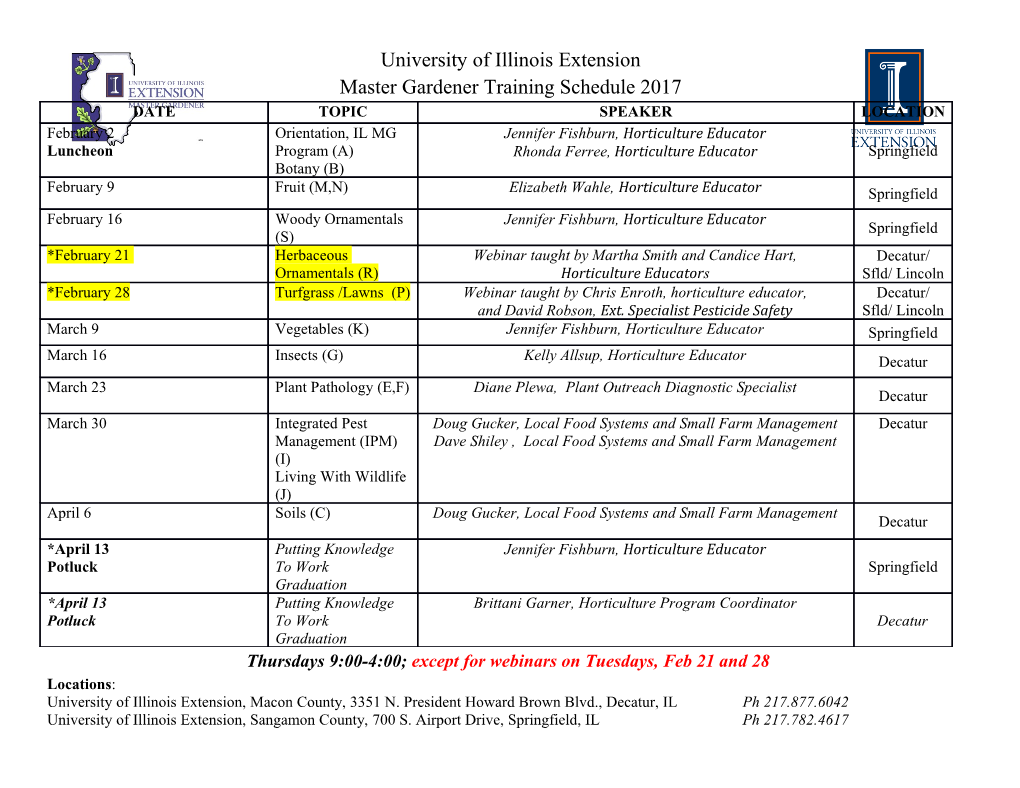
Machine Vision and Applications (2014) 25:1929–1951 DOI 10.1007/s00138-014-0596-3 SPECIAL ISSUE PAPER The ChaLearn gesture dataset (CGD 2011) Isabelle Guyon · Vassilis Athitsos · Pat Jangyodsuk · Hugo Jair Escalante Received: 31 January 2013 / Revised: 26 September 2013 / Accepted: 14 January 2014 / Published online: 21 February 2014 © Springer-Verlag Berlin Heidelberg 2014 Abstract This paper describes the data used in the collection software and the gesture vocabularies are down- ChaLearn gesture challenges that took place in 2011/2012, loadable from http://gesture.chalearn.org. We set up a forum whose results were discussed at the CVPR 2012 and for researchers working on these data http://groups.google. ICPR 2012 conferences. The task can be described as: com/group/gesturechallenge. user-dependent, small vocabulary, fixed camera, one-shot- learning. The data include 54,000 hand and arm gestures Keywords Computer vision · Gesture recognition · recorded with an RGB-D KinectTMcamera. The data are Sign language recognition · RGBD cameras · Kinect · organized into batches of 100 gestures pertaining to a small Dataset · Challenge · Machine learning · Transfer learning · gesture vocabulary of 8–12 gestures, recorded by the same One-shot-learning user. Short continuous sequences of 1–5 randomly selected gestures are recorded. We provide man-made annotations Mathematics Subject Classification (2000) 65D19 · (temporal segmentation into individual gestures, alignment 68T10 · 97K80 of RGB and depth images, and body part location) and a library of function to preprocess and automatically anno- tate data. We also provide a subset of batches in which the 1 Introduction user’s horizontal position is randomly shifted or scaled. We report on the results of the challenge and distribute sample 1.1 Motivations code to facilitate developing new solutions. The data, data Machine learning has been successfully applied to computer vision problems in the recent year, and particularly to the visual analysis of humans [36]. Some of the main advances This effort was initiated by the DARPA Deep Learning program and have been catalyzed by important efforts in data annota- was supported by the US National Science Foundation (NSF) under tion [2,15,43], and the organization of challenges such as grants ECCS 1128436 and ECCS 1128296, the EU Pascal2 network image CLEF [3] and the Visual Object Classes Challenges of excellence and the Challenges in Machine Learning foundation. Any opinions, findings, and conclusions or recommendations (VOC) [4], progressively introducing more difficult tasks in expressed in this material are those of the authors and do not image classification, 2D camera video processing and, since necessarily reflect the views of the funding agencies. recently, 3D camera video processing. In 2011/2012, we B organized a challenge focusing on the recognition of gestures I. Guyon ( ) from video data recorded with a Microsoft KinectTMcamera, ChaLearn, 955 Creston Road, Berkeley, CA 94708-1501, USA e-mail: [email protected] which provides both an RGB (red/blue/green) image and a depth image obtained with an infrared sensor (Fig. 1). V. Athitsos · P. Jangyodsuk KinectTMhas revolutionized computer vision in the past few University of Texas at Arlington, Arlington, TX, USA months by providing one of the first affordable 3D cameras. H. J. Escalante The applications, initially driven by the game industry, are INAOE, Puebla, Mexico rapidly diversifying. They include: 123 1930 I. Guyon et al. Fig. 1 Videos recorded with Kinect. Users performed gestures in front of a fixed KinectTMcamera, which recorded both a regular RGB image and a depth image (rendered as gray levels here) Sign language recognition Enable deaf people to commu- tions such as spatial and temporal resolution and unreliable nicate with machines and hearing people to get translations. depth cues. Technical difficulties include tracking reliably This may be particularly useful for deaf children of hearing hand, head and body parts, and achieving 3D invariance. On parents. the machine-learning side, much of the recent research has Remote control At home, replace your TV remote con- sacrificed the grand goal of designing systems ever approach- troller by a gesture enabled controller, which you cannot ing human intelligence for solving tasks of practical interest lose and works fine in the dark; turn on your light switch with more immediate reward. Humans can recognize new at night; allow hospital patients to control appliances and gestures after seeing just one example (one-shot-learning). call for help without buttons; allow surgeons and other pro- With computers though, recognizing even well-defined ges- fessional users to control appliances and image displays in tures, such as sign language, is much more challenging and sterile