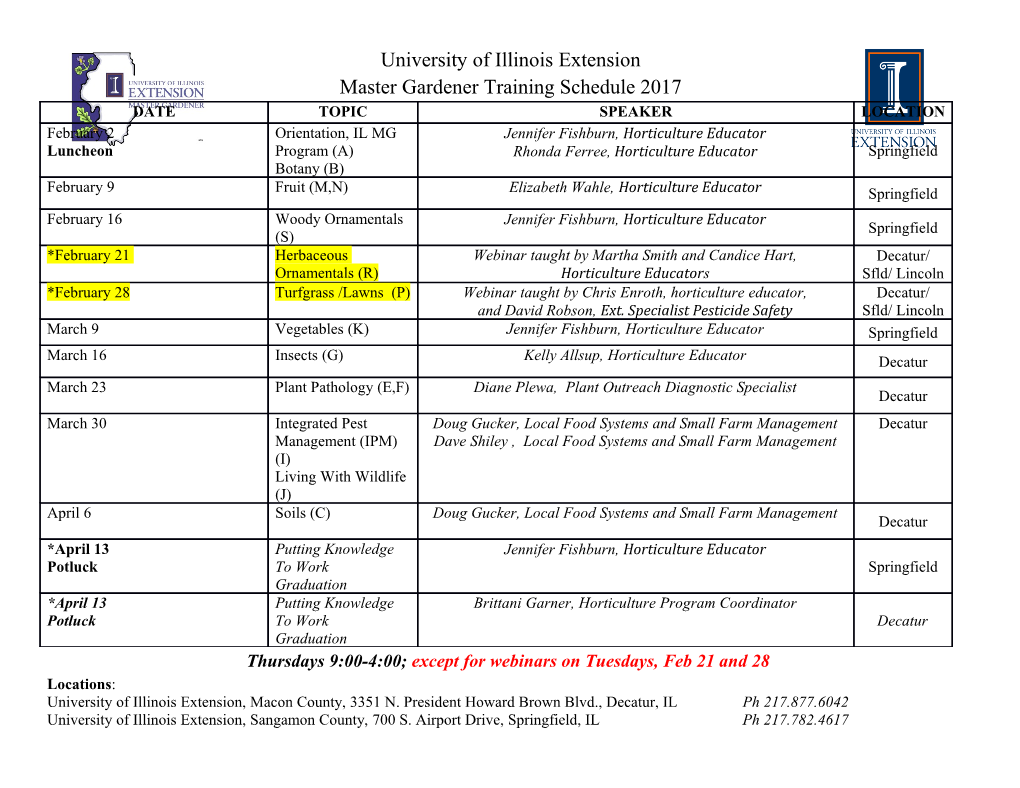
Lecture 4: Relaxing the Assumptions of the Linear Model R.G. Pierse 1 Overview 1.1 The Assumptions of the Classical Model E(ui) = 0 ; i = 1; ··· ; n (A1) 2 2 E(ui ) = σ ; i = 1; ··· ; n (A2) E(uiuj) = 0 ; i; j = 1; ··· ; n j 6= i (A3) X values are fixed in repeated sampling (A4) 0 The variables Xj are not perfectly collinear. (A4 ) ui is distributed with the normal distribution (A5) In this lecture we look at the implications of relaxing two of these assumptions: A2 and A3. Assumption A2 is the assumption of homoscedasticity, that the error variance is constant over all observations. If this assumption does not hold then the errors are heteroscedastic and 2 2 E(ui ) = σi ; i = 1; ··· ; n 2 where the subscript i on σi indicates that the error variance can be different for each observation. Assumption A3 is the assumption that the errors are serially uncorrelated. If this assumption does not hold then we say that the errors are serially correlated, or equivalently, that they exhibit autocorrelation. Symbolically E(uiuj) 6= 0 j 6= i 1 2 Autocorrelation Here we consider relaxing Assumption A3 and allowing the errors to exhibit au- tocorrelation. Symbolically E(uiuj) 6= 0 j 6= i : (2.1) Autocorrelation really only makes sense in time-series data where there is a natural ordering for the observations. Hence we assume for the rest of this section that we are dealing with time-series data and use the suffices t and s, rather than i and j to denote observations. 2.1 The First Order Autoregressive Model The assumption (2.1) is too general to deal with as it stands and we need to have a more precise model of the form that the autocorrelation takes. Specifically, we consider the hypothesis that the errors follow a first-order autoregressive or AR(1) scheme ut = ρut−1 + "t ; t = 1; ··· ;T (2.2) −1 < ρ < 1 where ut and "t are assumed to be independent error processes and "t has the standard properties: 2 2 E("t) = 0 ; E("t ) = σ" ; t = 1; ··· ;T and E("t"s) = 0 ; t; s = 1; ··· ; T s 6= t : By successive substitution in (2.2) we can write 2 t−1 ut = "t + ρεt−1 + ρ "t−2 + ··· + ρ "1 + u0 where the initial value u0 is taken as fixed with u0 = 0. Hence it follows that E(ut) = 0. Consider the variance of ut where ut follows a first order autoregressive process: 2 2 E(ut ) = E(ρut−1 + "t) 2 2 2 = ρ E(ut−1) + E("t ) + 2ρ E(ut−1"t) but the final term is zero since ut and "t are assumed independent and, in the first 2 2 term, E(ut−1) = E(ut ) since assumption A2 is still assumed to hold, so that σ2 E(u2) = " : t 1 − ρ2 2 Similarly, the first order covariance E(utut−1) = E(ρut−1 + "t) ut−1 2 = ρ E(ut−1) + ρ E(ut−1"t) σ2ρ = " 1 − ρ2 and, more generally, σ2ρs E(u u ) = " ; s ≥ 0 : t t−s 1 − ρ2 The autocorrelation between ut and ut−s decreases as the distance between the observations, s, increases. If ρ is negative, then this autocorrelation