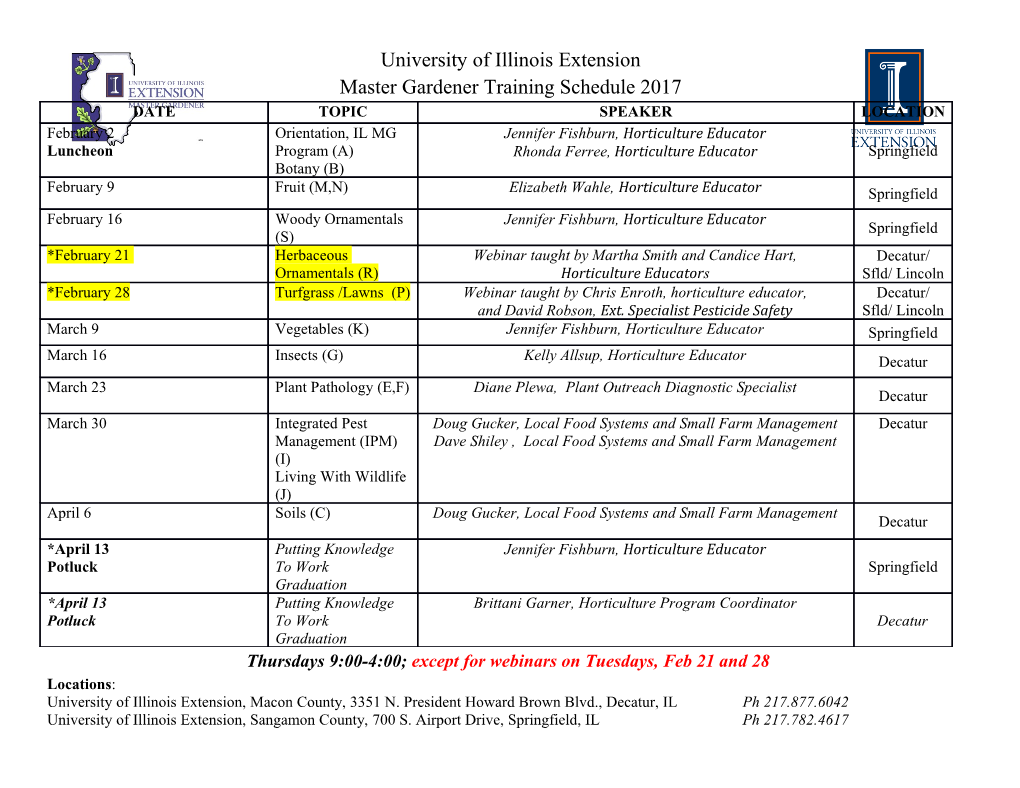
The Price of Stability for Network Design with Fair Cost Allocation Elliot Anshelevich∗ Anirban Dasguptay Jon Kleinberg z Ev´ a Tardosx Tom Wexler{ Tim Roughgardenk Abstract scheme can be derived from the Shapley value, and has a number of basic economic motivations. We Network design is a fundamental problem for show that the price of stability for network design which it is important to understand the effects with respect to this fair cost allocation is O(log k), of strategic behavior. Given a collection of self- where k is the number of users, and that a good interested agents who want to form a network con- Nash equilibrium can be achieved via best-response necting certain endpoints, the set of stable solutions dynamics in which users iteratively defect from a | the Nash equilibria | may look quite different starting solution. This establishes that the fair cost from the centrally enforced optimum. We study the allocation protocol is in fact a useful mechanism quality of the best Nash equilibrium, and refer to for inducing strategic behavior to form near-optimal the ratio of its cost to the optimum network cost equilibria. We discuss connections to the class of as the price of stability. The best Nash equilibrium potential games defined by Monderer and Shapley, solution has a natural meaning of stability in this and extend our results to cases in which users are context | it is the optimal solution that can be pro- seeking to balance network design costs with laten- posed from which no user will \defect". cies in the constructed network, with stronger results We consider the price of stability for network de- when the network has only delays and no construc- sign with respect to one of the most widely-studied tion costs. We also present bounds on the conver- protocols for network cost allocation, in which the gence time of best-response dynamics, and discuss cost of each edge is divided equally between users extensions to a weighted game. whose connections make use of it; this fair-division ∗Cornell University, Department of Computer Science, 1. Introduction Upson Hall, Ithaca, NY 14853. Supported by ITR grant 0311333. Email: [email protected]. yCornell University, Department of Computer Science, In many network settings, the system behavior Upson Hall, Ithaca, NY 14853. Supported by the Depart- arises from the actions of a large number of inde- ment of Computer Science. Email: [email protected]. pendent agents, each motivated by self-interest and zCornell University, Department of Computer Sci- optimizing an individual objective function. As a ence, Upson Hall, Ithaca, NY 14853. Email: klein- [email protected]. Supported in part by a David and Lucile result, the global performance of the system may Packard Foundation Fellowship and NSF grants 0081334 and not be as good as in a case where a central author- 0311333. ity can simply dictate a solution; rather, we need xCornell University, Department of Computer Science, to understand the quality of solutions that are con- Upson Hall, Ithaca, NY 14853. Supported in part by NSF grant CCR-032553, ITR grant 0311333, and ONR grant sistent with self-interested behavior. Recent theo- N00014-98-1-0589. Email: [email protected]. retical work has framed this type of question in the {Cornell University, Department of Computer Science, following general form: how much worse is the so- Upson Hall, Ithaca, NY 14853. Supported by ITR grant lution quality at a Nash equilibrium1, relative to 0311333. Email: [email protected]. kUC Berkeley, Computer Science Division, Soda Hall, 1Recall that a Nash equilibrium is a state of the system Berkeley, CA 94720. Supported by an NSF Postdoctoral Fel- in which no agent has an interest in unilaterally changing its lowship. Email: [email protected]. own behavior. the quality at a centrally enforced optimum? Ques- (Note that this cost can depend on the choices of tions of this genre have received considerable atten- the other users as well.) Although there are in tion in recent years, for problems including routing principle many possible cost-sharing mechanisms, [24, 25, 4], load balancing [5, 6, 16, 23], and facility research in this area has converged on a few mech- location [26]. anisms with good theoretical and empirical behav- An important issue to explore in this area is the ior; here we focus on the following particularly nat- middle ground between centrally enforced solutions ural one: the cost of each edge is shared equally and completely unregulated anarchy. In most net- by the set of all users whose paths contain it, so