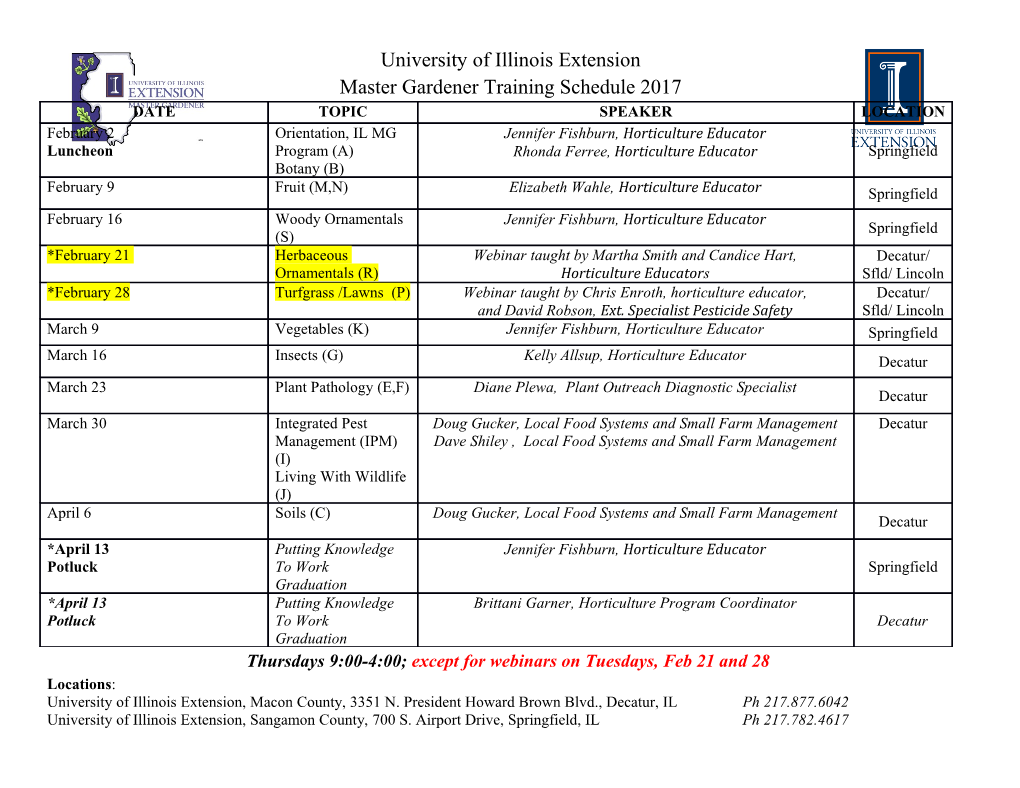
Symmetry 2010, 2, 1108-1120; doi:10.3390/sym2021108 OPEN ACCESS symmetry ISSN 2073-8994 www.mdpi.com/journal/symmetry Review Minimum Phi-Divergence Estimators and Phi-Divergence Test Statistics in Contingency Tables with Symmetry Structure: An Overview Leandro Pardo 1;? and Nirian Mart´ın 2 1 Department of Statistics and O.R., Complutense University of Madrid, 28040 Madrid, Spain 2 Department of Statistics, Carlos III University of Madrid, 28903 Getafe (Madrid), Spain ? Author to whom correspondence should be addressed; E-Mail: [email protected]. Received: 12 March 2010; in revised form: 7 May 2010 / Accepted: 10 June 2010 / Published: 11 June 2010 Abstract: In the last years minimum phi-divergence estimators (MϕE) and phi-divergence test statistics (ϕTS) have been introduced as a very good alternative to classical likelihood ratio test and maximum likelihood estimator for different statistical problems. The main purpose of this paper is to present an overview of the main results presented until now in contingency tables with symmetry structure on the basis of (MϕE) and (ϕTS). Keywords: minimum phi-divergence estimator; phi-divergence statistics; symmetry model Classification: MSC 62B10, 62H15 1. Introduction An interesting problem in a two-way contingency table is to investigate whether there are symmetric patterns in the data: Cell probabilities on one side of the main diagonal are a mirror image of those on the other side. This problem was first discussed by Bowker [1] who gave the maximum likelihood estimator as well as a large sample chi-square type test for the null hypothesis of symmetry. The minimum discrimination information estimator was proposed in [2] and the minimum chi-squared estimator in [3]. In [4–7] new families of test statistics, based on ϕ-divergence measures, were introduced. These families contain as a particular case the test statistic given by [1] as well as the likelihood ratio test. Symmetry 2010, 2 1109 Let X and Y denote two ordinal response variables, X and Y having I levels. When we classify subjects on both variables, there are I2 possible combinations of classifications. The responses (X; Y ) of a subject randomly chosen from some population have a probability distribution. Let pij = Pr(X = i; Y = j), with pij > 0, i; j = 1; :::; I. We display this distribution in a rectangular table having I rows for the categories of X and I columns for the categories of Y . Consider a random sample ofP size nPon (X; Y ) and we denote by nij the observed frequency in the (i; j)th cell for 2 × I I (i; j) I I with i=1 j=1 nij = n. The classical problem of testing for symmetry is given by H0 : pij = pji, (i; j) 2 I × I (1) versus ∗ 6 H1 : pij = pji, for at least one (i; j) pair (2) This problem was considered for the first time by