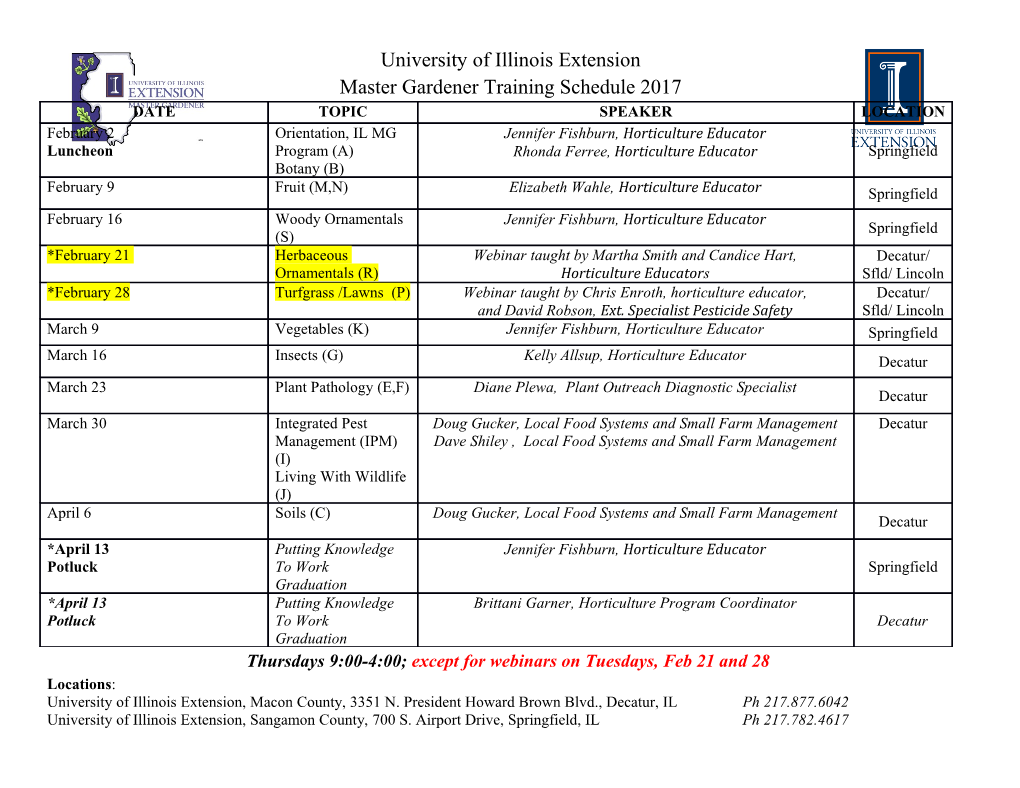
Lecture 3 Feedforward Networks and Backpropagation CMSC 35246: Deep Learning Shubhendu Trivedi & Risi Kondor University of Chicago April 3, 2017 Lecture 3 Feedforward Networks and Backpropagation CMSC 35246 • Going from one neuron to Feedforward Networks • Example: Learning XOR • Cost Functions, Hidden unit types, output types • Universality Results and Architectural Considerations • Backpropagation Things we will look at today • Recap of Logistic Regression Lecture 3 Feedforward Networks and Backpropagation CMSC 35246 • Example: Learning XOR • Cost Functions, Hidden unit types, output types • Universality Results and Architectural Considerations • Backpropagation Things we will look at today • Recap of Logistic Regression • Going from one neuron to Feedforward Networks Lecture 3 Feedforward Networks and Backpropagation CMSC 35246 • Cost Functions, Hidden unit types, output types • Universality Results and Architectural Considerations • Backpropagation Things we will look at today • Recap of Logistic Regression • Going from one neuron to Feedforward Networks • Example: Learning XOR Lecture 3 Feedforward Networks and Backpropagation CMSC 35246 • Universality Results and Architectural Considerations • Backpropagation Things we will look at today • Recap of Logistic Regression • Going from one neuron to Feedforward Networks • Example: Learning XOR • Cost Functions, Hidden unit types, output types Lecture 3 Feedforward Networks and Backpropagation CMSC 35246 • Backpropagation Things we will look at today • Recap of Logistic Regression • Going from one neuron to Feedforward Networks • Example: Learning XOR • Cost Functions, Hidden unit types, output types • Universality Results and Architectural Considerations Lecture 3 Feedforward Networks and Backpropagation CMSC 35246 Things we will look at today • Recap of Logistic Regression • Going from one neuron to Feedforward Networks • Example: Learning XOR • Cost Functions, Hidden unit types, output types • Universality Results and Architectural Considerations • Backpropagation Lecture 3 Feedforward Networks and Backpropagation CMSC 35246 Recap: The Logistic Function (Single Neuron) y^ 1 θ0 θd θ1 θ2 θ3 x1 x2 x3 ... xd 1 p(y = 1jx) = T 1 + exp(−θ0 − θ x) Lecture 3 Feedforward Networks and Backpropagation CMSC 35246 T yi T 1−yi p(yijxi; θ) = σ(θ0 + θ xi) (1 − σ(θ0 + θ xi)) The log-likelihood of θ (cross-entropy!): N X log p(Y jX; θ) = log p(yijxi; θ) i=1 N X T T = yi log σ(θ0 + θ xi) + (1 − yi) log(1 − σ(θ0 + θ xi)) i=1 Likelihood under the Logistic Model ( σ(θ + θT x ) if y = 1 p(y jx; θ) = 0 i i i T 1 − σ(θ0 + θ xi) if yi = 0 We can rewrite this as: Lecture 3 Feedforward Networks and Backpropagation CMSC 35246 The log-likelihood of θ (cross-entropy!): N X log p(Y jX; θ) = log p(yijxi; θ) i=1 N X T T = yi log σ(θ0 + θ xi) + (1 − yi) log(1 − σ(θ0 + θ xi)) i=1 Likelihood under the Logistic Model ( σ(θ + θT x ) if y = 1 p(y jx; θ) = 0 i i i T 1 − σ(θ0 + θ xi) if yi = 0 We can rewrite this as: T yi T 1−yi p(yijxi; θ) = σ(θ0 + θ xi) (1 − σ(θ0 + θ xi)) Lecture 3 Feedforward Networks and Backpropagation CMSC 35246 Likelihood under the Logistic Model ( σ(θ + θT x ) if y = 1 p(y jx; θ) = 0 i i i T 1 − σ(θ0 + θ xi) if yi = 0 We can