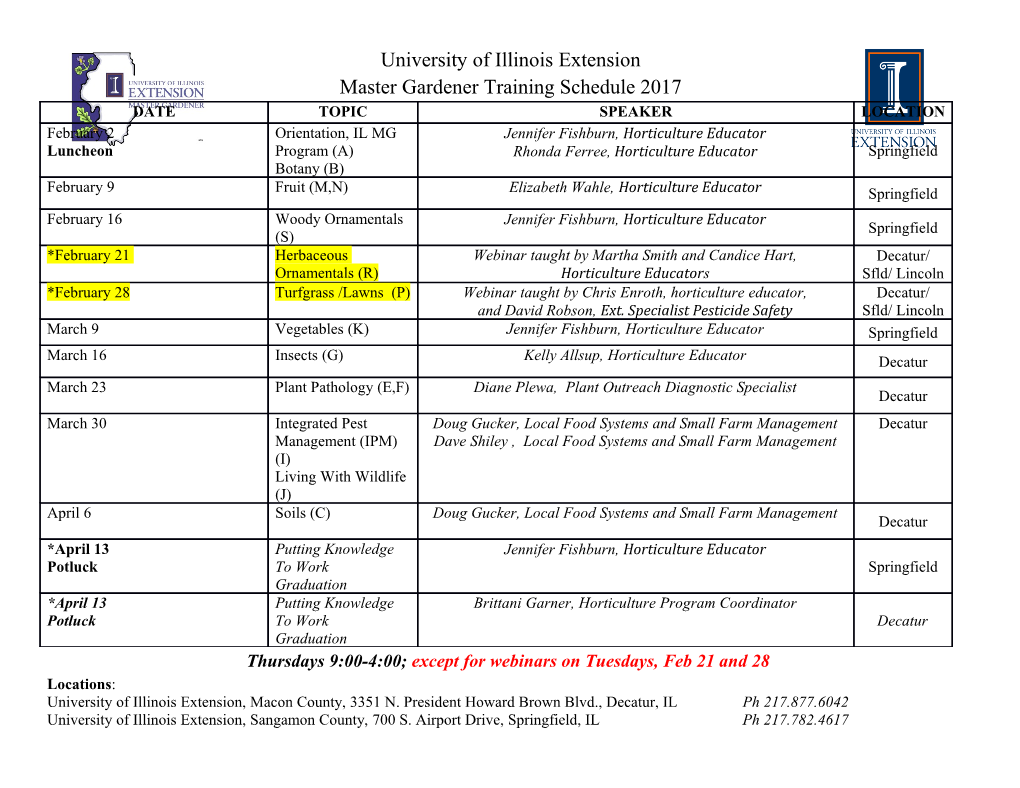
Introduction to Extreme Value Theory. Applications to Risk Analysis & Management Marie Kratz Abstract We present an overview of Univariate Extreme Value Theory (EVT) pro- viding standard and new tools to model the tails of distributions. One of the main is- sues in the statistical literature of extremes concerns the tail index estimation, which governs the probability of extreme occurrences. This estimation relies heavily on the determination of a threshold above which a Generalized Pareto Distribution (GPD) can be fitted. Approaches to this estimation may be classified into two classes, one qualified as ’supervised’, using standard Peak Over Threshold (POT) methods, in which the threshold to estimate the tail is chosen graphically according to the prob- lem, the other class collects unsupervised methods, where the threshold is algorith- mically determined. We introduce here a new and practically relevant method belonging to this second class. It is a self-calibrating method for modeling heavy tailed data, which we de- veloped with N. Debbabi and M. Mboup. Effectiveness of the method is addressed on simulated data, followed by applications in nero-science and finance. Results are compared with those obtained by more standard EVT approaches. Then we turn to the notion of dependence and the various ways to measure it, in particular in the tails. Through examples, we show that dependence is also a crucial topic in risk analysis and management. Underestimating the dependence among ex- treme risks can lead to serious consequences, as for instance those we experienced during the last financial crisis. We introduce the notion of copula, which splits the dependence structure from the marginal distribution, and show how to use it in prac- tice. Taking into account the dependence between random variables (risks) allows us to extend univariate EVT to mutivariate EVT. We only give the first steps of the latter, to motivate the reader to follow or to participate in the increasing research development on this topic. AMS 2000 subject classification. 60G70; 62G32; 62P05; 62G20; 91B30 Marie Kratz, ESSEC Business School, CREAR, Paris, France, (e-mail: [email protected]) 1 Contents Introduction to Extreme Value Theory. Applications to Risk Analysis & Management ::::::::::::::::::::::::::::::::::::::::::::::::::: 1 Marie Kratz 1 Introduction . 4 1.1 What is Risk? . 5 1.2 Impact of Extreme Risks . 6 2 Univariate EVT . 8 2.1 CLT versus EVT . 8 2.2 A limit theorem for Extremes: the Pickands theorem . 11 2.3 Supervised methods in EVT: standard thresholds methods 11 2.4 A self-calibrated method for heavy-tailed data . 15 3 Dependence . 22 3.1 Motivation . 22 3.2 Notion of dependence . 31 3.3 Copulas . 32 3.4 Notion of Rank Correlation . 36 3.5 Ranked Scatterplots. 37 3.6 Other type of dependence: Tail or Extremal dependence . 38 4 Multivariate EVT . 41 4.1 MEV distribution. 41 4.2 Copula Domain of Attraction . 42 5 Conclusion . 42 References . 