alternates in sign. 2.2 Generalised Least Squares Consider the multiple regression model Yt = β1 + β2X2t + ··· + βkXkt + ut ; t = 1; ··· ;T (2.3) Lagging this equation and multiplying by ρ gives ρYt−1 = ρβ1 + ρβ2X2;t−1 + ··· + ρβkXk;t−1 + ρut−1 (2.4) and, subtracting from the original equation, Yt − ρYt−1 = (1 − ρ)β1 + β2(X2t − ρX2;t−1) + ··· + βk(Xkt − ρXk;t−1) + ut − ρut−1 or ∗ ∗ ∗ ∗ Yt = β1 + β2X2t + ··· + βkXkt + "t ; t = 2; ··· ;T (2.5) ∗ ∗ where Yt = Yt − ρYt−1 and Xjt = Xjt − ρXj;t−1 are transformed variables known as quasi-differences. By transforming the equation, the error process has been tranformed into one that obeys all the classical assumptions so that, if the value of ρ is known, estimation of (2.5) gives the BLUE of the model. This estimator is known as the Generalised Least Squares or GLS estimator. Note that by quasi- differencing we lose one observation from the sample, because the first observation cannot be quasi-differenced. As long as T is large enough, we don't need to worry about this. For the simple regression model with only one explanatory variable PT (xt − ρxt−1)(yt − ρyt−1) eb = t=2 PT 2 t=2(xt − ρxt−1) is the GLS estimator and σ2 V ar(eb) = " : PT 2 t=2(xt − ρxt−1) 3 2.3 Estimating ρ: The Cochrane-Orcutt Procedure In practice, the autoregressive coefficient ρ is unknown and so has to be estimated. The Cochrane-Orcutt (1949) method is an iterative procedure to implement a feasible GLS estimator and estimate both ρ and the regression parameters β efficiently. Step 1: Estimate the regression equation Yt = β1 + β2X2t + ··· + βkXkt + ut ; t = 1; ··· ;T by OLS and form the OLS residuals et. Step 2: Run the regression et = ρet−1 + vt : to give the OLS estimator PT e e ρ = t=2 t t−1 b PT 2 t=2 et + + Step 3: Form the quasi-differenced variables Yt = Yt − ρYb t−1, and Xj;t = Xj;t − ρXb j;t−1, j = 2; ··· ; k and run the regression + + + + + Yt = β1 + β2X2t + ··· + βkXkt + "t ; t = 2; ··· ;T This gives a new set of estimates for the β parameters, and a new set of residuals, + et . Steps 2 and 3 are then executed repeatedly to derive new estimates of β and ρ and this process continues until there is no change in the estimate of ρ obtained from successive iterations. This is the full Cochrane-Orcutt iterative procedure. However, a simplified version is the two-step procedure which stops after the second step when the OLS estimator of ρ has been obtained. 2.4 Properties of OLS In the presence of autocorrelation, OLS is no longer BLUE. However, it remains unbiased since ! ! PT x y PT x u E(b) = E t=1 t t = b + E t=1 t t = b b PT 2 PT 2 t=1 xt t=1 xt where the last equality uses the result that E(ut) = 0. Because OLS is no longer BLUE, it follows that the OLS variance must be larger than that of the BLUE GLS estimator. Hence, in hypothesis testing, standard errors will be larger than they should be, so that coefficients will appear less significant than they truly are. This will lead to incorrect inference. 