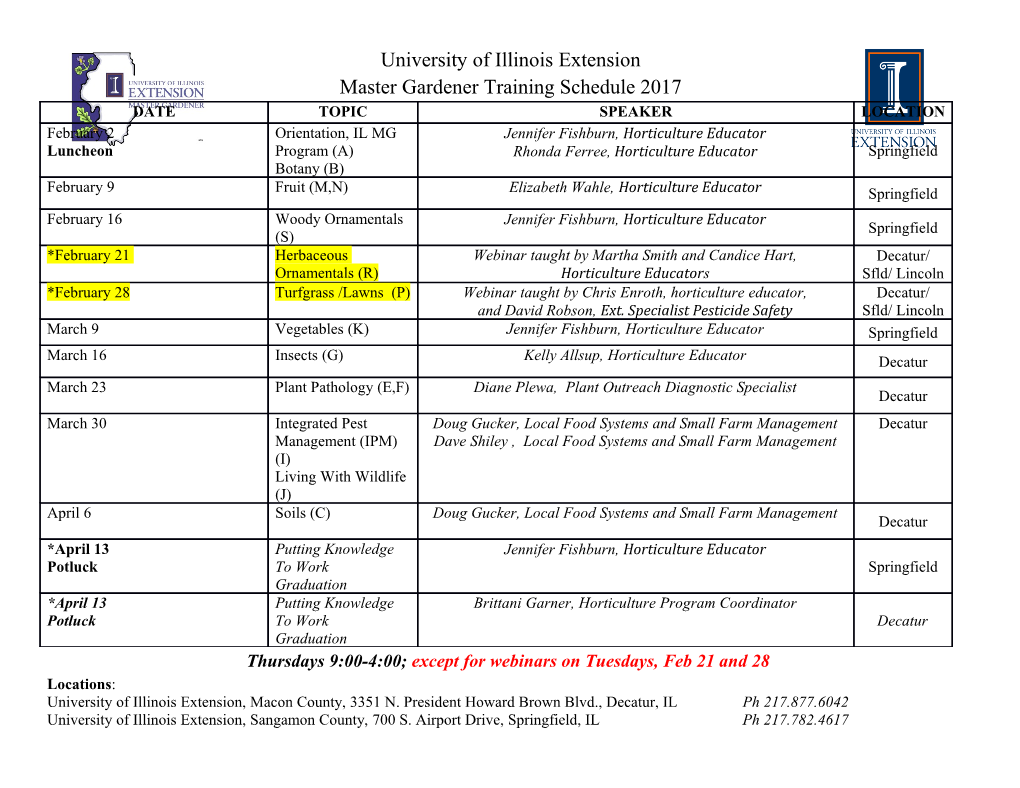
Univariate Analysis Statistics & Quantitative Analysis n Assumptions of Regression Model SIPA U4320 n Regression Line n Population Parameters n The standard regression equation is Segment 10: Multiple Regression Yi= a + bXi + ei n The only things that we observe is Y and X. Prof. Sharyn O’Halloran n From these data we estimate a and b. n But our estimate will always contain some error. Key Points Univariate Analysis (cont.) n This error is represented by: n Review Univariate Regression Model e i = Yi -Y n Introduce Multivariate Regression Y = a + bX n Assumptions i X2 X1 X e2 X n Estimation X X e e3 n 1 X Hypothesis Testing X X3 Yield X X n Interpreting Multiple Regression Model X X X X n “Impact of X on Y controlling for ....” a X X b intercept X X =0 n Slope Coefficient as a Multiplication Factor X X n Path Diagram and Causal Models n Direct and Indirect Effects Fertilizer Copy Right Sharyn O'Halloran 2001 1 Univariate Analysis (cont.) Univariate Analysis (cont.) n Underlying Assumptions n Sample Parameters n Linearity n Most times we don’t observe the underlying n The true relation between Y and X is captured in the equation: population parameters. Y = a + bX n All we observe is a sample of X and Y values n Homoscedasticity (Homogeneous Variance) from which make estimates of a and b. n Each of the ei has the same variance. n 2 2 The predicted line takes the form: $ E(ei )= s for all i Y = a+bX where: å xy n Independence b= a =Y -bX 2 Relation Between Yield and Fertilizer n Each of the ei's is independent from each other. That is, the å x value of one does not effect the value of any other 100 predicted line observation i's error. The predicted line is the expected 80 Cov(e ,e ) = 0 for i ¹ j value of Y for a given value of X. 60 i j 40 n Normality 20 2 For any value of the dependent Yield (Bushel/Acre) 0 n Each ei is normally distributed with mean=0 and variance s 2 variable, there is a single most likely 0 100 200 300 400 500 600 700 800 ei ~ N(0, s ) value for the independent variable. Fertilizer (lb/Acre) Univariate Analysis (cont.) Univariate Analysis (cont.) Probability of Y given X n So we introduce a new form of error in our analysis. P(Y/X) Estimated regression line $ ei = Yi - Y Yˆ = a + bX Source of error: Y=a+bX 2 Inherent variability X2 Yield s2 s e of sampling process X1 X 2 X X e e3 Y2 Y = a + bX 1 X X X3 s2 Yield X Y3 True regression line e 2 e 3 e 1 X Y1 X X a b=0 intercept X X X X1 X3 X X2 Fertilizer X Fertilizer Copy Right Sharyn O'Halloran 2001 2 Univariate Analysis (cont.) Univariate Analysis (cont.) n Inferences n Standard Error n Make inferences about the population given a n The standard error is exactly by how much sample. our estimate of b is off. Where, x2 = (X -X )2 n Best Fit Line s i Standard error of b = n We are estimating the population line by drawing the 2 N Sx (X - X )2 best fit line through our data, å i s = i =1 Y$ = a + bX N n Rewrite the Formula: Spread n We estimate both a slope and an intercept. s s s 1 of X Standard Error = = · 2 2 å xy n 2 æ Sx ö n å x b = × Sx nç ÷ 2 a = Y - bX n