ce working applications, it is not the case that agents that Ci(S1; S2; : : : ; Sk) = . This X jfj : e 2 Sj gj are completely unrestricted; rather, they interact e2Si with an underlying protocol that essentially pro- equal-division mechanism has a number of basic eco- poses a collective solution to all participants, who nomic motivations; it can be derived from the Shap- can each either accept it or defect from it. As a re- ley value [20], and it can be shown to be the unique sult, it is in the interest of the protocol designer to cost-sharing scheme satisfying a number of differ- seek the best Nash equilibrium; this can naturally ent sets of axioms [9, 11, 20]. For the former rea- be viewed as the optimum subject to the constraint son, we will refer to it as the Shapley cost-sharing that the solution be stable, with no agent having mechanism. Note that the total edge cost of the de- an incentive to unilaterally defect from it once it signed network is equal to the sum of the costs in the is offered. Hence, one can view the ratio of the union of all Si, and the costs allocated to users in solution quality at the best Nash equilibrium rela- the Shapley mechanism completely pay for this total n tive to the global optimum as a price of stability, edge cost: Ci(S1; S2; : : : ; Sk) = 2[ ce. Pi=1 Pe iSi since it captures the problem of optimization sub- Now, the general question is to determine how ject to this constraint. Some recent work [1, 4] has this basic cost-sharing mechanism serves to influ- considered this definition (termed the \optimistic ence the strategic behavior of the users, and what price of anarchy" in [1]); it stands in contrast to the effect this has on the structure and overall cost larger line of work in algorithmic game theory on of the network one obtains. Given a solution to the price of anarchy [21] | the ratio of the worst the network design problem consisting of a vec- Nash equilibrium to the optimum | which is more tor of paths (S1; : : : ; Sk) for the n users, user i suited to worst-case analysis of situations with es- would be interested in deviating from this solu- sentially no protocol mediating interactions among 0 tion if there were an alternate si-ti path Si so that the agents. Indeed, one can view the activity of a 0 changing to Si would lower its cost under the result- protocol designer seeking a good Nash equilibrium 0 ing allocation: Ci(S1; : : : ; Si−1; S ; Si+1; : : : ; Sk) < as being aligned with the general goals of mecha- i Ci(S1; : : : ; Si−1; Si; Si+1; : : : ; Sk): We say that a set nism design | producing a game that yields good of paths is a Nash equilibrium if no user has an inter- outcomes when players act in their own self-interest. est in deviating. As we will see below, there exists a set of paths in Nash equilibrium for every instance Network Design Games. Network design is a of this network design game. (In this paper, we natural area in which to explore the price of stabil- will only be concerned with pure Nash equilibrium; ity, given the large body of work in the networking i.e. with equilibria where each user deterministically literature on methods for sharing the cost of a de- chooses a single path.) signed network | often a virtual overlay, multicast The goal of a network design protocol is to sug- tree, or other sub-network of the Internet | among gest for each user i a path Si so that the resulting a collection of participants. (See e.g. [9, 11] for set of paths is in Nash equilibrium and its total cost overviews of work in this area). exceeds that of an optimal set of paths by as small a A cost-sharing mechanism can be viewed as the factor as possible; this factor is the price of stability underlying protocol that determines how much a of the instance. It is useful at this point to consider network serving several participants will cost to a simple example that illustrates how the price of each of them. Specifically, say that each user i has stability can grow to a super-constant value (with a pair of nodes (si; ti) that it wishes to connect; it k). Suppose k players wish to connect the common chooses an si-ti path Si; and the cost-sharing mech- source s to their terminal ti, assume player i has anism then charges user i a cost of Ci(S1; : : : ; Sk). its own path of cost 1=i, and all players can share a t 1 1 1 1 1 2 3 k-1 k ... s s1 s2 s3 k-1 sk 1+ε 0 0 0 0 0 v Figure 1. An instance in which the price of stability converges to H(k) = Θ(log k) as " ! 0.
Details
-
File Typepdf
-
Upload Time-
-
Content LanguagesEnglish
-
Upload UserAnonymous/Not logged-in
-
File Pages10 Page
-
File Size-