Bowker [1] using the Pearson test statistic XI XI (n − n )2 X2 = ij ji (3) n + n i=1 j=1 ij ji i<j 2 ∼ 2 1 − for which established that X χk for large n, where k = 2 I(I 1). In some real problems (i.e., medicine, psychology, sociology, etc.) the categorical response variables (X; Y ) represent the measure after or before a treatment. In such situations our interest is to determine the treatment effect, i.e., if X ≥ Y (we assume that X represents the measure after the treatment and Y before the treatment). In the following we understand that X is preferred or indifferent to Y , according to joint likelihood ratio ordering, if and only if (iff) pij ≥ pji 8i ≥ j. In this situation the alternative hypothesis is H1 : pij ≥ pji, for all i ≥ j (4) This problem was first considered by El Barmi and Kochar [8], who presented the likelihood ratio test for the problem of testing H0 : pij = pji against H1 : pij ≥ pji, 8i ≥ j and considered the application of it to a real life problem: They tested if the vision of both the eyes, for 7477 women, is the same against the alternative that the right eye has better vision than the left eye. In [5] these results were extended using ϕ-divergence measures. In this paper we present an overview on contingency tables with symmetry structure on the basis of divergence measures. We pay especial attention to the family of ϕ-divergence test statistics for testing ∗ H0 versus H1 , H0 against H1 and also for testing H1 against the alternative H2 of no restrictions over pij’s, i.e., H1 : pij ≥ pji, 8i ≥ j, against H2 : pij ̸≥ pji, 8i ≥ j (5) It is interesting to observe that not only we consider ϕ-divergence test statistics but also we consider minimum ϕ-divergence estimators in order to estimate of the parameters of the model. Symmetry 2010, 2 1110 2. Phi-divergences Measures We consider the set P P f ≤ ≤ 6 I I g Θ = θ : θ = (pij; 1 i; j I; (i; j) = (I;I)) with pij > 0 and i=1 j=1;(i;j)=(6 I;I)pij < 1 (6) P P T − I I × and we denote p(θ) = p = (p11; :::; pII ) , pII = 1 i=1 j=1;(i;j)=(6 I;I)pij or equivalently by the I I matrix p(θ) = [pij]. The ϕ-divergence between two probability distributions p = [pij], q = [qij] was introduced independently by [9] and [10]. It is defined as follows: ( ) XI XI p D (p; q) = q ϕ ij , ϕ 2 Φ∗ (7) ϕ ij q i=1 j=1 ij where Φ∗ is the class of all convex functions ϕ : [0; 1) ! R [ f1g, such that ϕ (1) = 0, ϕ00 (1) > 0; ∗ and we define 0ϕ (0=0) = 0 and 0ϕ (p=0) = limu!1 ϕ (u) =u. For every ϕ 2 Φ that is differentiable at x = 1, the function given by 0 (x) = ϕ (x) − ϕ (1) (x − 1) ∗ also belongs to Φ . Then we have D (p; q) = Dϕ(p; q), and has the additional property that 0 (1) = 0. Because the two divergence measures are equivalent, we can consider the set Φ∗ to be equivalent to the set Φ ≡ Φ∗ \ fϕ : ϕ0 (1) = 0g An important family of ϕ-divergences