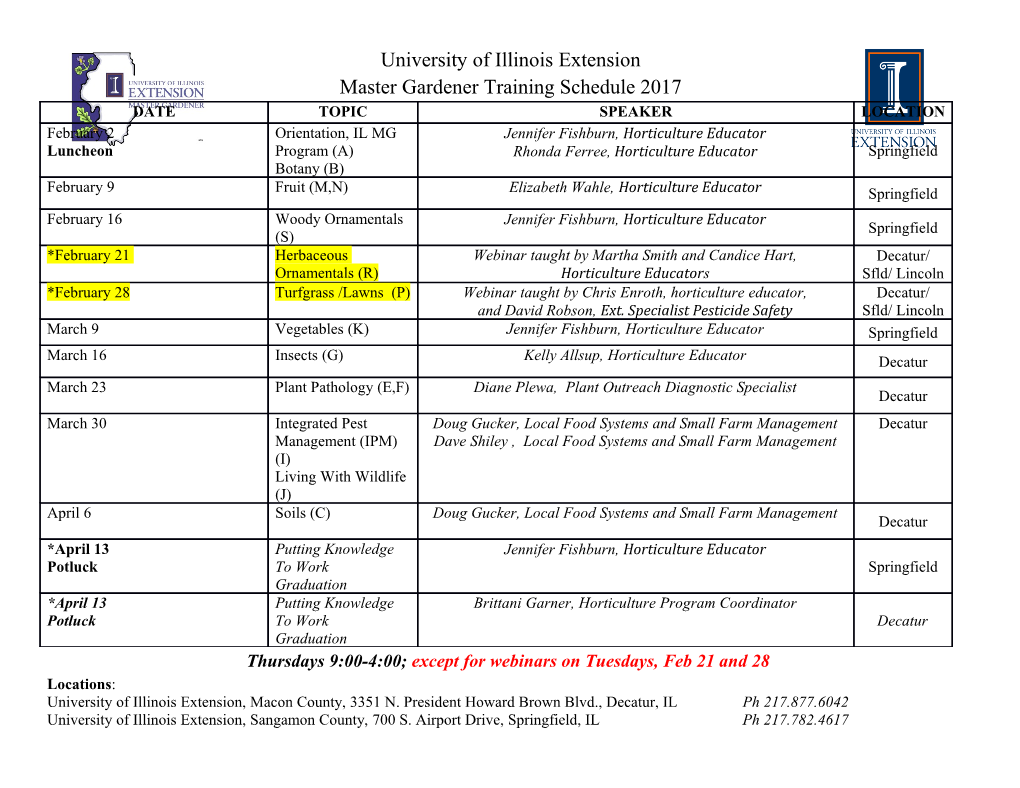
View metadata, citation and similar papers at core.ac.uk brought to you by CORE provided by DSpace at Rice University Free energy landscape for the binding process of Huperzine A to acetylcholinesterase Fang Baia,b, Yechun Xuc, Jing Chenc, Qiufeng Liuc, Junfeng Gua, Xicheng Wanga, Jianpeng Mad,e, Honglin Lif,1, José N. Onuchicg,1, and Hualiang Jiangc,f,1 aDepartment of Engineering Mechanics, State Key Laboratory of Structural Analysis for Industrial Equipment, and bFaculty of Chemical, Environmental, and Biological Science and Technology, Dalian University of Technology, Dalian 116023, China; cDrug Discovery and Design Center, State Key Laboratory of Drug Research, Shanghai Institute of Materia Medica, Chinese Academy of Sciences, Shanghai 201203, China; dDepartment of Bioengineering, and gCenter for Theoretical Biological Physics and Department of Physics, Rice University, Houston, TX 77005; eVerna and Marrs McLean Department of Biochemistry and Molecular Biology, Baylor College of Medicine, Houston, TX 77030; and fState Key Laboratory of Bioreactor Engineering and Shanghai Key Laboratory of New Drug Design, School of Pharmacy, East China University of Science and Technology, Shanghai 200237, China Contributed by José N. Onuchic, January 30, 2013 (sent for review December 17, 2012) Drug-target residence time (t = 1/koff,wherekoff is the dissociation further expansion of the drug design approaches from an affinity- rate constant) has become an important index in discovering better- emphasized paradigm to a BK-emphasized paradigm. Recently, or best-in-class drugs. However, little effort has been dedicated to energy landscape theory, which was originally used to investigate developing computational methods that can accurately predict this protein folding problems (13–15), has been extended to study k kinetic parameter or related parameters, off and activation free ligand–macromolecule binding (16–21). To extend the ideas from Δ ≠ energy of dissociation ( Goff). In this paper, energy landscape theory energy landscape theory for protein folding and function to that has been developed to understand protein folding and function quantitative drug design, it is necessary to develop a computational is extended to develop a generally applicable computational frame- method that may produce a rigorous and precise binding free en- work that is able to construct a complete ligand-target binding free ergy landscape (BFEL) for a ligand binding to its target protein. energy landscape. This enables both the binding affinity and the If such a landscape is produced, the binding free energy profile binding kinetics to be accurately estimated. We applied this method and free energy barriers for association and dissociation may be to simulate the binding event of the anti-Alzheimer’s disease drug k k obtained from the BFEL and and values could thus be BIOPHYSICS AND − − on off ( ) Huperzine A to its target acetylcholinesterase (AChE). The com- derived according to the Eyring equation if the transmission fac- COMPUTATIONAL BIOLOGY putational results are in excellent agreement with our concurrent tors are known. For most ligand–protein interactions, the trans- experimental measurements. All of the predicted values of binding mission factors are unknown; therefore it is difficult to directly free energy and activation free energies of association and dissoci- predict