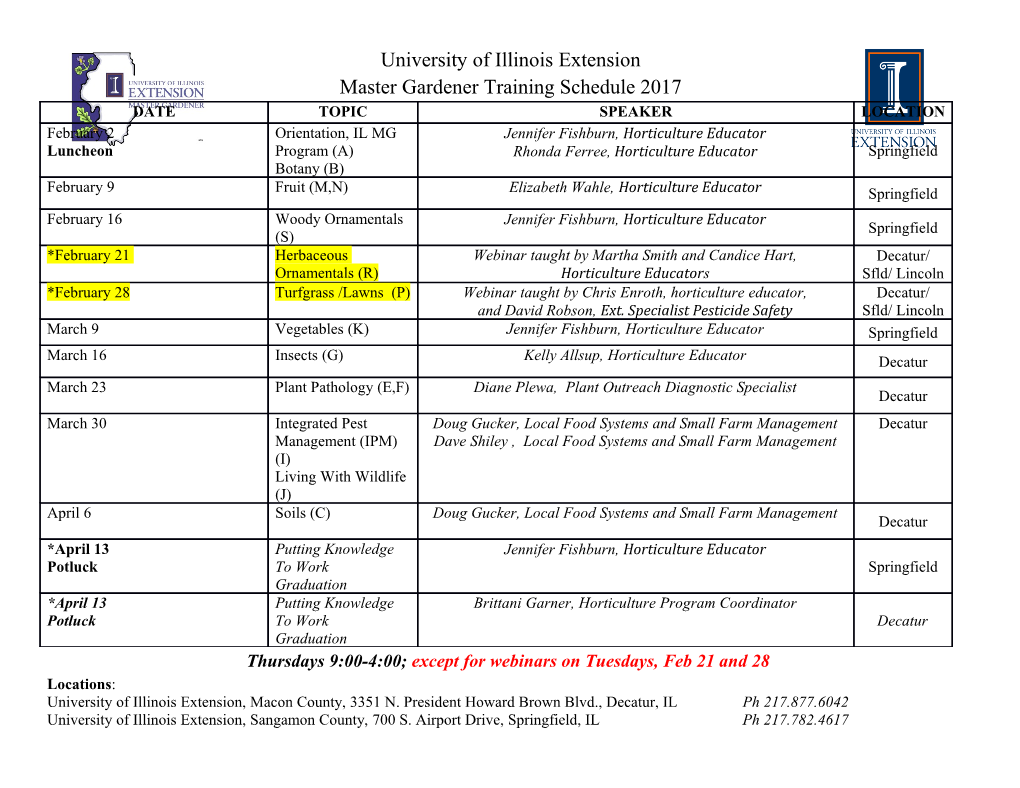
On some generalization of the normal distribution Krzysztof Zajkowski Faculty of Mathematics, University of Bialystok Ciolkowskiego 1M, 15-245 Bialystok, Poland [email protected] Abstract In this paper we propose some new generalization of the normal distribution. We will call it the α-normal (Gaussian) distribution. For α = 2 it becomes the standard normal distribution. We show expression for moments and the differ- ential entropy. We also calculate the (exponential) Orlicz norm of the standard α-normal random variables. 2020 Mathematics Subject Classification: Primary 60E05, Secondary 46E30. Key words: normal distribution, Weibull distribution, differential entropy, sub- exponential random variables, (exponential) Orlicz norms 1 Notion of the α-normal distribution Several types of generalization of the normal distribution can be distinguished. For ex- ample, the first one is the exponential power distribution, a comprehensive description of which can be found in [8]. The second one is the log-normal distribution, but in the form presented by the authors in [5, Appendix 8]. Another example is the skew distri- butions; see e.g. [7]. The above probability distributions become normal distributions for certain parameter values. One can also indicate other distributions related to the normal distribution, such as classical log-normal, folded normal, and inverse normal distributions but unlike the above mentioned generalizations these do not include the normal distribution as the special case. In this paper, we would like to present a generalization of the normal distribu- tion, which will be related, in some sense, to the Weibull distribution. For the same value of the shape parameter, it will have the same order of tail decay as the Weibull distribution. arXiv:2108.00272v2 [math.PR] 5 Aug 2021 The standard exponentially distributed random variable X has exponential tail decay, that is, P(X t) = exp( t) for t 0. Consider a random variable Wα,λ := 1 ≥ − ≥ λX /α for some α,λ > 0. Observe that for t 0 ≥ 1/α α α P(Wα,λ t)= P λX t = P X (t/λ) = exp (t/λ) . ≥ ≥ ≥ − Let us note that Wα,λ has the two-parameter Weibull distribution with the shape parameter α and the scale parameter λ; compare [6, Ch.8]. We will call it as the W eibull(α,λ) random variable (i.e., W W eibull(α,λ)). α,λ ∼ 1 Similarly as in the case of the Weibull distribution we define a generalization of the normal distribution, which was announced in [9]. Definition 1.1. For the standard normally distributed random variable G and pos- itive number α define a symmetric random variable Gα such that Gα has the same 2/α | | distribution as G . We will call Gα the standard α-normal (α-Gaussian) random variable. | | The