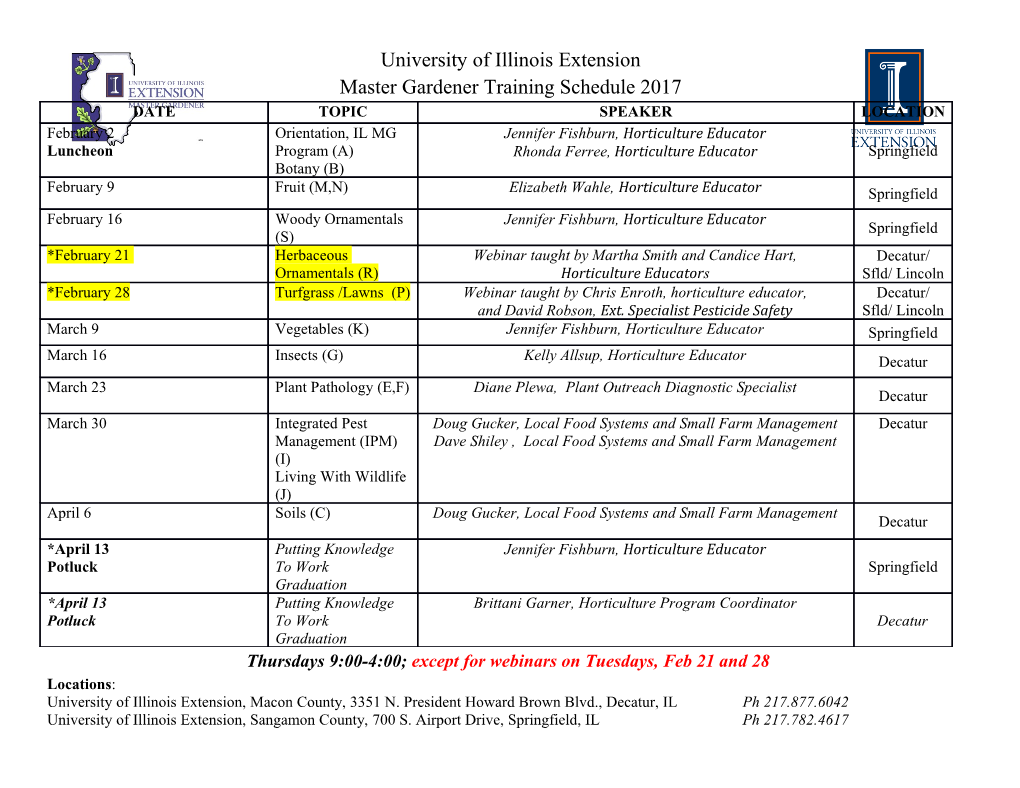
Hyperplane based Classification: Perceptron and (Intro to) Support Vector Machines Piyush Rai CS5350/6350: Machine Learning September 8, 2011 (CS5350/6350) Hyperplane based Classification September8,2011 1/20 Hyperplane Separates a D-dimensional space into two half-spaces Defined by an outward pointing normal vector w RD ∈ (CS5350/6350) Hyperplane based Classification September8,2011 2/20 Hyperplane Separates a D-dimensional space into two half-spaces Defined by an outward pointing normal vector w RD ∈ w is orthogonal to any vector lying on the hyperplane (CS5350/6350) Hyperplane based Classification September8,2011 2/20 Hyperplane Separates a D-dimensional space into two half-spaces Defined by an outward pointing normal vector w RD ∈ w is orthogonal to any vector lying on the hyperplane Assumption: The hyperplane passes through origin. (CS5350/6350) Hyperplane based Classification September8,2011 2/20 Hyperplane Separates a D-dimensional space into two half-spaces Defined by an outward pointing normal vector w RD ∈ w is orthogonal to any vector lying on the hyperplane Assumption: The hyperplane passes through origin. If not, have a bias term b; we will then need both w and b to define it b > 0 means moving it parallely along w (b < 0 means in opposite direction) (CS5350/6350) Hyperplane based Classification September8,2011 2/20 Linear Classification via Hyperplanes Linear Classifiers: Represent the decision boundary by a hyperplane w For binary classification, w is assumed to point towards the positive class (CS5350/6350) Hyperplane based Classification September8,2011 3/20 Linear Classification via Hyperplanes Linear Classifiers: Represent the decision boundary by a hyperplane w For binary classification, w is assumed to point towards the positive class Classification rule: y sign T b sign D w x b = (w x+ )= (Pj=1 j j + ) (CS5350/6350) Hyperplane based Classification September8,2011 3/20 Linear Classification via Hyperplanes Linear Classifiers: Represent the decision boundary by a hyperplane w For binary classification, w is assumed to point towards the positive class Classification rule: y sign T b sign D w x b = (w x+ )= (Pj=1 j j + ) wT x + b > 0 ⇒ y = +1 wT x + b < 0 ⇒ y = −1 (CS5350/6350) Hyperplane based Classification September8,2011 3/20 Linear Classification via Hyperplanes Linear Classifiers: Represent the decision boundary by a hyperplane w For binary classification, w is assumed to point towards the positive class Classification rule: y sign T b sign D w x b = (w x+ )= (Pj=1 j j + ) wT x + b > 0 ⇒ y = +1 wT x + b < 0 ⇒ y = −1 (CS5350/6350) Hyperplane based Classification September8,2011 3/20 Linear Classification via Hyperplanes Linear Classifiers: Represent the decision boundary by a hyperplane w For binary classification, w is assumed to point towards the positive class Classification rule: y sign T b sign D w x b = (w x+ )= (Pj=1 j j + ) wT x + b > 0 ⇒ y = +1 wT x + b < 0 ⇒ y = −1 Question: What about the points x for which wT x + b = 0? (CS5350/6350) Hyperplane based Classification September8,2011 3/20 Linear Classification via Hyperplanes Linear Classifiers: Represent the decision boundary by a hyperplane w For binary classification, w is assumed to point towards