è n ø n Standard å x Error Univariate Analysis (cont.) Univariate Analysis (cont.) n The Standard Error of slope b § Distribution of error terms n Parameter of interest is b s n Slope coefficient b measures the impact of one SE = Sx 2 variable on the dependent variable. n When b=0 implies X has no effect on Y E(b) =b n To construct a statistical test of the slope of the regression line, we need to know its mean and n This makes sense, b is the factor that standard error. relates the X’s to the Y, n Mean n The standard error depends on both the n The mean of the slope of the regression line expected variations in the Y’s and on the Expected value of b = b. variation in the X’s. Copy Right Sharyn O'Halloran 2001 3 Univariate Analysis (cont.) Univariate Analysis (cont.) n Hypothesis Testing n Example: Do people save more n 95% Confidence Intervals (s unknown) money as their income increases? n Confidence interval for the true slope of b given our estimate b: n Data: Suppose we observed 4 individual's income and saving rates? b = b± t.025 SE Income Savings X-deviation Y-deviation xy x2 Predicted-Y Deviation from Squared Deviation s Observation (X) (Y) from mean from mean Predicted Y from Predicted Y b = b ± t.025 2 1 22 2 1 -0.2 -0 1 2.34 -0.34 0.116 Sx 2 18 2 -3 -0.2 0.6 9 1.77 0.23 0.053 3 17 1.6 -4 -0.6 2.4 16 1.63 -0.03 0.0009 4 27 3.2 6 1 6 36 3.05 0.15 0.0225 n Test to see if the hypothesis lies within the Sum 84 8.8 0 0 8.8 62 8.79 0.1924 estimated range. Mean 21 2.2 ˆ x = (X i - X ) y = (Yi -Y ) Predicted Line Y = a + bX Univariate Analysis (cont.) Univariate Analysis (cont.) n P-values n Calculate the fitted line n P-value is the probability of observing an Y= a + bX event, given that the null hypothesis is true. n Estimate b b = Sxy / Sx2 = 8.8 / 62 = 0.142 n We can calculate the p-value by: n What does this mean? n Standardizing and calculating the t-statistic: b - b n On average, people save a little over 14% of every t = 0 extra dollar they earn. SE n Intercept a n Determine the Degrees of Freedom: n a = Y — b X = 2.2 - 0.142 (21) = -0.782 For univariate analysis = n-2 n What does this mean? n Find the probability associated with the t- n With no income, people borrow statistics with n-2 degrees of freedom in the t- table. n Regression equation is: Yˆ = - 0.78 + 0.142X Copy Right Sharyn O'Halloran 2001 4 . Univariate Analysis(cont.) Univariate Analysis (cont.) n What is the formula for the confidence interval? Savings Ratio by Income s .309 b = b ± t . b = .142 ± 4.30 · . 4 .025 å x2 62 3 Yˆ = - 0.78 + 0.142X 2 b = .142 ± .169 Þ -.027 £ b £ .311 1 n Reject or fail to reject the null hypothesis Savings 0 -.078 -1 0 5 10 15 20 25 30 n Since zero falls within this interval, we cannot reject the null hypothesis. -2 Income This is probably due to the small sample size Ø Each additional unit of income you save 14.2 cents Ø People with no income borrow. -.027 b=0 .311 Univariate Analysis (cont.) Univariate Analysis (cont.) n Calculate a 95% confidence interval n Additional Examples n State Hypothesis n How about the hypothesis that b = .50, so that n Now let's test