43 3 4 Contents 1 Introduction XXIst Century: a Stochasc World Ukraine Lehman-Brothers Isis Financial Crisis WTC Katrina 9/11 Rita Sovereign Wilma Debt Crisis London Bombing EyjaSallajökull 7/7/2005 Volcanic erupon 2001 2002 2003 2004 2005 2006 2007 2008 2009 2010 2011 2012 2013 2014 2015 Atocha Ebola Madrid Arab Internet 11/3/2004 Spring Bubble Christchurch Earthquake Japanese Tsunami Thaï Floods Bataclan 14/11/2015 M. Dacorogna & M. Kratz Charlie Hebdo 1/7/2015 Fig. 1 Some of the extreme events that hit the World between 2001 and 2015 Quantitative risk analysis used to rely, until recently, on classical probabilistic modeling where fluctuations around the average were taken into account. The stan- dard deviation was the usual way to measure risk, like, for instance, in Markowitz portfolio theory[17], or in the Sharpe Ratio[25]. The evaluation of “normal” risks is more comfortable because it can be well modelled and predicted by the Gaus- sian model and so easily insurable. The series of catastrophes that hit the World at the beginning of this century (see Figure 1, natural (earthquakes, volcano eruption, tsunami, ...) or financial (subprime crisis, sovereign crisis) or political (Arab Spring, ISIS, Ukraine, ...), made it clear that it is crucial nowadays to take also extreme occurrences into account; indeed, although it concerns events that rarely occur (i.e. with a very small probability), their magnitude is such that their consequences are dramatic when they hit unprepared societies. Including extreme risks in probabilistic models is recognized nowadays as a nec- essary condition for good risk management in any institution, and not restricted anymore to reinsurance companies, who are the providers of covers for natural catastrophes. For instance in finance, minimizing the impact of extreme risks, or even ignoring them because of a small probability of occurrence, has been consid- ered by many professionals and supervisory authorities, as a factor of aggravation of the financial crisis of 2008-09. The American Senate and the Basel Committee Contents 5 on Banking Supervision confirm this statement in their reports. Therefore, includ- ing and evaluating correctly extreme risks has become very topical and crucial for building up the resilience of our societies. The literature on extremes is very broad; we present here an overview of some stan- dard and new methods in univariate Extreme Value Theory (EVT) and refer the reader to books on the topic [1, 8, 9, 15, 22, 23, 24] and also on EVT with applica- tions in finance or integrated in quantitative risk management [16, 18, 20]. Then we develop the concept of dependence to extend univariate EVT to multivariate EVT. All along applications in various fields including finance, insurance and quantitative risk management, illustrate the various concepts or tools. 1.1 What is Risk? Risk is a word widely used by many people and not only by professional risk man- agers. It is therefore useful to spend a bit of time analysing this concept. We start by looking at its definition in common dictionaries. There, we find that it is mainly identified to the notion of danger of loss: The Oxford English Dictionary: Hazard, a chance of bad consequences, loss or exposure to mischance. For financial risks: ”Any event or action that may adversely affect an organiza- tion’s ability to achieve its objectives and execute its strategies” or, alternatively, ”the quantifiable likelihood of loss or less-than-expected returns”. Webster’s College Dictionary (insurance): ”The chance of loss” or ”The degree of probability of loss” or ”The amount of possible loss to the insuring company” or ”A person or thing with reference to the risk involved in providing insurance” or ”The type of loss that a policy covers, as fire, storm, etc.” However, strictly