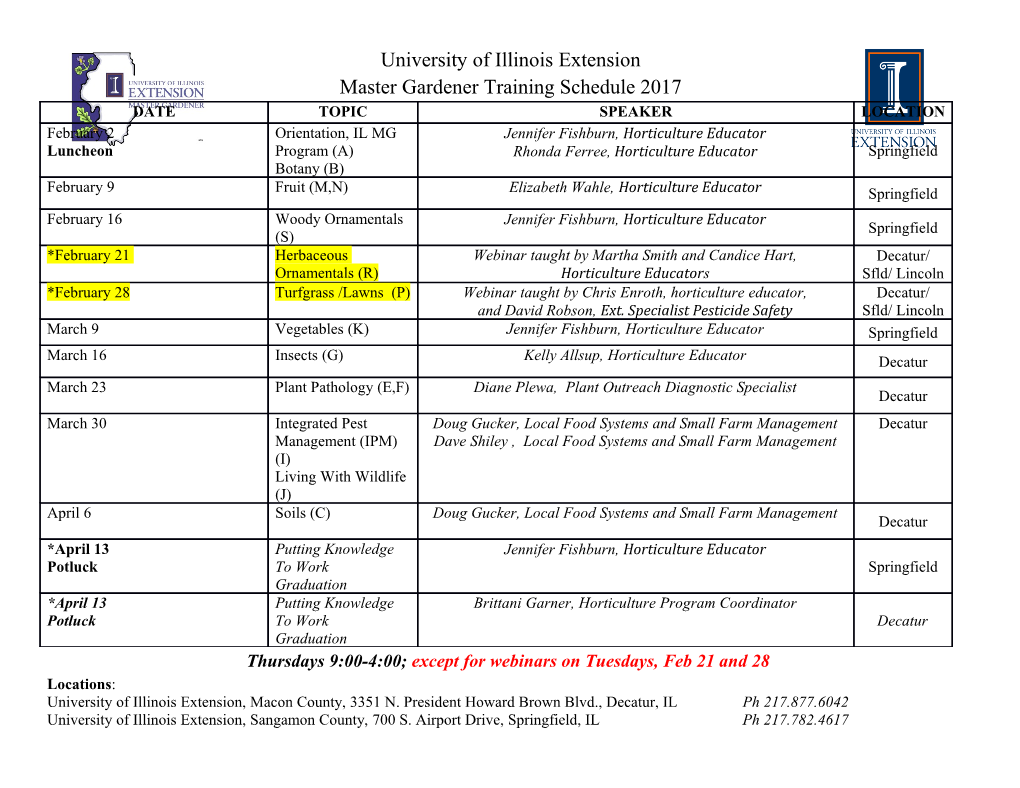
APRIL 2014 H A A N D S N Y D E R 1489 Influence of Surface Observations in Mesoscale Data Assimilation Using an Ensemble Kalman Filter SO-YOUNG HA AND CHRIS SNYDER National Center for Atmospheric Research, Boulder, Colorado (Manuscript received 6 April 2013, in final form 22 November 2013) ABSTRACT The assimilation of surface observations using an ensemble Kalman filter (EnKF) approach was success- fully performed in the Advanced Research version of the Weather Research and Forecasting Model (WRF) coupled with the Data Assimilation Research Testbed (DART) system. The mesoscale cycling experiment for the continuous ensemble data assimilation was verified against independent surface mesonet observations and demonstrated the positive impact on short-range forecasts over the contiguous U.S. (CONUS) domain throughout the month-long period of June 2008. The EnKF assimilation of surface observations was found useful for systematically improving the simulation of the depth and the structure of the planetary boundary layer (PBL) and the reduction of surface bias errors. These benefits were extended above PBL and resulted in a better precipitation forecast for up to 12 h. With the careful specification of observation errors, not only the reliability of the ensemble system but also the quality of the following forecast was improved, especially in moisture. In this retrospective case study of a squall line, assimilation of surface observations produced analysis increments consistent with the structure and dynamics of the boundary layer. As a result, it enhanced the horizontal gradient of temperature and moisture across the frontal system to provide a favorable con- dition for the convective initiation and the following heavy rainfall prediction in the Oklahoma Panhandle. Even with the assimilation of upper-level observations, the analysis without the assimilation of surface ob- servations simulated a surface cold front that was much weaker and slower than observed. 1. Introduction boundary layer (PBL); the background error co- variances are important because, in statistical data assim- Compared to other routine in situ observations, sur- ilation schemes, they specify how surface observations will face observations (2-m temperature and moisture, 10-m influence the analysis. Second, forecast models typically winds) have relatively good spatial and temporal reso- have limited skill in the PBL owing to significant errors lution, but their use in atmospheric data assimilation has in the parameterizations of vertical mixing, surface proven substantially more difficult. Indeed, many state- fluxes, and land surface processes. This paper concen- of-the-art systems do not assimilate surface observations trates on the first issue and presents results showing the directly; at the European Centre for Medium-Range effectiveness of the ensemble Kalman filter (EnKF) for Weather Forecasts (ECMWF), for example, surface the assimilation of surface observations, which we at- observations are used in a two-dimensional surface tribute to the EnKF’s use of background error co- analysis that forces the land surface model but are not variances computed from an ensemble of short-range used in the variational assimilation scheme for the at- forecasts and that are therefore consistent with the local mosphere (de Rosnay et al. 2013). The difficulty of as- structure and dynamics of the PBL. similating surface observations arises from at least two Specifying the background error covariances in the issues. First, the assimilation scheme must specify the PBL is challenging because of complexity and variability background error covariances, especially in the vertical in the structure of the PBL and in the relation of surface and in relation to the structure of the planetary variables to the rest of the atmosphere. Physical in- tuition suggests that observed surface variables may Corresponding author address: Dr. So-Young Ha, NCAR/ often have strong correlations with variables within the MMM, 3450 Mitchelle Ln., P. O. Box 3000, Boulder, CO 80301. PBL and weak correlations with flow outside the PBL, E-mail: [email protected] depending on the time of day and the boundary layer DOI: 10.1175/MWR-D-13-00108.1 Ó 2014 American Meteorological Society 1490 MONTHLY WEATHER REVIEW VOLUME 142 regime. Nudging schemes for assimilation have incor- special treatment of surface observations, either through porated this intuition by requiring the nudging coefficients bias correction of the prior forecasts or by reducing their for surface observations to decrease to zero above the PBL observation-error variance, resulted in better fits of the top (Stauffer et al. 1991). Such modifications to the co- analysis and 6-h prior forecasts to unassimilated surface efficients are, however, heuristic, in that they are based on observations. These benefits did not, however, extend the developer’s assumptions for the relation of surface above the surface. variables to other variables and other levels. In the present study, we continue the exploration of Three-dimensional variational data assimilation assimilation of real surface observations in the EnKF (3DVAR) schemes typically use stationary