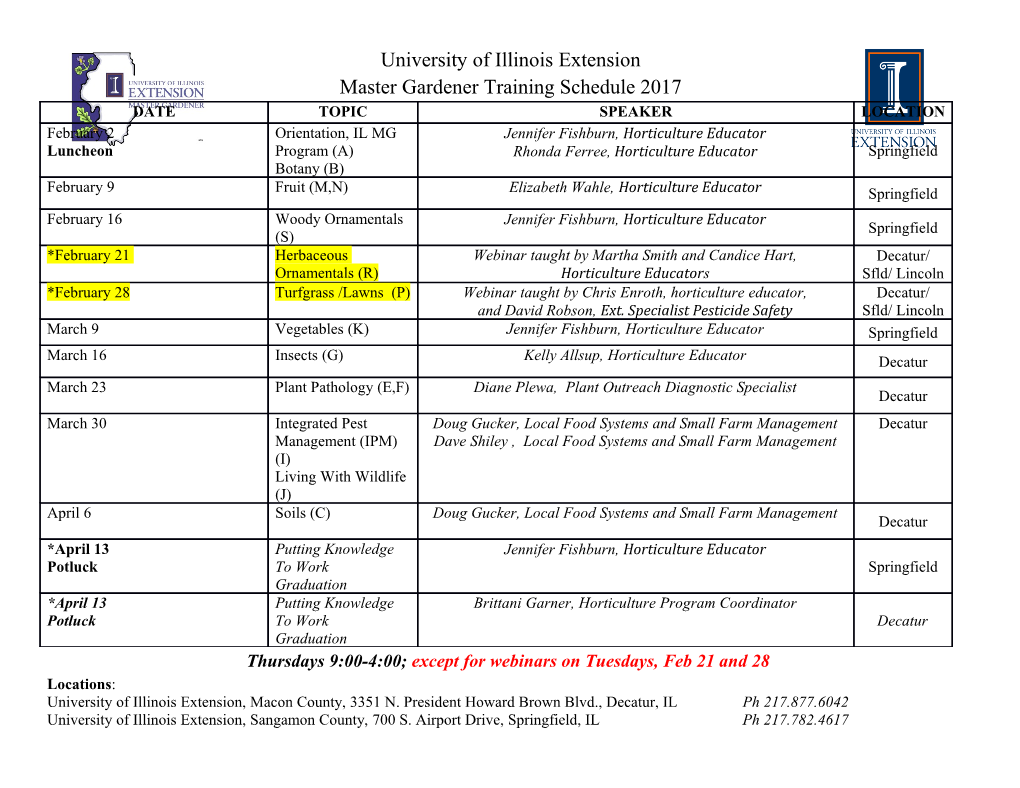
Bayesian inference and Bayesian model selection Klaas Enno Stephan Lecture as part of "Methods & Models for fMRI data analysis", University of Zurich & ETH Zurich, 28 November 2017 With slides from and many thanks to: Kay Brodersen, Will Penny, Sudhir Shankar Raman Why should I know about Bayesian inference? Because Bayesian principles are fundamental for • statistical inference in general • system identification • translational neuromodeling ("computational assays") – computational psychiatry – computational neurology • contemporary theories of brain function (the "Bayesian brain") – predictive coding – free energy principle – active inference Why should I know about Bayesian inference? Because Bayesian principles are fundamental for • statistical inference in general • system identification • translational neuromodeling ("computational assays") – computational psychiatry – computational neurology • contemporary theories of brain function (the "Bayesian brain") – predictive coding – free energy principle – active inference Bayes' theorem The Reverend Thomas Bayes (1702-1761) pp(y |θθ ) ( ) “Bayes‘ Theorem p(θy | ) describes, how an p()y ideally rational person processes information." posterior = likelihood ∙ prior / evidence Wikipedia Bayes’ Theorem Given data y and parameters , the joint probability is: p(,) y p (|)() y p y p (|)() y p Eliminating p(y,) gives Bayes’ rule: posterior likelihood prior p( y | ) p ( ) Py( | ) py() evidence Bayesian inference: an animation Generative models • specify a joint probability distribution over all variables (observations and parameters) • require a likelihood function and a prior: py(,|) m py (|,)(|) mp m p (|,) ym • can be used to randomly generate synthetic data (observations) by sampling from the prior – we can check in advance whether the model can explain certain phenomena at all • model comparison based on the model evidence p( y | m ) p ( y | , m ) p ( | m ) d Principles of Bayesian inference Formulation of a generative model likelihood function p(y|) Model prior distribution p() Observation of data Measurement data y Model inversion – updating one's beliefs maximum a posteriori p( | y ) p ( y | ) p ( ) (MAP) estimates model evidence Example of a shrinkage prior Priors Priors can be of different sorts, e.g. • empirical (previous data) • empirical (estimated from current data using a hierarchical model → "empirical Bayes") • uninformed • principled (e.g., positivity constraints) • shrinkage Generative models p( y | , m )pm( | ) p( | y , m ) 1. enforce mechanistic thinking: how could the data have been caused? 2. generate synthetic data (observations) by sampling from the prior – can model explain certain phenomena at all? 3. inference about parameters → p(|y) 4. inference about model structure → p(y|m) or p(m|y) 5. model evidence: index of model quality A generative modelling framework for fMRI & EEG: Dynamic causal modeling (DCM) dwMRI EEG, MEG fMRI Forward model: Model inversion: Predicting measured Estimating neuronal activity mechanisms dx y g(x,) f(,,) x u dt Friston et al. 2003, NeuroImage Stephan et al. 2009, NeuroImage DCM for fMRI Stephan et al. 2015, Neuron