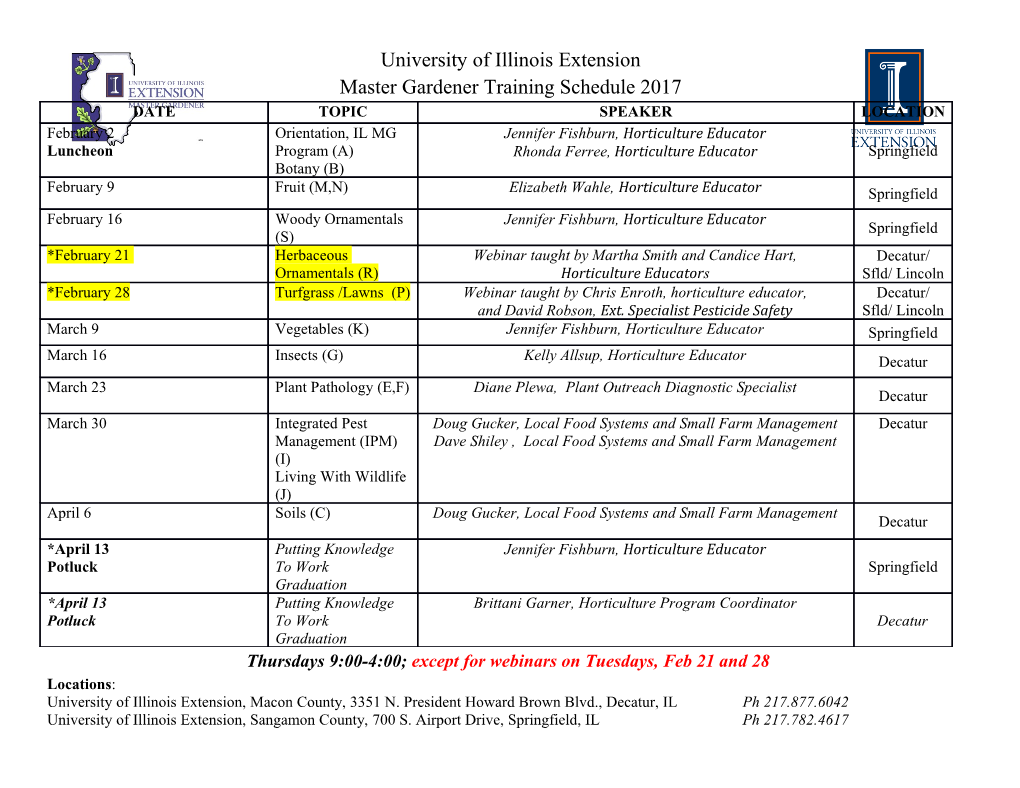
Experimental and Conceptual Approaches to Studying Bet Hedging in Microorganisms by Colin Scott Maxwell Department of Biology Duke University Date: Approved: Paul M. Magwene, Co-Supervisor L. Ryan Baugh, Co-Supervisor Nicolas E. Buchler Katharina V. Koelle Daniel J. Lew Dissertation submitted in partial fulfillment of the requirements for the degree of Doctor of Philosophy in the Department of Biology in the Graduate School of Duke University 2016 Abstract Experimental and Conceptual Approaches to Studying Bet Hedging in Microorganisms by Colin Scott Maxwell Department of Biology Duke University Date: Approved: Paul M. Magwene, Co-Supervisor L. Ryan Baugh, Co-Supervisor Nicolas E. Buchler Katharina V. Koelle Daniel J. Lew An abstract of a dissertation submitted in partial fulfillment of the requirements for the degree of Doctor of Philosophy in the Department of Biology in the Graduate School of Duke University 2016 Copyright c 2016 by Colin Scott Maxwell All rights reserved except the rights granted by the Creative Commons Attribution-Noncommercial Licence Abstract Bet-hedging strategies are used by organisms to survive in unpredictable environ- ments. To pursue a bet-hedging strategy, an organism must produce multiple phe- notypes from a single genotype. What molecular mechanisms allow this to happen? To address this question, I created a synthetic system that displays bet-hedging be- havior, and developed a new technique called `TrackScar' to measure the fitness and stress-resistance of individual cells. I found that bet-hedging can be generated by actively sensing the environment, and that bet-hedging strategies based on active sensing need not be metabolically costly. These results suggest that to understand how bet-hedging strategies are produced, microorganisms must be examined in the actual environments that they come from. iv Contents Abstract iv List of Tables ix List of Figuresx List of Abbreviations and Symbols xii Acknowledgements xiii 1 Introduction1 2 Bet Hedging by Active Sensing Need not be Costly4 2.1 Introduction................................4 2.2 Results...................................7 2.2.1 URA3 (counter-)selection mimics a classic model of bet-hedging7 2.2.2 Plasticity to estradiol can generate a phenotypic trade-off...9 2.2.3 Sensing an uninformative cue enables a bet-hedging strategy. 10 2.2.4 Bet hedging by plasticity need not carry a cost......... 14 2.2.5 Bet hedging by sensing an uniformative cue can be tuned by mutation.............................. 16 2.3 Discussion................................. 18 2.3.1 Bet hedging by active sensing is plausible............ 19 2.3.2 Bet hedging by active sensing is evolvable........... 20 2.3.3 Bet hedging strategies based on phenotypic plasticity need not be costly.............................. 21 v 2.3.4 Sensing uninformative cues can serve as the basis of bet-hedging strategies............................. 22 2.4 Methods.................................. 24 2.4.1 Strains and media......................... 24 2.4.2 Plasmid construction....................... 24 2.4.3 Strain construction........................ 26 2.4.4 Flow cytometry and OD measurements............. 