the positive class Classification rule: y sign T b sign D w x b = (w x+ )= (Pj=1 j j + ) wT x + b > 0 ⇒ y = +1 wT x + b < 0 ⇒ y = −1 Question: What about the points x for which wT x + b = 0? Goal: To learn the hyperplane (w, b) using the training data (CS5350/6350) Hyperplane based Classification September8,2011 3/20 Concept of Margins Geometric margin γn of an example xn is its distance from the hyperplane wT x + b γ = n n w || || (CS5350/6350) Hyperplane based Classification September8,2011 4/20 Concept of Margins Geometric margin γn of an example xn is its distance from the hyperplane wT x + b γ = n n w || || Geometric margin may be positive (if yn = +1) or negative (if yn = 1) − Margin of a set x ,..., xN is the minimum absolute geometric margin { 1 } T (w xn + b) γ = min γn = min | | 1≤n≤N | | 1≤n≤N w || || (CS5350/6350) Hyperplane based Classification September8,2011 4/20 Concept of Margins Geometric margin γn of an example xn is its distance from the hyperplane wT x + b γ = n n w || || Geometric margin may be positive (if yn = +1) or negative (if yn = 1) − Margin of a set x ,..., xN is the minimum absolute geometric margin { 1 } T (w xn + b) γ = min γn = min | | 1≤n≤N | | 1≤n≤N w || || T Functional margin of a training example: yn(w xn + b) (CS5350/6350) Hyperplane based Classification September8,2011 4/20 Concept of Margins Geometric margin γn of an example xn is its distance from the hyperplane wT x + b γ = n n w || || Geometric margin may be positive (if yn = +1) or negative (if yn = 1) − Margin of a set x ,..., xN is the minimum absolute geometric margin { 1 } T (w xn + b) γ = min γn = min | | 1≤n≤N | | 1≤n≤N w || || T Functional margin of a training example: yn(w xn + b) Positive if prediction is correct; Negative if prediction is incorrect (CS5350/6350) Hyperplane based Classification September8,2011 4/20 Concept of Margins Geometric margin γn of an example xn is its distance from the hyperplane wT x + b γ = n n w || || Geometric margin may be positive (if yn = +1) or negative (if yn = 1) − Margin of a set x ,..., xN is the minimum absolute geometric margin { 1 } T (w xn + b) γ = min γn = min | | 1≤n≤N | | 1≤n≤N w || || T Functional margin of a training example: yn(w xn + b) Positive if prediction is correct; Negative if prediction is incorrect Absolute value of the functional margin = confidence in the predicted label ..or “mis-confidence” if prediction is wrong (CS5350/6350) Hyperplane based Classification September8,2011 4/20 Concept of Margins Geometric margin γn of an example xn is its distance from the hyperplane wT x + b γ = n n w || || Geometric margin may be positive (if yn = +1) or negative (if yn = 1) − Margin of a set x ,..., xN is the minimum absolute geometric margin { 1 } T (w xn + b) γ = min γn = min | | 1≤n≤N | | 1≤n≤N w || || T Functional margin of a training example: yn(w xn + b) Positive if prediction is correct; Negative if prediction is incorrect Absolute value of the functional margin = confidence in the predicted label ..or “mis-confidence” if prediction is wrong large margin ⇒ high confidence (CS5350/6350) Hyperplane based Classification September8,2011 4/20 The Perceptron Algorithm One of the earliest algorithms for linear classification (Rosenblatt, 1958) Based on finding a separating