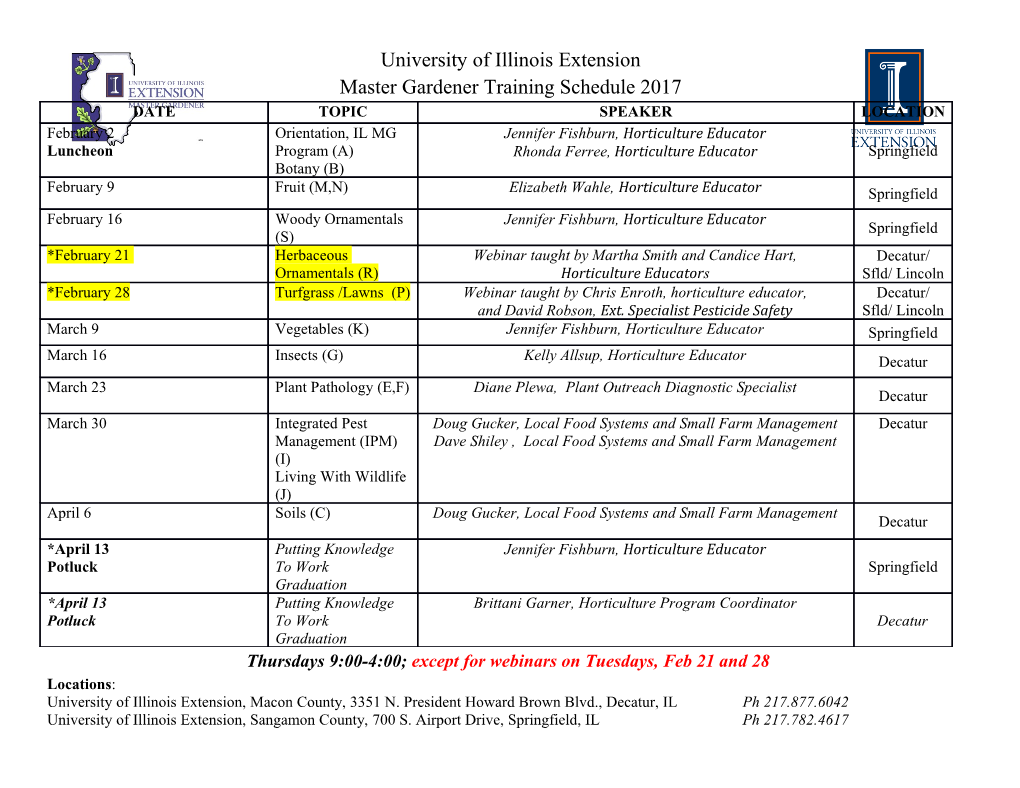
1 Two-Moment Inequalities for Renyi´ Entropy and Mutual Information Galen Reeves Abstract—This paper explores some applications of a two- and chi-square divergence. One application of these results moment inequality for the integral of the r-th power of a function, is to provide bounds for mutual information in terms of 0 <r< 1 where . The first contribution is an upper bound on the divergence measures that dominate relative entropy, such as Renyi´ entropy of a random vector in terms of the two different moments. When one of the moments is the zeroth moment, these the chi-square divergence; see e.g. [13], [15]. bounds recover previous results based on maximum entropy distributions under a single moment constraint. More generally, A. Overview of results evaluation of the bound with two carefully chosen nonzero moments can lead to significant improvements with a modest The starting point of our analysis (Proposition 2) is an increase in complexity. The second contribution is a method for inequality for the integral of the r-th power of a function. upper bounding mutual information in terms of certain integrals Specifically, for any numbers p,q,r with with respect to the variance of the conditional density. The bounds 1 r have a number of useful properties arising from the connection 0 <r< 1 and p< − < q, with variance decompositions. r Index Terms—Information Inequalities, Mutual Information, the following inequality holds: Renyi´ Entropy. 1 r λ 1−λ f r(x) dx C x pf(x) dx x qf(x) dx , ≤ | | | | I. INTRODUCTION Z Z Z for all non-negative functions f : R+ R+ where C and Measures of entropy and information play a central role 0 <λ< 1 are given explicitly in terms→ of the tuple (p,q,r). in applications throughout information theory, statistics, com- An extension to functions defined on an arbitrary subset of Rn puter science, and statistical physics. In many cases, there is is also provided (Proposition 3). interest in understanding maximal properties of these measures The remainder of the paper shows how this inequality can over a given family of distributions. One example is given be used to provide bounds on information measures such as by the principle of maximum entropy, which originated in R´enyi entropy and mutual information. Some useful properties statistical mechanics and was introduced in broader context of the bounds include: by Jaynes [1]. • Simplicity: Beyond the existence of a density, these Entropy-moment inequalities can be used to describe prop- bounds do not require further regularity conditions such erties of distributions characterized by moment constraints. as boundedness or sub-exponential tails. As a conse- Perhaps the most well known entropy-moment inequality quence, these bounds can be applied under relatively mild follows from the fact that the Gaussian distribution maximizes technical assumptions. differential entropy over all distributions with the same vari- • Tightness: For some applications, the bounds can provide ance [2, Theorem 8.6.5]. This inequality leads to remarkably an accurate characterization of the underlying information arXiv:1702.07302v1 [cs.IT] 23 Feb 2017 simple proofs for fundamental results in information theory measures. For example, a special case of Proposition 9 in and estimation theory. this paper played a key role in the author’s recent work A variety of entropy-moment inequalities have also been [16]–[18], where it was used to bound the relative entropy studied in the context of R´enyi entropy [3]–[7], which is a between low-dimensional projections of a random vector generalization of Shannon entropy. Recent work has focused and a Gaussian approximation. on the extremal distributions for the closely related R´enyi • Geometric Interpretation: Our bounds on the mutual divergence [8]–[12]. information between random variables X and Y can be Another line of work focuses on relationships between expressed in terms of the variance of the conditional measures of dissimilarity between probability distributions density of Y given X. Specifically, the bounds depend provided by the family of f-divergences [13], [14], which on integrals of the form: includes as special cases, the total variation distance, relative entropy (or Kullback-Leibler divergence), R´enyi divergence, y s Var(f (y X)) dy. k