28 2.4.5 Fitness and competition experiments in liquid culture..... 28 2.4.6 Competiton experiments on agar plates............. 28 2.4.7 Patterns of growth, image processing, and statistics...... 29 2.5 Strains, primers, and plasmids used in this study........... 30 3 Known Unknowns: Sensing Uninformative Cues can Generate Bet- hedging Strategies 34 3.1 Introduction................................ 34 3.2 Bet hedging in microbes and stochastic gene expression........ 36 3.3 Bet hedging can be generated by either environmental or physiological noise.................................... 37 3.4 Active sensing of an uninformative cue to can constitute a bet-hedging strategy.................................. 39 3.4.1 Uninformative cues can be reliable............... 43 3.5 Conclusions................................ 44 4 The Quick and the Dead: Microbial Demography at the Yeast Ther- mal Limit 46 4.1 Introduction................................ 46 4.2 Results................................... 48 4.2.1 TrackScar measures the fecundity and mortality of single cells 48 4.2.2 Identifying diverse responses to heat stress........... 50 vi 4.2.3 Heat sensitivity is associated with the emergence of heteroge- neous subpopulations....................... 51 4.2.4 Increased mortality explains slow population growth for some heat sensitive strains....................... 53 4.2.5 Fecundity can be positively or negatively associated with age during stress............................ 56 4.2.6 Heat stress can cause premature senescence or early life mortality 57 4.2.7 Early life mortality is associated with a failure to inherit mi- tochondria............................. 59 4.3 Discussion................................. 62 4.3.1 TrackScar is a powerful method to measure fecundity and mor- tality................................ 63 4.3.2 Asymmetries during cell division contribute to phenotypic di- versity............................... 63 4.3.3 Mortality or slower division rate can limit growth during heat stress................................ 64 4.3.4 Fecundity and mortality can be age structured during heat stress 65 4.3.5 Diverse physiological factors delimit the niche of S. cerevisiae 66 4.4 Methods.................................. 67 A Supplementary Information for The Quick and the Dead 68 A.1 Introduction................................ 68 A.2 Results................................... 69 A.2.1 TrackScar minimally affects cellular physiology......... 69 A.2.2 TrackScar provides a sensitive measure of differences in fecundity 70 A.2.3 Population Growth Rate and Mean Fecundity Are Well Cor- related............................... 71 A.2.4 Six Hours of Recovery Is Sufficient To Distinguish Live from Dead Cells............................. 71 A.2.5 Heat stress can alter the distribution of ages in a population. 72 vii A.3 Supplementary Methods......................... 73 A.3.1 TrackScar Staining........................ 73 A.3.2 Microscopy............................ 74 A.3.3 Image processing and data analysis............... 75 A.3.4 Data collection and microscopy for time series......... 76 A.3.5 Growth curves........................... 77 A.3.6 Strain construction........................ 77 Bibliography 82 Biography 93 viii List of Tables 2.1 Strains used in Chapter2........................ 