the absolute values of kon and koff (22). Nevertheless, for ation deviate from the experimental data only by less than 1 kcal/ the drug design case we expect that changes to the ligand will affect mol. The method also provides atomic resolution information for the barriers much more than the transmission coefficient, which for (−)−Huperzine A binding pathway, which may be useful in design- small molecules should not be large. Therefore, prediction of the PHYSICS ing more potent AChE inhibitors. We expect this methodology to be activation free energy of dissociation should be sufficient for drug widely applicable to drug discovery and development. discovery. Recently, an increasing number of groups have been in- thermodynamics | flexible docking | metastable states | transition states terested in kinetic simulation and prediction for ligand–protein binding. By performing 495 molecular dynamics simulations, raditionally, drug discovery is driven by the idea that binders Buch et al. constructed the binding process for zamidine to fi fi Twith higher binding af nity to a target should be more ef ca- trypsin and obtained accurate binding free energy with an error fi cious than those with lower binding af nity to the same target (1). of ∼1 kcal/mol compared with the experimental value, although fi It is obvious that the ef cacy of a drug is not only associated with both the predicated k and k deviate from the experimental thermodynamics but also related to the binding kinetics between on off fi values by at least an order of magnitude (23). Huang and the drug and a de ned target (2). Numerous examples demon- Caflisch investigated the spontaneous dissociation processes of strated that drug efficacy does not always linearly correlate with fi fi six small ligands from the active site of the FK506 binding pro- binding af nity (3). Therefore, an af nity-based drug discovery tein (24). However, the calculation results seem qualitative approach is less than complete, and an emerging paradigm em- rather than quantitative because their constructed BFEL is not phasizing both thermodynamics and kinetics of drug action has complete and accurate (24). Held et al. developed a computa- been widely recognized and appreciated in drug discovery (1). In tional method to analyze the ensemble of association pathways particular, ligand–receptor binding kinetics (BK), which have been and used this approach to study the binding of a phosphate ion to overlooked in traditional drug discovery approaches, are un- the Escherichia coli phosphate-binding protein (25). Moreover, precedentedly emphasized in almost all steps along the drug dis- the published results for kinetics simulations have not been covery and development pipeline (1, 4, 5). Indeed, a statistical concerned with pharmacologically important targets and ligands analysis on existing drugs demonstrated that the BK profile can be as complex as real drugs. On the other hand, all these predictions a key differentiator between different drugs (6). Drug-target res- need large-scale molecular dynamics simulations, which are too idence time or dissociative half-life (t = 0.693/k ) has become 1/2 off expensive to be applied in drug design. an important index in lead optimization (4). Thus, the BK-based paradigm will be promising in discovering better- or best-in-class drugs (1). Author contributions: F.B., X.W., J.M., H.L., J.N.O., and H.J. designed