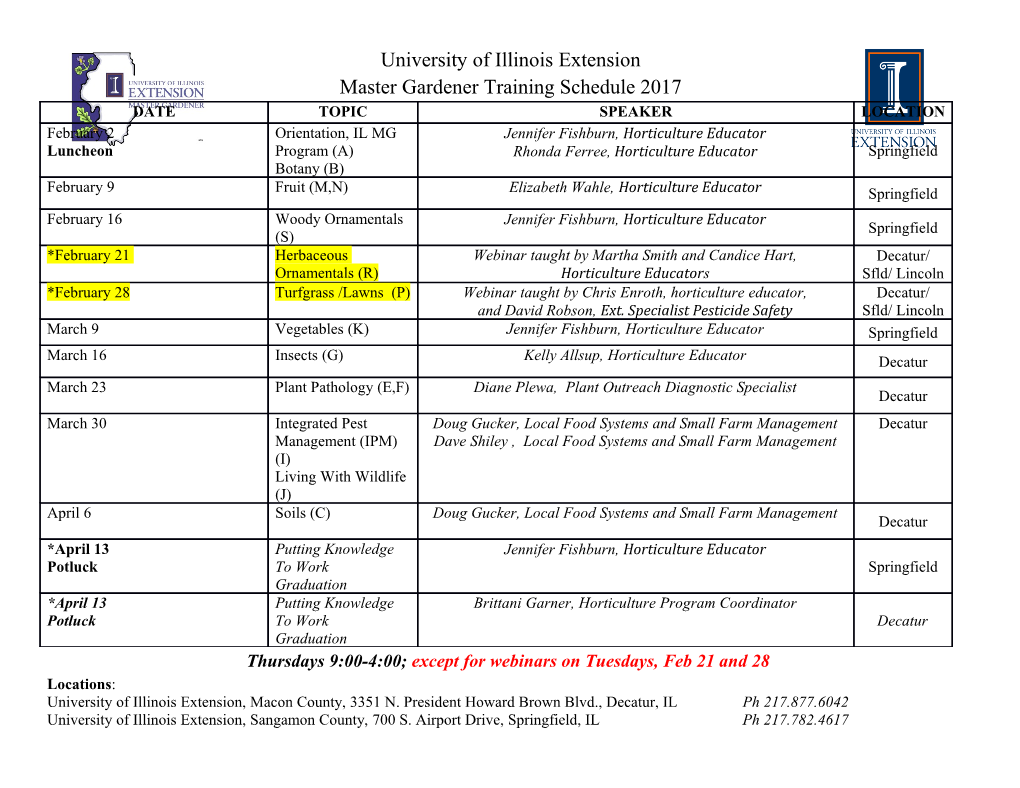
18.06.16: The four fundamental subspaces Lecturer: Barwick Monday 14 March 2016 18.06.16: The four fundamental subspaces Here it is again: Theorem (Rank-Nullity Theorem). If 퐴 is an 푚 × 푛 matrix, then dim(ker(퐴)) + dim(im(퐴)) = 푛. We saw a proof of this by reducing to rref or rcef, and then checking it there. There’s just one thing that might bug us here. If I think of the linear map 푛 푚 푇퐴∶ R R , then we see that ker(퐴) is a subspace of the source R푛, but im(퐴) is a subspace of the target R푚. So why should these spaces be related? 18.06.16: The four fundamental subspaces To answer this question, there’s another matrix we can contemplate, the trans- pose 퐴⊺. This is an 푛 × 푚 matrix, and so it corresponds to a linear map in the other direction: 푚 푛 푇퐴⊺ ∶ R R . This is the map that takes a column vector ~푣 and builds the column vector 퐴⊺~푣, but we can perform a trick here. Instead of thinking about transposing 퐴, we can think about transposing the vectors. 18.06.16: The four fundamental subspaces So (R푚)∨ will be the set of all 푚-dimensional row vectors; equivalently, the set of all 1 × 푚 matrices; equivalently again, the set of all transposes 푣 ≔ (~푣)⊺ ~ of vectors ~푣 ∈ R푚. We call (R푚)∨ the dual R푚. 1 0 So, e.g., if ~푣 = ( −2 ), then 푣 = ( 1 0 −2 1 0 ). ~ 1 ( 0 ) 18.06.16: The four fundamental subspaces The neat thing about row vectors is that they do stuff to column vectors. If ~푣 ∈ R푛 and 푤 ∈ (R푛)∨, then 푤~푣 is a number. (Question: How is this related to ~ ~ the dot product?) If 푉 ⊆ R푛 is a vector subspace, then we define 푉⟂ ≔ {푤 ∈ (R푛)∨ | for any ~푣 ∈ 푉, 푤~푣 = 0} ⊆ (R푛)∨. ~ ~ Dually, if 푊 ⊆ (R푛)∨ is a vector subspace, then we define 푊⟂ ≔ {~푣 ∈ R푛 | for any 푤 ∈ 푊, 푤~푣 = 0} ⊆ R푛. ~ ~ Fact: dim(푉) = 푛 − dim(푉⟂), and dim(푊) = 푛 − dim(푊⟂). (Why?) 18.06.16: The four fundamental subspaces Now, since (퐴⊺~푣)⊺ = (~푣)⊺퐴 = 푣퐴, ~ we can leave 퐴 just as it is, and we can consider the linear map ∨ 푚 ∨ 푛 ∨ 푇퐴 ∶ (R ) (R ) given by the formula 푇∨(푣) ≔ 푣퐴. 퐴 ~ ~ 18.06.16: The four fundamental subspaces So when we contemplate the kernel and image of 퐴⊺, we can think about it ∨ via the map 푇퐴 . For example, ker(퐴⊺) is the set of all vectors 푣 ∈ (R푛)∨ such that 푣퐴 = 0. This ~ ~ ~ space is also called the cokernel or the left kernel of 퐴. I write coker(퐴). We also have the image of 퐴⊺, which is the set of all row vectors 푤 ∈ (R푛)∨ ~ such that there exists a row vector 푣 ∈ (R푚)∨ for which 푤 = 푣퐴. This space is ~ ~ ~ also called the coimage of 퐴, or, since its the span of the columns of 퐴⊺, which is the span of the rows of 퐴, it is also called the row space of 퐴. I write coim(퐴). 18.06.16: The four fundamental subspaces In all, we have four vector spaces that are what Strang call the fundamental subspaces attached to 퐴: ker(퐴), im(퐴), coker(퐴) ≔ ker(퐴⊺), coim(퐴) ≔ im(퐴⊺). 18.06.16: The four fundamental subspaces Here’s the abstract statement of the Rank-Nullity Theorem: (1) ker(퐴) = coim(퐴)⟂, so that dim(ker(퐴)) = 푛 − dim(coim(퐴)). (2) im(퐴) = coker(퐴)⟂, so that dim(im(퐴)) = 푚 − dim(coker(퐴)). (3) 퐴 provides a bijection coim(퐴) ≅ im(퐴), so that dim(coim(퐴)) = dim(im(퐴))..
Details
-
File Typepdf
-
Upload Time-
-
Content LanguagesEnglish
-
Upload UserAnonymous/Not logged-in
-
File Pages9 Page
-
File Size-