in statistical problems, is the power divergence family xλ+1 − x + λ (1 − x) ϕ (x) = ; λ =6 0; λ =6 −1 (8) (λ) λ (λ + 1) ϕ(0) (x) = lim ϕ(λ) (x) = x ln x − x + 1 λ!0 ϕ(−1) (x) = lim ϕ(λ) (x) = − ln x + x − 1 λ→−1 which was introduced and studied by [11]. Notice that ϕ(λ) 2 Φ. In the following we shall 2 R denote the power-divergence measures by Dϕ(λ) (p; q), λ . For more details about ϕ-divergence measures see [12]. ∗ 3. Hypothesis Testing: H0 versus H1 We define I(I+1) −1 P f T 2 R 2 g B = (a11; :::; a1I ; a22;:::; a2I ; :::; aI−1I−1; aI−1I ) + : i≤jaij < 1, i; j = 1; ::; I the hypothesis (1) can be written as T H0 : θ = g(β); β= (p11; :::; p1I ; p22; :::; p2I ; :::; pI−1I−1; pI−1I ) 2B (9) Symmetry 2010, 2 1111 where the function β is defined by β = (gij; i; j = 1; :::; I; (i; j) =6 (I;I)) with ( pij; i ≤ j gij(β) = ; i; j = 1; :::; I − 1 pji; i > j and gIj(β) = pjI ; j = 1; :::; I − 1 giI (β) = piI ; i = 1; :::; I − 1 T Note that p(g(β)) = (gij(β); i; j = 1; :::; I) , where P − I gII (β) = 1 i;j=1;(i;j)=(6 I;I)gij(β) The maximum likelihood estimator (MLE) of β can be defined as b β = arg min DKL(pb; p(g(β))) a.s. β2B where DKL(pb; p(g(β))) is the Kullback–Leibler divergence measure (see [13,14]) defined by XI XI pb D (pb; p(g(β))) = pb log ij KL ij g (β) i=1 j=1 ij b b b b b T b pbij +pbji We denote by θ = g(θ) and by p(θ) = (p11(θ); :::; pII (θ)) . It is well known that pij(θ) = 2 ; i = 1; :::; I; j = 1; :::; I : Using the ideas developed in [15], we can consider the minimum ϕ2-divergence estimator (Mϕ2E) replacing the Kullback–Leibler divergence by a ϕ2-divergence measure in the following way bϕ2 b 2 ∗ θ = arg min Dϕ2 ((p; p(g(β)))); ϕ2 Φ (10) β2B where ( ) XI XI pb D (pb; p(g(β))) = g (β)ϕ ij ϕ2 ij 2 g (β) i=1 j=1 ij S,ϕ ϕ We denote θb 2 = g(βb 2 ) and we have (see [7,16]) p ( ) bϕ2 − −!L N S −1 n(g(β ) β) 0; IF (β) n!1 where IS (θ) = Σ − Σ B(θ)T (B(θ)ΣT B(θ))−1B(θ)Σ F θ ( θ ) θ θ T @hij (θ) being Σθ = diag(θ)−θθ and B(θ) = . The functions hij are given by @θij I(I−1) × 2− 2 (I 1) hij(θ) = pij − pji; i < j, i = 1; :::; I − 1, j = 1; :::; I S It is not difficult to establish that the matrix IF (θ) can be written as S T −1 IF (θ) = M β IF (β) M β Symmetry 2010, 2 1112 where IF (β) is the Fisher information matrix corresponding to β 2 B. If we consider the family of power divergences we get the minimum power-divergence estimator, S,λ θb of θ, under the hypothesis of symmetry, whose expression is given by ! 1 bλ+1 bλ+1 λ+1 pij + pji 2 bS,λ θij = ! 1 ; i; j = 1; :::; I (11) XI XI bλ+1 bλ+1 λ+1 pij + pji 2 i=1 j=1 For λ = 0 we get pb + pb θbS;0 = ij ji ; i; j = 1; :::; I ij 2 hence, we obtain the maximum likelihood estimator for symmetry introduced by [1].
Details
-
File Typepdf
-
Upload Time-
-
Content LanguagesEnglish
-
Upload UserAnonymous/Not logged-in
-
File Pages13 Page
-
File Size-