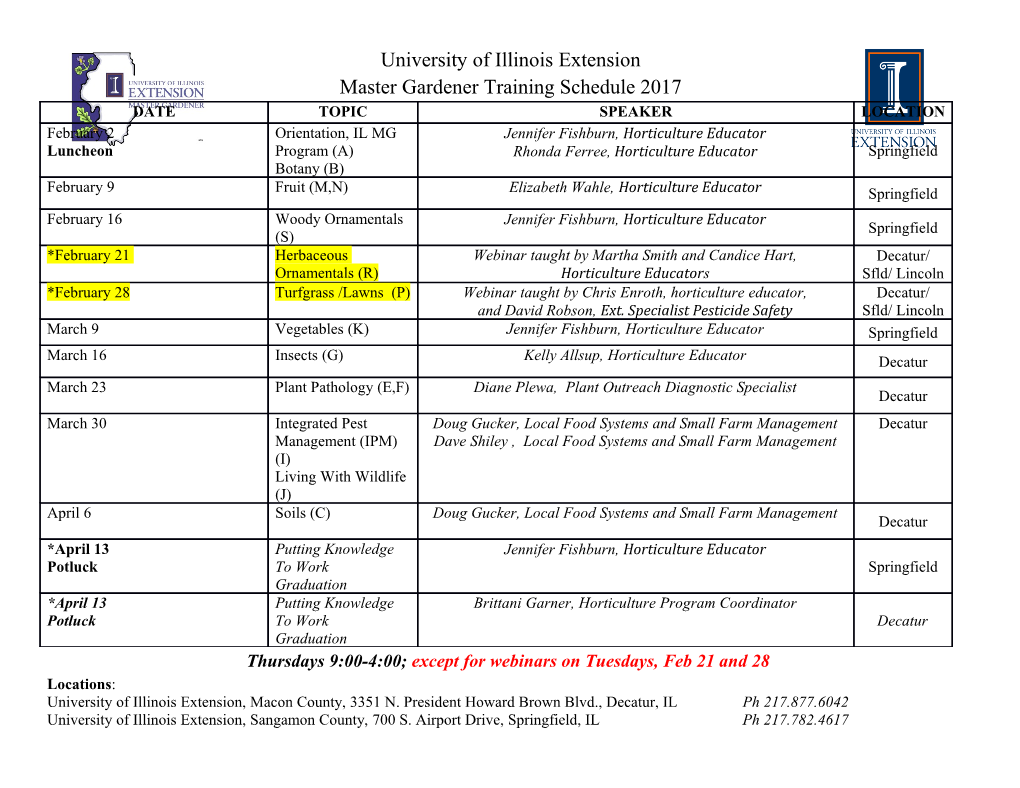
Chapter 5 Optimization The field of optimization is vast and complex, and much of it lies beyond the scope of this course. We will focus on the core techniques for optimization commonly en- countered in the context of robotics. If you are interested in more on optimization, please see the bibliography for this section — most of the resources referenced go into much more depth than we will in these notes. 5.1 Calculus for Optimization Many common optimization techniques are rooted in calculus. As such, we will begin our study of optimization with a review of some concepts from this area. We will assume basic knowledge of Calculus I through III (roughly, derivatives, integrals, and techniques for computing both, as well as the basics of vectors and vector-valued functions). For a more complete introduction to calculus, we recom- mend the excellent resources available in Dawkins [1,2,3]. 5.1.1 Partial Derivatives Most optimization problems encountered in robotics pertain to functions of mul- tiple variables. As such, we often need to be able to compute a derivative of one of these functions with respect to a single variable. We have already seen partial derivatives in earlier sections (such as in the Euler-Lagrange equations), but they are especially critical for solving optimization problems of many kinds. We will now briefly review the definition and computation of a partial derivative: Definition 5.1 (The Partial Derivative of a Real-Valued Function) For some func- tion f : n ! , i.e. f(x ; : : : ; x ), we define @f , the partial derivative R R 1 n @xi of f with respect to x , as @f = d g(x ), where g(x ) is defined as f with i @xi dxi i i 130 5.1. CALCULUS FOR OPTIMIZATION 131 x ; : : : ; x ; x ; : : : ; x held constant. In other words, @f is the derivative of f 1 i−1 i+1 n @xi with every input variable other than xi treated as though it were constant. Given this definition, we can see that computing a partial derivative is nearly equivalent to computing an ordinary derivative. The partial derivative of a function is the ordinary derivative of that function with all but one variable treated as con- stant, so computing a partial derivative is as simple as imagining that every variable other than some xi is a constant and computing the ordinary derivative of f with respect to xi. As an example, consider the following: 2 4x2 Example 5.1 Take f(x; y; z) = 3 sin(x) tan (y) + log(z) . We wish to compute @f @f @f @x and @z @x . @f To begin, we will compute @x by taking y and z to be constants and computing as follows: d d 2 4x2 dx g(x) = dx 3 sin(x) tan (y) + log(z) d 2 d 4x2 = dx 3 sin(x) tan (y) + dx log(z) The derivative of a sum is the sum of the derivatives 2 d 4 d 2 = 3 tan (y) dx sin(x) + log(z) dx x Pull out the constants 2 8x = 3 tan (y) cos(x) + log(z) @f @f @f Computing @z @x is very similar. We begin with @x as computed above and proceed as follows: d d 2 8x dz h(z) = dz 3 cos(x) tan (y) + log(z) d 2 d 8x = dz 3 cos(x) tan (y) + dz log(z) The derivative of a