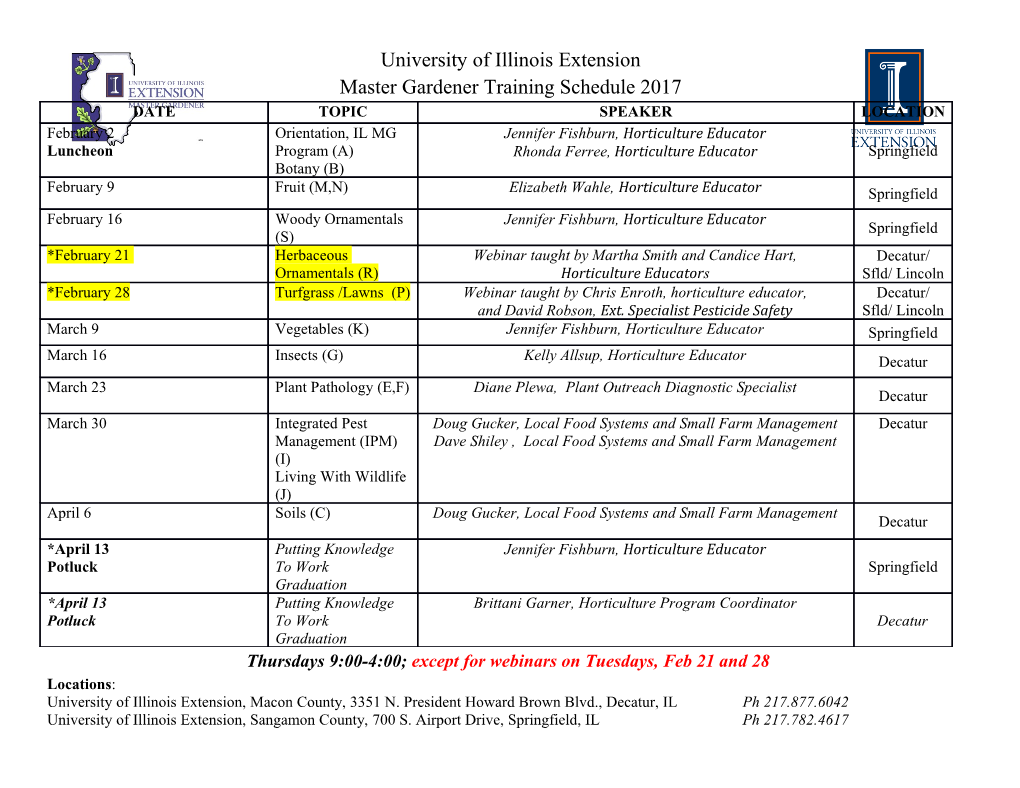
ST 520 Statistical Principles of Clinical Trials Lecture Notes (Modified from Dr. A. Tsiatis’ Lecture Notes) Daowen Zhang Department of Statistics North Carolina State University c 2009 by Anastasios A. Tsiatis and Daowen Zhang TABLE OF CONTENTS ST 520, A. Tsiatis and D. Zhang Contents 1 Introduction 1 1.1 Scopeandobjectives .............................. .... 1 1.2 Brief Introduction to Epidemiology . ......... 2 1.3 Brief Introduction and History of Clinical Trials . .............. 12 2 Phase I and II clinical trials 18 2.1 PhasesofClinicalTrials . ...... 18 2.2 PhaseIIclinicaltrials . .. .. ...... 20 2.2.1 Statistical Issues and Methods . ...... 21 2.2.2 Gehan’sTwo-StageDesign. ... 28 2.2.3 Simon’sTwo-StageDesign . ... 29 3 Phase III Clinical Trials 35 3.1 Whyareclinicaltrialsneeded . ....... 35 3.2 Issues to consider before designing a clinical trial . ............... 36 3.3 EthicalIssues ................................... ... 39 3.4 The Randomized Clinical Trial . ....... 40 3.5 Review of Conditional Expectation and Conditional Variance............ 43 4 Randomization 49 4.1 Design-basedInference . ...... 49 4.2 FixedAllocationRandomization. ........ 53 4.2.1 SimpleRandomization . .. 56 4.2.2 Permutedblockrandomization. ..... 57 4.2.3 StratifiedRandomization. .... 59 4.3 Adaptive Randomization Procedures . ........ 66 4.3.1 Efronbiasedcoindesign . ... 66 4.3.2 UrnModel(L.J.Wei)............................. 67 4.3.3 Minimization Method of Pocock and Simon . ..... 67 i TABLE OF CONTENTS ST 520, A. Tsiatis and D. Zhang 4.4 ResponseAdaptiveRandomization . ....... 70 4.5 MechanicsofRandomization. ...... 71 5 Some Additional Issues in Phase III Clinical Trials 74 5.1 BlindingandPlacebos ............................. .... 74 5.2 Ethics .......................................... 75 5.3 TheProtocolDocument ............................. ... 77 6 Sample Size Calculations 81 6.1 HypothesisTesting ............................... .... 81 6.2 Deriving sample size to achieve desired power . ........... 87 6.3 Comparingtworesponserates . ...... 88 6.3.1 Arcsinsquareroottransformation . ....... 92 7 Comparing More Than Two Treatments 96 7.1 Testing equality using independent normally distributedestimators . 97 7.2 Testing equality of dichotomous response rates . ............. 98 7.3 Multiplecomparisons. .. .. .102 7.4 K-sample tests for continuous response . ..........109 7.5 Sample size computations for continuous response . .............112 7.6 EquivalencyTrials ............................... .113 8 Causality, Non-compliance and Intent-to-treat 118 8.1 Causality and Counterfactual Random Variables . ............118 8.2 Noncompliance and Intent-to-treat analysis . .............122 8.3 A Causal Model with Noncompliance . .. .124 9 Survival Analysis in Phase III Clinical Trials 131 9.1 Describing the Distribution of Time to Event . ...........132 9.2 Censoring and Life-Table Methods . .......136 9.3 Kaplan-Meier or Product-Limit Estimator . ..........140 ii TABLE OF CONTENTS ST 520, A. Tsiatis and D. Zhang 9.4 Two-sampleTests................................. .144 9.5 PowerandSampleSize.............................. .149 9.6 K-SampleTests ................................... 157 9.7 Sample-size considerations for the K-sample logrank test ..............160 10 Early Stopping of Clinical Trials 164 10.1 General issues in monitoring clinical trials . ..............164 10.2 Information based design and monitoring . ...........167 10.3TypeIerror..................................... 