4 2.5 Testing for Autocorrelation In practice we do not know whether or not we have autocorrelation. If autocorre- lation is ignored, then although parameter estimates remain unbiased, inference based on those estimates will be incorrect. It is thus important to be able to test for autocorrelation in the OLS model. If autocorrelation is detected, then we can re-estimate the model by GLS. 2.5.1 The Durbin-Watson test This is the most famous test for autocorrelation. It is an exact test, in that the distribution of the test statistic holds for all sizes of sample. However, it is only valid when (a) the regressors X are fixed in repeated samples and (b) an intercept is included in the regression. Note in particular that the first assumption is violated if the regressors include any lagged dependent variables. The Durbin- Watson (1951) statistic is given by the formula PT (e − e )2 d = t=2 t t−1 : (2.6) PT 2 t=2 et Expanding the numerator gives PT e2 + PT e2 − 2 PT e e d = t=2 t t=2 t−1 t=2 t t−1 PT 2 t=2 et ! PT e e ' 2 1 − t=2 t t−1 = 2(1 − ρ) PT 2 b t=2 et PT 2 PT −1 2 PT 2 since t=2 et−1 ≡ t=1 et ' t=2 et . Note that 0 ≤ d ≤ 4, with E(d) = 2, and d < 2 indicative of positive autocorrelation, and d > 2 indicative of negative autocorrelation. In general, the distribution of d is a function of the explanatory variables X, so to compute the exact distribution of the statistic is very complicated. However, Durbin and Watson were able to show that the distribution of d is bounded by the distributions of two other statistics dL and dU which do not depend on X. Critical values of the distributions of dL and dU were tabulated by Durbin and Watson. The procedure for applying the Durbin-Watson test for testing positive auto- correlation is as follows: d < dL : reject the null hypothesis of no positive autocorrelation dL ≤ d ≤ dU : inconclusive region d > dU : do not reject the null hypothesis of no autocorrelation 5 Similarly, to test for negative autocorrelation: d > 4 − dL : reject the null hypothesis of no negative autocorrelation 4 − dU ≤ d ≤ 4 − dL : inconclusive region d < 4 − dU : do not reject the null hypothesis of no autocorrelation In both cases, there is an inconclusive region where the test does not allow us to say whether or not there is autocorrelation. For example, with T = 25 and k = 2, the 5% critical values are dL = 1:29 and dU = 1:45 so that, for any value of d in this range, the result of the test is indeterminate. The inconclusive region in the Durbin-Watson test is clearly a nuisance. One solution is to adopt the modified d test, where test values in the inconclusive region are treated as rejections of the null hypothesis.
Details
-
File Typepdf
-
Upload Time-
-
Content LanguagesEnglish
-
Upload UserAnonymous/Not logged-in
-
File Pages12 Page
-
File Size-