research; F.B., Y.X., J.C., Like the experimental approaches for drug discovery, the cur- Q.L., J.G., H.L., and H.J. performed research; F.B., H.L., and H.J. analyzed data; and F.B., J.M., rent computational drug design methods mainly emphasize bind- H.L., J.N.O., and H.J. wrote the paper. fi – ing af nity (7 11). Nevertheless, despite more than 30 y of effort, The authors declare no conflict of interest. predicting binding free energies for ligands interacting to targets 1To whom correspondence may be addressed. E-mail: [email protected], [email protected]. with sufficient accuracy is still an unsolved problem (12). The ac.cn, or [email protected]. fi — shortages of existing methods for predicting binding af nity fast This article contains supporting information online at www.pnas.org/lookup/suppl/doi:10. but inaccurate or accurate but slow—seem to be turning against the 1073/pnas.1301814110/-/DCSupplemental. www.pnas.org/cgi/doi/10.1073/pnas.1301814110 PNAS Early Edition | 1of6 Here, we present a computational method that could construct algorithm-based flexible docking method for binding configuration a complete free energy landscape for a drug binding to its target, sampling (details in SI Text). ΔGo from which the binding free energy ( binding) and activation free ΔG≠ ΔG≠ Binding Free Energy Calculation. Balancing speed and physical rig- energies for association ( on) and dissociation ( off) can be quantitatively estimated. We applied this method to simulate the orousness, we used a hybrid MM-GBSA method by combining binding process between (−)−Huperzine A (HupA), a marketed pair-wise–based and grid-based methods (28, 29) to calculate the natural product drug against Alzheimer’s disease (26), and its binding free energy. Furthermore, to enhance the calculation ac- target acetylcholinesterase (AChE) (27). The prediction is in ex- curacy, we modified the formulation of MM-GBSA (details in SI cellent agreement with the experimental result we performed in Text); i.e., the four energy terms of MM-GBSA were weighted by ΔGo fi ω –ω parallel in the present study. The predicted value of binding is four coef cients ( 1 4), respectively, very close to the experimental value, and the predicted values of ΔG≠ ΔG≠ ΔGo = ω ΔE + ω ΔE + ω ΔG + ω ΔG ; [1] on and off deviate from the experimental data only by 2.4 binding 1 vdw 2 es 3 gb 4 sa and 1.6 kJ/mol, respectively. Additionally, our method also pro- vided the atomic resolution information of the HupA binding where ΔEvdw, ΔEes, ΔGgb,andΔGsa are, respectively, the Lennard– pathway, possible binding transition states, and metastable states, Jones 6–12 potential, Coulombic potential, and polarization and which should be useful in designing more potent AChE inhibitors. nonpolarization components of salvation free energy. Weighting coefficients ω1–ω4 are obtained by fitting computational data with Results experimental data, using the multiple linear regression method Strategy for Construction of Binding Free Energy Landscape. The (details in SI Text). In the present study, the experimental binding results of this work are based on the analysis of computational and data of eight available AChE inhibitors with TcAChE were used experimental data for the binding process between HupA and to fit the values of ω1–ω4 (Table S1).
Details
-
File Typepdf
-
Upload Time-
-
Content LanguagesEnglish
-
Upload UserAnonymous/Not logged-in
-
File Pages6 Page
-
File Size-