171 10.3.1 Equalincrementsofinformation . .......175 10.4 Choiceofboundaries . .. .. .177 10.4.1 Pocockboundaries . .. .. 178 10.4.2 O’Brien-Fleming boundaries . .. .179 10.5 Power and sample size in terms of information . ...........181 10.5.1 InflationFactor ............................... 184 10.5.2 Informationbasedmonitoring . .. .188 10.5.3 Averageinformation . .189 10.5.4 Steps in the design and analysis of group-sequential tests with equal incre- mentsofinformation ..............................193 iii CHAPTER 1 ST 520, A. TSIATIS and D. Zhang 1 Introduction 1.1 Scope and objectives The focus of this course will be on the statistical methods and principles used to study disease and its prevention or treatment in human populations. There are two broad subject areas in the study of disease; Epidemiology and Clinical Trials. This course will be devoted almost entirely to statistical methods in Clinical Trials research but we will first give a very brief intro- duction to Epidemiology in this Section. EPIDEMIOLOGY: Systematic study of disease etiology (causes and origins of disease) us- ing observational data (i.e. data collected from a population not under a controlled experimental setting). Second hand smoking and lung cancer • Air pollution and respiratory illness • Diet and Heart disease • Water contamination and childhood leukemia • Finding the prevalence and incidence of HIV infection and AIDS • CLINICAL TRIALS: The evaluation of intervention (treatment) on disease in a controlled experimental setting. The comparison of AZT versus no treatment on the length of survival in patients with • AIDS Evaluating the effectiveness of a new anti-fungal medication on Athlete’s foot • Evaluating hormonal therapy on the reduction of breast cancer (Womens Health Initiative) • PAGE 1 CHAPTER 1 ST 520, A. TSIATIS and D. Zhang 1.2 Brief Introduction to Epidemiology Cross-sectional study In a cross-sectional study the data are obtained from a random sample of the population at one point in time. This gives a snapshot of a population. Example: Based on a single survey of a specific population or a random sample thereof, we determine the proportion of individuals with heart disease at one point in time. This is referred to as the prevalence of disease. We may also collect demographic and other information which will allow us to break down prevalence broken by age, race, sex, socio-economic status, geographic, etc. Important public health information can be obtained this way which may be useful in determin- ing how to allocate health care resources. However such data are generally not very useful in determining causation. In an important special case where the exposure and disease are dichotomous, the data from a cross-sectional study can be represented as D D¯ E n11 n12 n1+ E¯ n21 n22 n2+ n+1 n+2 n++ where E = exposed (to risk factor), E¯ = unexposed; D = disease, D¯ = no disease. In this case, all counts except n++, the sample size, are random variables. The counts (n11, n12, n21, n22) have the following distribution: (n , n , n , n ) multinomial(n ,P [DE],P [DE¯ ],P [DE¯],P [D¯E¯]). 