Bayesian system identification u(t) Design experimental inputs Neural dynamics dx dt f(,,) x u Define likelihood model Observer function y g(,) x p(y |,m) N(g( ),( )) Specify priors p(,m) N( , ) Inference on model p(y | m) p(y |,m) p( )d Invert model structure p(y |,m) p(,m) Inference on parameters p( | y,m) p(y | m) Make inferences Why should I know about Bayesian inference? Because Bayesian principles are fundamental for • statistical inference in general • system identification • translational neuromodeling ("computational assays") – computational psychiatry – computational neurology • contemporary theories of brain function (the "Bayesian brain") – predictive coding – free energy principle – active inference Generative models as "computational assays" p( y | , m )pm( | ) p( | y , m ) Computational assays: Models of disease mechanisms Translational Neuromodeling dx f(,,) x u dt Individual treatment prediction Detecting physiological subgroups (based on inferred mechanisms) disease mechanism A Application to brain activity and disease mechanism B behaviour of individual patients disease mechanism C Stephan et al. 2015, Neuron Differential diagnosis based on generative models of disease symptoms SYMPTOM y (behaviour or physiology) p( y | , mk ) p( mk | y ) HYPOTHETICAL ... ... MECHANISM m1 mk mK p( y | mkk ) p ( m ) pm(k | y) p( y | mkk ) p ( m ) k Stephan et al. 2016, NeuroImage Why should I know about Bayesian inference? Because Bayesian principles are fundamental for • statistical inference in general • system identification • translational neuromodeling ("computational assays") – computational psychiatry – computational neurology • contemporary theories of brain function (the "Bayesian brain") – predictive coding – free energy principle – active inference Perception = inversion of a hierarchical generative model environm. states neuronal states others' mental states bodily states forward model p( y | x , m ) p ( x | m ) p( x | y , m ) perception Example: free-energy principle and active inference sensations – predictions Prediction error Change Change sensory input predictions Action Perception Maximizing the evidence (of the brain's generative model) Friston et al. 2006, = minimizing the surprise about the data (sensory inputs). J Physiol Paris How is the posterior computed = how is a generative model inverted? Bayesian Inference Analytical solutions Approximate Inference Variational MCMC Bayes Sampling How is the posterior computed = how is a generative model inverted? • compute the posterior analytically – requires conjugate priors – even then often difficult to derive an analytical solution • variational Bayes (VB) – often hard work to derive, but fast to compute – cave: local minima, potentially inaccurate approximations • sampling methods (MCMC) – guaranteed to be accurate in theory (for infinite computation time) – but may require very long run time in practice – convergence difficult to prove Conjugate priors If the posterior p(θ|x) is in the same family as the prior p(θ), the prior and posterior are called "conjugate distributions", and the prior is called a "conjugate prior" for the likelihood function. p(y | ) p( ) p( | y) p(y) same form analytical