k Y |X | Z The work of G. Reeves was supported in part by funding from the For , this integral is the expected squared 2 Laboratory for Analytic Sciences (LAS). Any opinions, findings, conclusions, s = 0 L and recommendations expressed in this material are those of the author and distance between the conditional density fY |X and the do not necessarily reflect the views of the sponsors. marginal density fY . G. Reeves is with the Department of Electrical and Computer Engineering and the Department of Statistical Science, Duke University, Durham (e-mail: The paper is organized as follows: Section II provides [email protected]) integral inequalities for nonnegative functions; Section III 2 gives bounds on R´enyi entropy of orders less than one; and a different and very simple proof that depends only on H¨older’s Section IV provides bounds on mutual information. inequality. Proposition 1. Let f be a nonnegative Lebesgue measurable II. MOMENT INEQUALITIES function defined on the positive reals R+. For any number Rk Rk Throughout this section, we assume that f is a real-valued 0 <r< 1 and vectors s and ν +, we have Lebesgue measurable function defined on a measurable subset ∈ ∈ k of Rn. For any positive number , the function is S p p f c (ν,s) ν µ (f). defined according to k·k k kr ≤ r i si i=1 1 X p k p Proof. Let si . Then, we have f = f(x) dx . g(x)= i=1 νi x k kp | | S r −r r Z f r =P g (fg) 1 Recall that for 0 <p< 1, the function p is not a norm k k k −r k r k·k g 1 (gf) 1 because it does not satisfy the triangle inequality. The s-th 1−r r ≤k − k k k r 1−r moment of f is defined according to = g 1−r gf r k k1 k k1 k r µ (f)= x s f(x) dx, s = cr(ν,s) νi µs (f) , S k k i Z i=1 where denotes the standard Euclidean norm on vectors. X k·k where second step follows from H¨older’s inequality with conjugate exponents 1/(1 r) and 1/r. A. Multiple Moments − Consider the following optimization problem: B. Two Moments maximize f The next result follows from Proposition 1 for the case of k kr subject to f(x) 0 for all x S two moments. ≥ ∈ µ (f) m for 1 i k. Proposition 2. Let f be a nonnegative Lebesgue measureable si ≤ i ≤ ≤ function defined on the positive reals R+. For any numbers For r (0, 1) this is a convex optimization problem because with and , we have r ∈ p,q,r 0 <r< 1 p< 1/r 1 < q r is concave and the moment constraints are linear. By − k·k 1−r r λ 1−λ standard theory in convex optimization (see e.g., [19]), it can f r [ψr(p, q)] [µp(f)] [µq(f)] , be shown that if the problem is feasible and the maximum is k k ≤ where λ = (q +1 1/r)/(q p) and finite, then the maximizer has the form − − 1 k r−1 1 rλ r(1 λ) ∗ ∗ si ψr(p, q)= B , − , (1) f (x)= νi x , for all x S. (q p) 1 r 1 r k k ∈ − − − i=1 X a+b −a −b ∗ ∗ where B(a,b)=B(a,b)(a +eb) a b and B(a,b) is the The parameters ν1 , ,νk are nonnegative and the i-th mo- ··· ∗ Beta function. ment constraint holds with equality for all i such that νi ∗ ∗ e 1−λ −λ is strictly positive, that is νi > 0 = µsi (f ) = mi. Proof. Letting s = (p, q) and ν = (γ ,γ ) with λ > 0, Consequently, the maximum can be expressed⇒ in terms of a we have linear combination of the moments: ∞ r r − − 1−r 1−λ p −λ q 1 r k [cr(ν,s)] = γ x + γ x dx. 0 f ∗ r = (f ∗)r = f ∗(f ∗)r−1 = ν∗m . Z r 1 1 i i 1 k k k k k k − i=1 Making the change of variable x (γu) q p leads to X 7→ ∞ b−1 For the purposes of this paper, is it is useful to consider r 1 u B(a,b) 1−r a relative inequality in terms of the moments of the function [cr(ν,s)] = a+b du = , (q p) 0 (1 + u) (q p) itself. Given a number 0 <r< 1 and vectors s Rk and − Z − Rk ∈ where a = r λ and b = r (1 λ) and the second step ν + the function cr(ν,s) is defined according to 1−r 1−r ∈ follows from the integral representation− of the Beta function 1−r r r ∞ k − 1−r [20, Eq. (1.1.19)]. Therefore, by Proposition 1, the inequality si cr(ν,s)= νi x dx , 1−r r Z0 i=1 ! B(a,b) 1−λ −λ X f r γ µp(f)+ γ µq(f) , k k ≤ q p if the integral exists. Otherwise, cr(ν,s) is defined to be pos- − itive infinity. It can be verified that cr(ν,s) is finite provided holds for all γ > 0. Evaluating this inequality with that there exists i, j such that ν and ν are strictly positive i j λµ (f) and s < (1 r)/r <s .
Details
-
File Typepdf
-
Upload Time-
-
Content LanguagesEnglish
-
Upload UserAnonymous/Not logged-in
-
File Pages12 Page
-
File Size-