rewrite this as: T yi T 1−yi p(yijxi; θ) = σ(θ0 + θ xi) (1 − σ(θ0 + θ xi)) The log-likelihood of θ (cross-entropy!): N X log p(Y jX; θ) = log p(yijxi; θ) i=1 N X T T = yi log σ(θ0 + θ xi) + (1 − yi) log(1 − σ(θ0 + θ xi)) i=1 Lecture 3 Feedforward Networks and Backpropagation CMSC 35246 Setting derivatives to zero: N @ log p(Y jX; θ) X = (y − σ(θ + θT x )) = 0 @θ i 0 i 0 i=1 N @ log p(Y jX; θ) X = (y − σ(θ + θT x ))x = 0 @θ i 0 i i;j j i=1 T Can treat yi − p(yijxi) = yi − σ(θ0 + θ xi) as the prediction error The Maximum Likelihood Solution N X T T log p(Y jX; θ) = yi log σ(θ0+θ xi)+(1−yi) log(1−σ(θ0+θ xi)) i=1 Lecture 3 Feedforward Networks and Backpropagation CMSC 35246 N @ log p(Y jX; θ) X = (y − σ(θ + θT x )) = 0 @θ i 0 i 0 i=1 N @ log p(Y jX; θ) X = (y − σ(θ + θT x ))x = 0 @θ i 0 i i;j j i=1 T Can treat yi − p(yijxi) = yi − σ(θ0 + θ xi) as the prediction error The Maximum Likelihood Solution N X T T log p(Y jX; θ) = yi log σ(θ0+θ xi)+(1−yi) log(1−σ(θ0+θ xi)) i=1 Setting derivatives to zero: Lecture 3 Feedforward Networks and Backpropagation CMSC 35246 T Can treat yi − p(yijxi) = yi − σ(θ0 + θ xi) as the prediction error The Maximum Likelihood Solution N X T T log p(Y jX; θ) = yi log σ(θ0+θ xi)+(1−yi) log(1−σ(θ0+θ xi)) i=1 Setting derivatives to zero: N @ log p(Y jX; θ) X = (y − σ(θ + θT x )) = 0 @θ i 0 i 0 i=1 N @ log p(Y jX; θ) X = (y − σ(θ + θT x ))x = 0 @θ i 0 i i;j j i=1 Lecture 3 Feedforward Networks and Backpropagation CMSC 35246 The Maximum Likelihood Solution N X T T log p(Y jX; θ) = yi log σ(θ0+θ xi)+(1−yi) log(1−σ(θ0+θ xi)) i=1 Setting derivatives to zero: N @ log p(Y jX; θ) X = (y − σ(θ + θT x )) = 0 @θ i 0 i 0 i=1 N @ log p(Y jX; θ) X = (y − σ(θ + θT x ))x = 0 @θ i 0 i i;j j i=1 T Can treat yi − p(yijxi) = yi − σ(θ0 + θ xi) as the prediction error Lecture 3 Feedforward Networks and Backpropagation CMSC 35246 But log p(Y jX; x) is jointly concave in all components of θ Or, equivalently, the error is convex Gradient Descent/ascent (descent on − log p(yjx; θ), log loss) Finding Maxima No closed form solution for the Maximum Likelihood for this model! Lecture 3 Feedforward Networks and Backpropagation CMSC 35246 Or, equivalently, the error is convex Gradient Descent/ascent (descent on − log p(yjx; θ), log loss) Finding Maxima No closed form solution for the Maximum Likelihood for this model! But log p(Y jX; x) is jointly concave in all components of θ Lecture 3 Feedforward Networks and Backpropagation CMSC 35246 Gradient Descent/ascent (descent on − log p(yjx; θ), log loss) Finding Maxima No closed form solution for the Maximum Likelihood for this model! But log p(Y jX; x) is jointly concave in all components of θ Or, equivalently, the error is convex Lecture 3 Feedforward Networks and Backpropagation CMSC 35246 Finding Maxima No closed form solution for the Maximum Likelihood for this model! But log p(Y jX; x) is jointly concave in all components of θ Or, equivalently, the error is convex Gradient Descent/ascent (descent on − log p(yjx; θ), log loss) Lecture 3 Feedforward Networks and Backpropagation CMSC 35246 N 1 X − log p(y jx ; θ) N i i i=1 Gradient update: ηt @ X θ(t+1) := θt + log p(y jx ; θ(t)) N @θ i i i Gradient on one example: @ log p(y jx ; θ) = (y − σ(θT x ))x @θ i i i i i Above is