hyperplane of the data (CS5350/6350) Hyperplane based Classification September8,2011 5/20 The Perceptron Algorithm One of the earliest algorithms for linear classification (Rosenblatt, 1958) Based on finding a separating hyperplane of the data Guaranteed to find a separating hyperplane if the data is linearly separable (CS5350/6350) Hyperplane based Classification September8,2011 5/20 The Perceptron Algorithm One of the earliest algorithms for linear classification (Rosenblatt, 1958) Based on finding a separating hyperplane of the data Guaranteed to find a separating hyperplane if the data is linearly separable If data not linear separable Make it linearly separable (more on this when we cover Kernel Methods) (CS5350/6350) Hyperplane based Classification September8,2011 5/20 The Perceptron Algorithm One of the earliest algorithms for linear classification (Rosenblatt, 1958) Based on finding a separating hyperplane of the data Guaranteed to find a separating hyperplane if the data is linearly separable If data not linear separable Make it linearly separable (more on this when we cover Kernel Methods) .. or use a combination of multiple perceptrons (Neural Networks) (CS5350/6350) Hyperplane based Classification September8,2011 5/20 The Perceptron Algorithm Cycles through the training data by processing training examples one at a time (an online algorithm) (CS5350/6350) Hyperplane based Classification September8,2011 6/20 The Perceptron Algorithm Cycles through the training data by processing training examples one at a time (an online algorithm) Starts with some initialization for (w, b) (e.g., w = [0,..., 0]; b = 0) (CS5350/6350) Hyperplane based Classification September8,2011 6/20 The Perceptron Algorithm Cycles through the training data by processing training examples one at a time (an online algorithm) Starts with some initialization for (w, b) (e.g., w = [0,..., 0]; b = 0) An iterative mistake-driven learning algorithm for updating (w, b) (CS5350/6350) Hyperplane based Classification September8,2011 6/20 The Perceptron Algorithm Cycles through the training data by processing training examples one at a time (an online algorithm) Starts with some initialization for (w, b) (e.g., w = [0,..., 0]; b = 0) An iterative mistake-driven learning algorithm for updating (w, b) Don’t update if w correctly predicts the label of the current training example (CS5350/6350) Hyperplane based Classification September8,2011 6/20 The Perceptron Algorithm Cycles through the training data by processing training examples one at a time (an online algorithm) Starts with some initialization for (w, b) (e.g., w = [0,..., 0]; b = 0) An iterative mistake-driven learning algorithm for updating (w, b) Don’t update if w correctly predicts the label of the current training example Update w when it mispredicts the label of the current training example (CS5350/6350) Hyperplane based Classification September8,2011 6/20 The Perceptron Algorithm Cycles through the training data by processing training examples one at a time (an online algorithm) Starts with some initialization for (w, b) (e.g., w = [0,..., 0]; b = 0) An iterative
Details
-
File Typepdf
-
Upload Time-
-
Content LanguagesEnglish
-
Upload UserAnonymous/Not logged-in
-
File Pages117 Page
-
File Size-