11 12 21 22 ∼ ++ With this study, we can obtain estimates of the following parameters of interest n prevalence of disease P [D] (estimated by +1 ) n++ n probability of exposure P [E] (estimated by 1+ ) n++ PAGE 2 CHAPTER 1 ST 520, A. TSIATIS and D. Zhang n prevalence of disease among exposed P [D E] (estimated by 11 ) | n1+ n prevalence of disease among unexposed P [D E¯] (estimated by 21 ) | n2+ ... We can also assess the association between the exposure and disease using the data from a cross-sectional study. One such measure is relative risk, which is defined as P [D E] ψ = | . P [D E¯] | It is easy to see that the relative risk ψ has the following properties: ψ > 1 positive association; that is, the exposed population has higher disease probability • ⇒ than the unexposed population. ψ =1 no association; that is, the exposed population has the same disease probability • ⇒ as the unexposed population. ψ < 1 negative association; that is, the exposed population has lower disease probability • ⇒ than the unexposed population. Of course, we cannot state that the exposure E causes the disease D even if ψ > 1, or vice versa. In fact, the exposure E may not even occur before the event D. Since we got good estimates of P [D E] and P [D E¯] | | n n P [D E]= 11 , P [D E¯]= 21 , | n1+ | n2+ b b the relative risk ψ can be estimated by P [D E] n /n ψ = | = 11 1+ . P [D E¯] n21/n2+ b b | b Another measure that describes the association between the exposure and the disease is the odds ratio, which is defined as P [D E]/(1 P [D E]) θ = | − | . P [D E¯]/(1 P [D E¯]) | − | Note that P [D E]/(1 P [D E]) is called the odds of P [D E]. It is obvious that | − | | PAGE 3 CHAPTER 1 ST 520, A. TSIATIS and D. Zhang ψ > 1 θ> 1 • ⇐⇒ ψ =1 θ =1 • ⇐⇒ ψ < 1 θ< 1 • ⇐⇒ Given data from a cross-sectional study, the odds ratio θ can be estimated by P [D E]/(1 P [D E]) n /n /(1 n /n ) n /n n n θ = | − | = 11 1+ − 11 1+ = 11 12 = 11 22 . P [D E¯]/(1 P [D E¯]) n21/n2+/(1 n21/n2+) n21/n22 n12n21 b b − b | − | b b It can be shown that the variance of log(θ) has a very nice form given by b1 1 1 1 var(log(θ)) = + + + . n11 n12 n21 n22 d b The point estimate θ and the above variance estimate can be used to make inference on θ. Of course, the total sampleb size n++ as well as each cell count have to be large for this variance formula to be reasonably good. A (1 α) confidence interval (CI) for log(θ) (log odds ratio) is − log(θ) z [Var(log(θ))]1/2. ± α/2 b d b Exponentiating the two limits of the above interval will giveusaCIfor θ with the same confidence level (1 α). − Alternatively, the variance of θ can be estimated (by the delta method) b 1 1 1 1 Var(θ)= θ2 + + + , n11 n12 n21 n22 d b b and a (1 α) CI for θ is obtained as − θ z [Var(θ)]1/2. ± α/2 b d b For example, if we want a 95% confidence interval for log(θ) or θ, we will use z0.05/2 = 1.96 in the above formulas. From the definition of the odds-ration, we see that if the disease under study is a rare one, then P [D E] 0, P [D E¯] 0. | ≈ | ≈ PAGE 4 CHAPTER 1 ST 520, A. TSIATIS and D. Zhang In this case, we have θ ψ. ≈ This approximation is very useful. Since the relative risk ψ has a much better interpretation (and hence it is easier to communicate with biomedical researchers using this measure), in stud- ies where we cannot estimate the relative risk ψ but we can estimate the odds-ratio θ (see retrospective studies later), if the disease under studied is a rare one, we can approximately estimate the relative risk by the odds-ratio estimate.
Details
-
File Typepdf
-
Upload Time-
-
Content LanguagesEnglish
-
Upload UserAnonymous/Not logged-in
-
File Pages200 Page
-
File Size-