the null hypothesis that b = 0. people save half their extra income? n That is, the hypothesis that people do not save any of the extra money they earn. n It is outside the confidence interval, so we can reject this hypothesis. H0: b = 0 Ha: b ¹ 0; n Let's say that it is well known that Japanese at the 5% significance level. consumers save 20% of their income on average. n Construct the Confidence Interval n Can we use these data (presumably from American families) n What do we need to calculate the confidence interval? to test the hypothesis that Japanese save at a higher rate 2 than Americans? n Degrees of Freedom 2 (Yi - Y) .192 s = = = 0.096 n Since 20% also falls within the confidence interval, we cannot n a-level = .05 n - 2 2 reject the null hypothesis that Americans save at the same rate n Sample variance s = 0.096 = .309 as Japanese. Copy Right Sharyn O'Halloran 2001 5 Regression in Excel Regression in Excel(cont.) Relation between Powerboat Registrtion (1000) n Example: and Manatee Deaths Graph Data: 60 n Manatees are large gentle sea creatures that live 50 along the Florida coast. 40 -35.18 + 0.11X 1 ˆ 30 Y = * * Manatees Killed n Many Manatees are killed or injured by (-4.57 ) (8.93) 20 powerboats each year. 10 0 Registration -100 0 100 200 300 400 500 600 700 800 n The US Fish and Wildlife Service conducted a -10 study on the impact on registration permits and For each additional -20 -30 number of Manatees killed. 1000 powerboats -40 registered, we expect Manatee Data an increase of .11 *Note: t-statistics in parentheses. * indicates p-value <0.05 Number of Manatee Manatee Deaths. Coefficients Standard Error t Stat P-value Powerboats Deaths Intercept -35.18 7.70 -4.57 0.000314 Powerboat registration (1000) 0.11 0.01 8.93 0.000000 Regression in Excel Regression in Excel(cont.) These are the data collected: n Hypothesis Testing Powerboat Manatees Powerboat Manatees H0: b1 = 0 Year registration (1000) Killed registration (1000) Killed 1977 447 13 1978 460 21 Descriptive Statistics Ha: b1 ¹ 0 1979 481 24 Mean 601.56 Mean 32.61 1980 498 16 Standard Error 24.46 Standard Error 3.02 n Calculate a 95% Confidence Interval 1981 513 24 Median 599.50 Median 33.50 1982 512 20 1983 526 15 Mode 716.00 Mode 24.00 n-1-k 1984 559 34 Standard Deviation 103.79 Standard Deviation 12.82 b ± t * SE 1985 585 33 Sample Variance 10773.32 Sample Variance 164.25 .025 b 1986 614 33 Range 288.00 Range 40.00 a=.025 a=.025 1987 645 39 1988 675 43 Minimum 447.00 Minimum 13.00 0.11± 2.12*0.01 1989 711 50 Maximum 735.00 Maximum 53.00 1990 719 47 Sum 10828.00 Sum 587.00 0.11212 0.10788 1991 716 53 Count 18.00 Count 18.00 0.11± 0.00212 1992 716 38 Confidence Confidence 1993 716 35 1994 735 49 Level(95.0%) 51.62 Level(95.0%) 6.37 n Reject or Fail to Reject Null Hypothesis Ø Does the number of Registered Powerboats increase n Therefore, we reject the null hypothesis that b1=0 in the number of Manatees killed? favor of the alternative that it is not equal to 0.
Details
-
File Typepdf
-
Upload Time-
-
Content LanguagesEnglish
-
Upload UserAnonymous/Not logged-in
-
File Pages25 Page
-
File Size-