expression for posterior examples (likelihood-prior): • Normal-Normal • Normal-inverse Gamma • Binomial-Beta • Multinomial-Dirichlet Posterior mean & variance of univariate Gaussians Likelihood & Prior 2 y p( y | ) N ( , e ) pN()(,) 2 pp Posterior: p( | y ) N ( , 2 ) Posterior 1 1 1 Likelihood 2 2 2 e p p Prior 1 1 2 2 2 p e p Posterior mean = variance-weighted combination of prior mean and data mean Same thing – but expressed as precision weighting Likelihood & prior 1 y p( y | ) N ( , e ) pN()(,) 1 pp Posterior: p( | y ) N ( , 1 ) Posterior Likelihood e p p Prior e p p Relative precision weighting Variational Bayes (VB) Idea: find an approximate density 푞(휃) that is maximally similar to the true posterior 푝 휃 푦 . This is often done by assuming a particular form for 푞 (fixed form VB) and then optimizing its sufficient statistics. true hypothesis posterior class 푝 휃 푦 divergence KL 푞||푝 best proxy 푞 휃 Kullback–Leibler (KL) divergence • asymmetric measure of the difference between two probability distributions P and Q • Interpretations of DKL(P‖Q): – "Bayesian surprise" when Q=prior, P=posterior: measure of the information gained when one updates one's prior beliefs to the posterior P – a measure of the information lost when Q is used to approximate P • non-negative: 0 (zero when P=Q) Variational calculus Standard calculus Variational Newton, Leibniz, and calculus others Euler, Lagrange, and others • functions 푓: 푥 ↦ 푓 푥 • functionals Leonhard Euler d푓 퐹: 푓 ↦ 퐹 푓 (1707 – 1783) • derivatives Swiss mathematician, d푥 d퐹 • derivatives ‘Elementa Calculi d푓 Variationum’ Example: maximize the likelihood Example: maximize expression 푝 푦 휃 the entropy 퐻 푝 w.r.t. 휃 w.r.t. a probability distribution 푝 푥 Variational Bayes ln 푝 푦 ∗ ln 푝 푦 ln 푝(푦) = KL[푞| 푝 + 퐹 푞, 푦 KL[푞| 푝 divergence neg. free 퐹 푞, 푦 ≥ 0 energy KL[푞| 푝 (unknown) (easy to evaluate for a given 푞) 퐹 푞 is a functional wrt. the 퐹 푞, 푦 approximate posterior 푞 휃 . Maximizing 퐹 푞, 푦 is equivalent to: • minimizing KL[푞| 푝 • tightening 퐹 푞, 푦 as a lower bound to the log model evidence initialization … When 퐹 푞, 푦 is maximized, 푞 휃 is … convergence our best estimate of the posterior. Derivation of the (negative) free energy approximation • See whiteboard! • (or Appendix to Stephan et al. 2007, NeuroImage 38: 387-401) Mean field assumption Factorize the approximate posterior 푞 휃 into independent 푞 휃1 푞 휃 partitions: 2 푞 휃 = ෑ 푞푖 휃푖 푖 where 푞푖 휃푖 is the approximate posterior for the 푖th subset of parameters. For example, split parameters and hyperparameters: 휃2 휃1 Jean Daunizeau, www.fil.ion.ucl.ac.uk/ p, | y q , q q ~jdaunize/presentations/Bayes2.pdf VB in a nutshell (under mean-field approximation) Neg. free-energy lnp y | m F KL q , , p , | y approx. to model evidence. Fln p y | , KL q , , p , | m q Mean field approx. Maximise neg. free q exp I exp ln p y , , energy wrt. q = q() minimise divergence, q exp I exp ln p y , , by maximising q() variational energies pIterative, | y updating q , of sufficient q q statistics of approx. posteriors by gradient ascent. VB (under mean-field
Details
-
File Typepdf
-
Upload Time-
-
Content LanguagesEnglish
-
Upload UserAnonymous/Not logged-in
-
File Pages59 Page
-
File Size-