batch gradient descent Gradient Descent Solution Objective is the average log-loss Lecture 3 Feedforward Networks and Backpropagation CMSC 35246 Gradient update: ηt @ X θ(t+1) := θt + log p(y jx ; θ(t)) N @θ i i i Gradient on one example: @ log p(y jx ; θ) = (y − σ(θT x ))x @θ i i i i i Above is batch gradient descent Gradient Descent Solution Objective is the average log-loss N 1 X − log p(y jx ; θ) N i i i=1 Lecture 3 Feedforward Networks and Backpropagation CMSC 35246 Gradient on one example: @ log p(y jx ; θ) = (y − σ(θT x ))x @θ i i i i i Above is batch gradient descent Gradient Descent Solution Objective is the average log-loss N 1 X − log p(y jx ; θ) N i i i=1 Gradient update: ηt @ X θ(t+1) := θt + log p(y jx ; θ(t)) N @θ i i i Lecture 3 Feedforward Networks and Backpropagation CMSC 35246 Above is batch gradient descent Gradient Descent Solution Objective is the average log-loss N 1 X − log p(y jx ; θ) N i i i=1 Gradient update: ηt @ X θ(t+1) := θt + log p(y jx ; θ(t)) N @θ i i i Gradient on one example: @ log p(y jx ; θ) = (y − σ(θT x ))x @θ i i i i i Lecture 3 Feedforward Networks and Backpropagation CMSC 35246 Gradient Descent Solution Objective is the average log-loss N 1 X − log p(y jx ; θ) N i i i=1 Gradient update: ηt @ X θ(t+1) := θt + log p(y jx ; θ(t)) N @θ i i i Gradient on one example: @ log p(y jx ; θ) = (y − σ(θT x ))x @θ i i i i i Above is batch gradient descent Lecture 3 Feedforward Networks and Backpropagation CMSC 35246 Feedforward Networks Lecture 3 Feedforward Networks and Backpropagation CMSC 35246 Ideal classifier: y = f ∗(x) with x and category y Feedforward Network: Define parametric mapping y = f(x; θ) Learn parameters θ to get a good approximation to f ∗ from available sample Naming: Information flow in function evaluation begins at input, flows through intermediate computations (that define the function), to produce the category No feedback connections (Recurrent Networks!) Introduction Goal: Approximate some unknown ideal function f ∗ : X!Y Lecture 3 Feedforward Networks and Backpropagation CMSC 35246 Feedforward Network: Define parametric mapping y = f(x; θ) Learn parameters θ to get a good approximation to f ∗ from available sample Naming: Information flow in function evaluation begins at input, flows through intermediate computations (that define the function), to produce the category No feedback connections (Recurrent Networks!) Introduction Goal: Approximate some unknown ideal function f ∗ : X!Y Ideal classifier: y = f ∗(x) with x and category y Lecture 3 Feedforward Networks and Backpropagation CMSC 35246 Learn parameters θ to get a good approximation to f ∗ from available sample Naming: Information flow in function evaluation begins at input, flows through intermediate computations (that define the function), to produce the category No feedback connections (Recurrent Networks!) Introduction Goal: Approximate some unknown ideal function f ∗ : X!Y Ideal classifier: y = f ∗(x) with x and category y Feedforward Network: Define parametric mapping y = f(x; θ) Lecture 3 Feedforward Networks and Backpropagation CMSC 35246 Naming: Information
Details
-
File Typepdf
-
Upload Time-
-
Content LanguagesEnglish
-
Upload UserAnonymous/Not logged-in
-
File Pages241 Page
-
File Size-