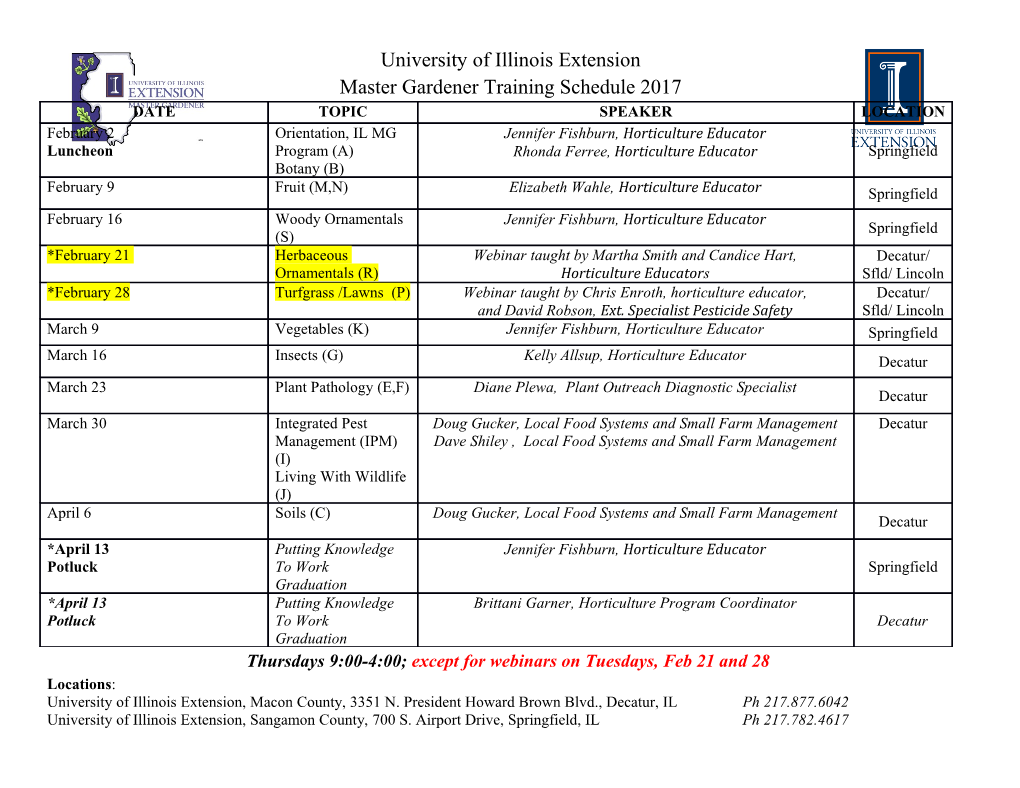
Simulating Urban Growth on the U.S.-Mexico Border: Nogales, Arizona and Nogales, Sonora Soe W. Myint, Jyoti Jan Christopher Lukinbeal, Francisco Lara-Valencia 2010 Working Paper Number 25 Simulating urban growth on the U.S.-Mexico border: Nogales, Arizona and Nogales, Sonora (Research Paper) (1) Soe W. Myint, (2) Jyoti Jain, (3) Christopher Lukinbeal, (4) Francisco Lara-Valencia All authors: School of Geographical Sciences and Urban Planning Arizona State University P.O. Box 875302 Tempe, AZ 85287-5302 Phone: (480) 965-7533 Fax: (480) 965-8313 Corresponding author - Email: [email protected]; Ph: (480) 965-6514; Fax: (480) 965- 8313 1 Abstract The paired U.S.-Mexico border cities of Nogales, Arizona and Nogales, Sonora, known as Ambos Nogales, are the largest and most rapidly growing cities on the Arizona-Sonora border. The growing urban population is producing extensive land-use and land-cover change in the region. The continued expansion of paired cities presents many environmental management and urban planning challenges. This research employs a cellular automata model to examine the difference between the patterns and rates of urban growth and land-use change under different environmental and planning strategies in the two cities over the next 20 years (2004-2025). A series of Landsat TM images acquired over different time periods (October 1985, July 1991, February 1995, September 2000, and July 2004) were used to simulate urban growth using four planning scenarios: business as usual; environmental protection; road network; and anti-growth strategy. The study revealed that urban growth trends in the first three scenarios simulated enormous edge growth throughout the region. The anti-growth scenario, which emphasizes environmental protection, is considered the most desirable for future urban development and planning in the Ambos Nogales region. Keywords: Nogales, planning scenarios, urban growth, Landsat 2 Introduction Urbanization is the general process of city growth and has often been viewed as a necessary component of regional economic growth; however, unplanned and mismanaged urban growth has provoked concerns over land-use and land-cover change such as the loss of large areas of primary forest and agricultural land, inadvertent climate repercussions, and environmental degradation (Yang, 2002). Li and Yeh (2000) stated that under current growth trends the complete depletion of agricultural land resources could occur in some fast growing areas. Land-use and land-cover change is one of the most significant forms of global environmental change and is felt in both developing and developed countries (Turner et al., 1993). Furthermore, land-use change has received growing attention alongside other human-induced environmental change, such as climate and atmosphere (Walker and Steffen, 1997 & 1999; Cihlar and Jansen, 2001). Urbanization alters the biophysical and socio-economic environment. Urban storm water runoff is a major contributing factor to water quality degradation (Driver and Troutman, 1989; U.S. EPA, 1997). Storm water changes hydrologic patterns, accelerates natural stream flows, destroys aquatic habitat, and elevates pollutant concentrations (Burton and Pitt, 2002). In addition, urbanization changes soil’s physical and chemical properties, water availability, vegetation, and associated animal and microbial communities (Jenerette and Wu, 2001). Urbanization poses other socio-economic challenges, such as demographic pressure, infrastructure problems, inadequate resources for service delivery, and planning (United Nations Human Settlement Programme, 2003). The inability to effectively manage these related challenges is rapidly increasing risks associated with poor housing conditions, uncollected solid waste, over consumption of 3 limited freshwater supplies, untreated waste water, and urban air pollution (Masser, 2001). Furthermore, complex interactions among physical, biological, economic, and social forces in both the spatial and temporal domain control urban growth and sprawl (Turner, 1987). Cities along the U.S.-Mexico border have grown at an unprecedented pace over the last few decades. The urban growth that began with the emergence of maquila industries and passage of the North American Free Trade Agreement (NAFTA) has resulted in drastic land-use and land-cover change across the region (Esparza et al., 2001). Land-use and land-cover change has been extensive in paired border cities which produces a plethora of urban problems and social pathologies (SCERP, 2005). Furthermore, rapidly growing border cities are placing strains on landscape to accommodate growth (Esparza et al., 2001). Researchers have studied various aspects of urban growth along the U.S.