speaking, risk is not simply associated to a danger. In its modern acceptance, it can also be seen as an opportunity for a profit. It is the main reason why people would accept to be exposed to risk. In fact, this view started to develop already in the 18th century. For instance, the French philosopher, Etienne de Condil- lac (1714-1780) defined risk as ”The chance of incurring a bad outcome, coupled, with the hope, if we escape it, to achieve a good one”. Another concept born from the management of risk is the insurance industry. In the 17th Century, the first insurance for buildings is created after the big London fire (1666). During the 18h century appears the notion that social institutions should protect people against risk. This contributed to the development of life insurance that was not really acceptable by religion at the time. In this context, the definition of ’Insurance’ as the transfer of risk from an individual to a group (company) takes its full meaning. The 19th century sees the continuous development of private insur- ance companies. Independently of any context, risk relates strongly to the notion of randomness and the uncertainty of future outcomes. The distinction between “uncertainty”’ and 6 Contents “risk” was first introduced by the American economist Frank H. Knight (1885- 1972), although they are related concepts. According to Knight, risk can be defined as randomness with knowable probabilities, contrary to uncertainty, which is ran- domnessRisk and with Risk unknowable Measures probabilities. We could then define risk as a measurable uncertainty,Risk can be the measured ’known-unknowns’ in terms of probability distributions, according to Donald Rumsfeld’s terminology, however, it is some time useful to express it with one number. whereasThis uncertaintynumber is called risk is measure unmeasurable,. the ’unknown-unknowns’ (D.Rumsfeld). Of course,We research want a measure and that improvedcan give us a risk knowledge in form of a capital help to transform some uncertainty into risk withamount knowable. probabilities. The risk measure should have the following properties In what(coherence) follows,: we focus on the notion of risk, in particular extreme risk, and its 1. Scalable (twice the risk should give a twice bigger measure), quantification.2. Ranks risks We correctly choose (bigger risks a possibleget bigger measure), definition of risk, used within probabilistic framework,3. Allows namely for diversification the variation (aggregated risks from should have the a expectedlower outcome over time. measure), 4. Translation invariance (proper treatment of riskless cash flows), There are5. numerousRelevance (non-zero ways risks get to non-zero measure risk measures). risk and many risk measures have been devel- oped in the literature. Most modern measures of the risk in a portfolio are statistical Economy of Risk in Insurance Michel M. Dacorogna 7 quantities describing the conditionalMoF, ZH, Sept. – Dec. 2009 or unconditional loss distribution of the port- folio over some predetermined horizon. Here we present only popular ones, used in particular in regulation. We recall their definition and refer to Emmer et al. ([10]) and references therein for their mathematical properties.
Details
-
File Typepdf
-
Upload Time-
-
Content LanguagesEnglish
-
Upload UserAnonymous/Not logged-in
-
File Pages44 Page
-
File Size-