cumulative distribution function FGα (t) of the α-Gaussian random variable Gα, for t> 0, has the form F (t) = P(G t)=1 P(G > t) Gα α ≤ − α 1 = 1 P( G > t) (by symmetry of G ) − 2 | α| α 1 2 = 1 P( G α > t) (by distribution of G ) − 2 | | α α α α = 1 P(G > t 2 )= P(G t 2 )=Φ(t 2 ), − ≤ where Φ denotes the cumulative distribution function of the standard normal distribu- tion. ′ One can calculate that its density function fα := FGα has the form α α/2−1 −|x|α/2 fα(x)= x e . 2√2π | | Let us stress that for α = 2 we get the density of the standard normal distribution. Density function description (The following description and the graphic were made by the student Jacek Oszczepali´nski). The density function of the random variable Gα is even. One can calculate that, for x> 0, its derivative has the following form 2 α α−4 α 2 1 α ′ 2 α − 2 x fα(x)= − x x − e . 4√2π − α Let us observe that for 0 <α< 2 the density function has infinite negative slope at 0 ′ ′ (limx→0+ fα(x)= ) and it is negative for any x> 0. If α = 2 then limx→0+ f2(x)= ′ −∞ ′ f2(0)=0. If2 <α< 4 then the slope at 0 is infinite positive, for α = 4 limx→0+ f4(x)= ′ 2/√π, and fα(0) = 0 if α > 4. In general, for any 2 < α and x > 0 the slope of the α α−2 ′ α α−2 density function is positive until the value α , fα α = 0, and it is negative q q above α α−2 . q α By the above we get that the form of the density function of the α-normal distri- bution changes drastically with the value of α. And so, for 0 <α< 2, the density 2 function has the vertical asymptote at zero. For α = 2, we have the density of the standard normal distribution. For α > 2, the density function have a local minimum at zero with a value zero and two maxima at α α−2 . ± q α The following graphic shows examples of α-normal density functions depending on the shape parameter, such as α = 1 (red), α = 2 (blue), α = 3 (purple) and α = 5 (green). Figure 1: Density function fα depending on the value of parameter α. Remark 1.2. Because the cumulative distribution function of Gα has the form FGα (x)= α α 2 2 Φ(x ) if x 0 and FGα (x)=1 Φ( x ) if x< 0 then we see that Gα tends in distri- bution to Rademacher’s≥ distribution− | as| α . →∞ Moments and the moment generating function. Since, for G (0, 1) and p> 0, ∼N 2p/2 p +1 E( G p)= Γ , | | √π 2 we immediately get p/α p 2p/α 2 p 1 E( Gα )= E( G )= Γ + . | | | | √π α 2 Thus the moment generating function of Gα equals 1 ∞ (41/αs)k 2k 1 E exp(sG )= Γ + . α √π k! α 2 Xk=0 Now we compare the distribution of Gα with the distributions of the Weibull(α,λ) random variables for some λ’s. It is define that a random variable X majorizes a random variable Y in distribution, if there exists t0 0 such that ≥ P( X t) P( Y t), | | ≥ ≥ | | ≥ 3 for any t > t0; see for instance [1, Def. 1.1.2]. Proposition 1.3. The standard α-normal random variable majorizes the Weibull(α,1) random variable and it is majorized by the Weibull(α, 21/α) random variable. Proof. It is known that the tails of the Gaussian random variable can be estimated from above in the following way P( G t) exp( t2/2) | | ≥ ≤ − for any t 0; see for instance [2, Prop.2.2.1]) . Hence for the α-normal random variable we get ≥ P( G t)= P( G tα/2) exp (t/21/α)α . (1) | α| ≥ | | ≥ ≤ − 1 Let us observe that the right hand side is the tails of the Weibull(α, 2 /α) random variable. It means that the Weibull(α, 21/α) random variable majorizes the α-normal random variable. In the same source [2, Prop.2.2.1]) one can find the following lower estimate of the tails of the Gaussian random variable 1 1 P( G t) exp( t2/2) | | ≥ ≥ t √2π − 2 for t 1. Because √2πt exp( t /2) tends to 0 as t then there exists t0 such that ≥ 2 − 2 →∞ √2πt exp( t /2) 1 for t t0. It gives exp( t /2) 1/√2πt and, in consequence, − ≤ ≥ − ≤ 1 1 P( G t) exp( t2/2) exp( t2) | | ≥ ≥ t √2π − ≥ − for t t0. By the above ≥ 2/α α/2 α P( G t)= P( G t)= P( G t ) exp( t )= P(W 1 t), | α| ≥ | | ≥ | | ≥ ≥ − α, ≥ 2/α for t t0 , which means that the α-normal random variable majorizes the Weibull(α, 1) random≥ variable. Although the the standard α-normal distribution is comparable to the Weibull distribution in the above sense, it is significantly different. We will show it on the ex- ample of the entropy function. Recall that the differential entropy of the two-parameter Weibull distribution is given by the formula 1 λ H(Wα,λ)= γ 1 + ln +1, − α α where γ is the Euler-Mascheroni constant. In the following proposition we derive a formula on the differential entropy for Gα. 4 Proposition 1.4. The differential entropy of Gα has the following form 1 1 2√2π 1 H(Gα)= (γ + ln 2) + ln + , α − 2 α 2 where γ denotes the Euler-Mascheroni constant. Proof. By the definition of the differential entropy and the form of density fα of Gα we get ∞ H(Gα) = fα(x) ln fα(x)dx − Z−∞ ∞ α α 1 α = fα(x) ln + 1 ln x x dx − Z−∞ h 2√2π 2 − | | − 2| | i ∞ ∞ 2√2π α 1 α = ln + 1 ln x fα(x)dx + x fα(x)dx. (2) α − 2 Z−∞ | | 2 Z−∞ | | Let us observe that ∞ α α 2 x fα(x)dx = E Gα = EG =1. (3) Z−∞ | | | | The integral ∞ ∞ α α/2−1 −|x|α/2 ln x fα(x)dx = ln x x e dx. (4) Z−∞ | | 2√2π Z−∞ | || | Substituting u = xα/2 (x> 0) we obtain ∞ ∞ 2 1 α 2 8 1 2 α/ − −|x| / − 2 u ln x x e dx = 2 e ln udu. (5) Z−∞ | || | α Z0 By [4, 4.333] we have that ∞ 1 2 1 e− 2 u ln udu = (γ + ln 2)√2π (6) Z0 −4 Summing up (6), (5), (4), (3) and substituting into (2) we obtain 1 1 2√2π 1 H(Gα)= (γ + ln 2) + ln + .
Details
-
File Typepdf
-
Upload Time-
-
Content LanguagesEnglish
-
Upload UserAnonymous/Not logged-in
-
File Pages7 Page
-
File Size-