-Mexico border, such as air quality (US EPA, 2006), water quality (Reynolds, 2000), soil contamination, and hazardous waste (Guhathakurta et al., 2000). Social, cultural and quality of life issues along the border have also been discussed (SCERP, 2005). The paired U.S.-Mexico border cities of Nogales, Arizona and Nogales, Sonora, known as Ambos Nogales, are the most rapidly growing cities on the Arizona-Sonora border. Recently much research has been done on the Ambos Nogales watershed such as modeling land use change and the impact of water quality on urban growth and human health (Norman, 2005), Colonia development and settlement patterns (Norman et al., 2006), and modeling nonpoint source pollution (Norman, 2007; Norman et al., 2008). Continuous urban growth not only reduces the amount of open space, vegetation, and 4 forest areas but threatens the scenic, historic, and biological value of the Ambos Nogales region. Therefore, more research is needed that simulates the spatial consequences of urban growth and how it changes the landscape of U.S.-Mexico border cities. Traditional change detection methods can only provide a static diagnosis of changes that occur during fixed periods of time. However, urban growth and sprawl is a continuous and ongoing process that requires dynamic information that often goes beyond the temporally fixed coverage of remote sensing data. The most useful information for the decision-maker is not just what and where change occurs but why such change happens, at what pace, and what will the landscape look like if the driving factors continue under normal or alternative conditions (Weng, 2002). Answers to these questions rely on an effective change process model to predict the spatial distribution of the specific land-use and land-cover classes in future years by utilizing knowledge gained from previous years. Spatial transition-based models such as the Markov chain model (Muller and Middleton, 1994; Myint and Wang, 2006) and the Cellular Automata (CA) model (Clark, 1998) have been instrumental in predicting land-cover and land-use change. It may not be feasible to evaluate and compare the effectiveness of numerous land change models because they are completely different in many ways. For example, Pontius and Chen (2003) developed IDRISI’s GEOMOD that simulates change between two land categories (Pontius et al. 2001) whereas IDRISI’s cellular automata and Markov change (CA_MARKOV) simulates change among several land categories (Wagner 1997; Wu and Webster 1998; Pontius and Malanson 2005). Other models such as the dynamic geo-referenced land use/cover model (CLUE-CR) (Veldkamp and Fresco 1996) simulates 5 change in quantitative parameters as opposed to categorical variables. Some land change models are built in raster format, while others are in vector or grid format (Pontius and Chen, 2003). Pijanowski et al (2002) developed a land transformation model that employs artificial neural network (ANN) and GIS to predict development patterns within the six-county Grand Traverse Bay Watershed in Michigan. The study examined the relationship between several predictor variables and urbanization, and reported that the model performed with a relatively good predictive ability (46%) at a resolution of 100 m. Silva and Clarke (2001) employed the Slope, Land Cover, Exclusion, Urbanization, Transportation, and Hill-shade (SLEUTH) model (Clarke et al., 1997) to examine the differences in the model's behavior when different environmental variables of a European city are entered and modeled to predict future urban growth. Jantz et al (2010) developed methods that expand the capability of SLEUTH to incorporate economic, cultural, and policy information forecasts to 2030 of urban development under a current trends scenario across the entire Chesapeake Bay drainage basin. Herold et al. (2003) explored combining remote sensing, spatial metrics and spatial modeling using the SLEUTH model to analyze and model urban growth in Santa Barbara, California. It is widely accepted that there is no perfect landscape change model, but there are models that have been developed to achieve significantly different objectives (Baker, 1989; EPA, 2000; Pontius and Chen, 2003). The Environmental Protection Agency (2000) reviewed various landscape change models for assessing the effects of community growth and change on land-use patterns, and provides explicitly defined criteria to select the best fit land change model to project future land-use. The criteria to choose the right model include relevancy, availability of 6 resources (e.g., hardware, software), model support (e.g., model document, user discussion, training), technical expertise, data requirements, reliability and accuracy, data resolution, temporal capabilities, versatility (multiple variables), linkage potential,
Details
-
File Typepdf
-
Upload Time-
-
Content LanguagesEnglish
-
Upload UserAnonymous/Not logged-in
-
File Pages57 Page
-
File Size-