conditions without touching. has traditionally required thousands of training examples to Games Replace your joy stick with your bare hands. Teach teach the software. your computer the gestures you prefer to control your games. One of our goals was to evaluate transfer learning algo- Play games of skills, reflexes and memory, which require rithms that exploit data resources from similar but dif- learning new gestures and/or teaching them to your com- ferent tasks (e.g., a different vocabulary of gestures) to puter. improve performance on a given task for which training Gesture communication learning Learn new gesture data are scarce (e.g., only a few labeled training examples vocabularies from the sign language for the deaf, from pro- of each gesture are available). Using a one-shot-learning set- fessional signal vocabularies (referee signals, marshaling ting (only one labeled training example of each gesture is signals, diving signals, etc.), and from subconscious body available) pushes the need for exploiting unlabeled data or language. data that are labeled, but pertain to a similar but different Video surveillance Teach surveillance systems to watch for vocabularies of gestures. While the one-shot-learning set- particular types of gestures (shop-lifting gestures, aggres- ting has implications of academic interest, it has also prac- sions, etc.) tical ramifications. Every application needs a specialized Video retrieval Look for videos containing a gesture that gesture vocabulary. The availability of gesture recognition you perform in front of a camera. This includes dictionary machines, which easily get tailored to new gesture vocabu- lookup in databases of videos of sign language for the deaf. laries, would facilitate tremendously the task of application developers. Gesture recognition provides excellent benchmarks for Another goal was to explore the capabilities of algorithms computer vision and machine-learning algorithms. On the to perform data fusion (in this case fuse the RGB and depth computer vision side, the recognition of continuous, nat- modalities). Yet another goal was to force the participants to ural gestures is very challenging due to the multi-modal develop new methods of feature extraction from raw video nature of the visual cues (e.g., movements of fingers and lips, data (as opposed, for instance, to work from extracted skele- facial expressions, body pose), as well as technical limita- ton coordinates). 123 The ChaLearn gesture dataset 1931 Beyond the goal of benchmarking algorithms of ges- development phase (December 7, 2011 to April 6, 2012) and ture recognition, our dataset offers possibilities to conduct a final evaluation phase (April 7, 2012 to April 10. 2012): research on gesture analysis from the semiotic, linguistic, During the development phase of the competition, the par- sociological, psychological, and aesthetic points of view. We ticipants built a learning system capable of learning from a have made an effort to select a wide variety of gesture vocab- single training example a gesture classification problem. To ularies and proposed a new classification of gesture types, that end, they obtained development data consisting of sev- which is more encompassing than previously proposed clas- eral batches of gestures, each split into a training set (of one sifications [28,34]. example for each gesture) and a test set of short sequences Our new gesture database complements other publicly of one to five gestures. Each batch contained gestures from available datasets in several ways. It complements sign lan- a different small vocabulary of 8–12 gestures, for instance guage recognition datasets (e.g., [16,33]) by providing access diving signals, signs of American Sign Language represent- to a wider variety of gesture types. It focuses on upper ing small animals, Italian gestures, etc. The test data labels body movement of users facing a camera, unlike datasets were provided for the development data, so the participants featuring full-body movements [22], poses [42] or activi- can self-evaluate their systems. To evaluate their progress ties [14,27,29,37]. As such, it provides an unprecedentedly and compare themselves with others, they could use valida- large set of signs performed with arms and hand suitable tion data, for which one training example was provided for to design man–machine interfaces, complementing datasets each gesture token in each batch, but the test data labels were geared towards shape and motion estimation [5,22] or object withheld. Kaggle provided a website to which the prediction manipulation [31]. The spacial resolution of Kinect limits results could be submitted.1 A real-time leaderboard showed the use of the depth information
Details
-
File Typepdf
-
Upload Time-
-
Content LanguagesEnglish
-
Upload UserAnonymous/Not logged-in
-
File Pages23 Page
-
File Size-