covariances, approach using the Advanced Research version of the calculated from statistics of forecast differences, which Weather Research and Forecasting Model (WRF) can capture basic aspects of PBL dynamics such as the coupled with the Data Assimilation Research Testbed correlation between vertical vorticity and horizontal (DART) system (WRF–DART) and examine the in- divergence at synoptic scales (Derber and Bouttier fluence of surface observations on mesoscale analyses in 1999). Nevertheless, because they use stationary back- an optimized environment over the contiguous U.S. ground covariances, 3DVAR schemes generally do not (CONUS) domain. Our goal is to investigate the effec- account for any dependence of analysis increments on tiveness of the EnKF in the assimilation of surface ob- the instantaneous structure, the diurnal cycle, or the servations and demonstrate improvements of the regime of the PBL (e.g., Benjamin et al. 2004). following forecasts in the boundary layer and farther More sophisticated statistical assimilation approaches, aloft, as well as at the surface. For this purpose, we such as four-dimensional variational data assimilation perform experiments with and without assimilation of (4DVAR) and the EnKF schemes, extract dynamical surface observations, and make forecasts from the mean information directly from the forecast model and pro- EnKF analysis for 72 h. Through a mesoscale convective duce analysis increments that depend on the local state case study, we examine how the assimilation of surface of the PBL and its recent history. In the EnKF, the data can provide a favorable condition for the initiation relative weighting in the analysis between the back- and the development of severe convection associated ground and observations, and the influence of observa- with a squall line and to what extent the analysis in- tions away from the station locations, are determined by crement affects the structure and evolution of the anisotropic and inhomogeneous background covariances. boundary layer. These covariances, in turn, are estimated from an en- Surface pressure observations reflect the distribution semble of short-range forecasts using the full, nonlinear of mass vertically integrated through the depth of the model, and the covariances will, therefore, change as the entire atmosphere, and their use in the EnKF has been PBL evolves. explored by Dirren et al. (2007) and Compo et al. (2006), Several previous studies have specifically examined for example. It will not be a main focus here, since they the assimilation of surface observations with an EnKF are not particularly sensitive to or informative of con- method. Hacker and Snyder (2005) considered simu- ditions in the PBL and their assimilation is subject to lated surface observations in a single-column model. neither of the two main issues noted in the first part of Their results confirmed the physical intuition that sur- this session. face observations are strongly correlated with, and The data assimilation system implemented here therefore when assimilated informative of, conditions largely ignores forecast model errors, despite their po- throughout the well-mixed PBL or even into the pre- tential importance in the PBL and therefore in the as- vious day’s residual layer. Fujita et al. (2007) assimilated similation of surface observations. The system includes actual surface observations in the EnKF. In addition to only a spatially and temporally varying, adaptive in- examining the role of forecast model error, they dem- flation (Anderson 2009), which compensates for un- onstrated improvements to the analysis of important derestimation of the analysis variance by the EnKF as mesoscale features for two severe weather cases as well well as underdispersion in the prior ensemble forecasts as improvements lasting 6–12 h in the subsequent fore- owing to unrepresented model error. Other possible casts. Meng and Zhang (2008) also briefly examined approaches aimed specifically at representing model assimilation of surface observations in a case study of uncertainties within the EnKF are employing multiple a mesoscale convective vortex but found little influence suites of physical parameterizations (Fujita et al. 2007; on the short-range forecast. Results from a longer, Meng and Zhang 2008; Hacker et al. 2011) and including 5-week period of assimilation appeared in Ancell et al. an additive-noise or ‘‘backscatter’’ scheme within the (2011). They did not examine the influence of surface model (Hacker et al. 2011; Berner et al. 2011). We will observations on the analysis directly, but did show that examine these approaches in a subsequent study. APRIL 2014 H A A N D S N Y D E R 1491 FIG. 1. Model domains with aviation routine weather report (METAR) stations reported at 0000 UTC 9 Jun 2008. Stations that are far away from the model terrain more than 100 m but less than 300 m marked as an open circle (o), and the ones with more than 300-m height dif- ference marked as a crisscross (3). Stations shown as filled dots have the height discrepancy less than 100 m.
Details
-
File Typepdf
-
Upload Time-
-
Content LanguagesEnglish
-
Upload UserAnonymous/Not logged-in
-
File Pages20 Page
-
File Size-