31 2.2 Plasmids used in Chapter2....................... 32 2.3 Primers used in Chapter2........................ 33 A.1 Strains used in Chapter4........................ 78 ix List of Figures 2.1 Ura3p mediates a phenotypic trade-off.................8 2.2 Sensing estradiol can control a phenotypic trade-off.......... 10 2.3 Sensing estradiol can actualize a bet-hedging strategy......... 13 2.4 Plasticity to estradiol does not always carry a cost........... 15 2.5 Fluorescent tags do not affect the cost of plasticity.......... 17 2.6 Mutation can tune bet-hedging strategies based on active sensing.. 19 2.7 Tuning Ura3p stability with degron tags................ 25 3.1 A schematic of the fitness consequences of bet hedging and adaptive plasticity.................................. 38 3.2 A schematic of the developmental mechanism undelying bet hedging and active sensing............................. 40 3.3 A schematic of a bet-hedging strategy based on active sensing.... 42 4.1 TrackScar measures the fecundity and viability of individual yeast... 50 4.2 Genetic diversity in thermotolerance among environmental yeast isolates 52 4.3 Subpopulations of cells with low fecundity in heat sensitive strains.. 54 4.4 The contribution of mortality to slow growth during heat stress... 55 4.5 Age structure of fecundity of S288C................... 57 4.6 The age structure of fecundity and mortality during heat stress... 58 4.7 Mitochondrial inheritance defects in a strain with early life mortality 60 4.8 Mitochondrial morphology during heat stress.............. 62 x A.1 TrackScar is sensitive and minimally affects cellular physiology.... 70 A.2 Correlation between TrackScar and growth curves........... 79 A.3 Heat stress can alter the distribution of ages in a population..... 80 A.4 Histograms of all sensitive and robust strains examined........ 81 xi List of Abbreviations and Symbols Abbreviations SC+5FOA Synthetic yeast media supplemented by uracil and 5-fluororotic acid. SC-ura Synthetic yeast media lacking uracil. xii Acknowledgements Thank-you to Paul Magwene for staying excited about my work; to Ryan Baugh for teaching me to write a paper; to Daniel Lew, Nicolas Buchler, Katia Koelle, Daniel McShea, Debra Murray, Daniel Skelly, and Helen Murphy for helpful conversations; to David McCandlish for a critically important citation; and to Kriti Sharma for her inspiration, determined optimism, and unflagging love. This work was supported in part by NIH (P50GM081883) and NSF (MCB1330545) awards. xiii 1 Introduction The expansion, persistence, decline, or extinction of populations is ultimately due to the rates of birth and death of individuals. However, since it is difficult to measure the fitness of individual cells, microorganisms are often treated as populations. A population-level view is appropriate when the phenotypes of individuals are similar to the average phenotype, but can obscure the underlying demographic and molecu- lar mechanisms that lead to population-level phenotypes when there is pronounced variability between individuals. Populations
Details
-
File Typepdf
-
Upload Time-
-
Content LanguagesEnglish
-
Upload UserAnonymous/Not logged-in
-
File Pages106 Page
-
File Size-