sum is the sum of the derivatives d 1 = 0 + 8x dz log(z) The first term is all constants, with a derivative of zero = −8x z log2(z) As you may be able to see from this example, the order in which we evaluate partial derivatives does not change the final result. The intuition for this property is that the other variables in the equation play no role in the computation of a partial 132 CHAPTER 5. OPTIMIZATION derivative with respect to some specific variable, so the partial derivatives each have @f @f a sort of independence. To better see this for yourself, try computing @x @z and @f @f comparing your result to the result of @z @x in the above example. For more on the partial derivative, see Dawkins [3]. 5.1.2 The Gradient The gradient is core to a large number of optimization techniques. It can be con- sidered as a more general version of the derivative, expanding the notion of the change of a function with respect to some variable to multiple dimensions. Com- puting the gradient is quite simple: For a function with input variables x1; : : : ; xn, the gradient is the vector consisting of the function’s partial derivative with respect to each of the xi in turn. More formally: Definition 5.2 (Gradient of a Real-Valued Function) Given a differentiable func- n tion f : R ! R, i.e. f(x1; : : : ; xn), we define the gradient of f to be rf = h @f ;:::; @f i, the vector of all first-order partial derivatives of f. @x1 @xn The gradient is useful because it tells us the direction of increase of a function at every point. If we imagine placing a ball at a point (x1; : : : ; xn) on the surface created by the values of a function f, then (−∇f)(x1; : : : ; xn) tells us which way that ball would roll (obviously, this intuition makes the most sense when n = 2 or 3). The gradient’s value is a vector with magnitude equivalent to the slope of f and direction equivalent to the direction in which f is increasing fastest. The utility of the gradient to optimization is thus fairly straightforward: If we want to solve an optimization problem, we typically want to find the point(s) where a function is at its extrema — where it is largest or smallest. Given that the gra- dient tells us the direction of increase of a function (and thus also the direction of decrease of a function, by negating the gradient), it seems intuitive that we could “follow” the gradient to find the extrema of the function. We will see some tech- niques for doing so in Section 5.2. Finally, we have already encountered the Jacobian matrix in a number of con- texts. The Jacobian can be thought of as the further generalization of the gradient to vector-valued functions. It is the matrix of first order derivatives of a vector- valued function. In short, each row of the Jacobian of a vector-valued function f~ is the gradient of each element of the column vector which comprises f~, in order. 5.1.3 The Hessian Matrix The Hessian matrix for a function is a measure of the function’s local curvature. It has many applications, some of which we will see in later sections. For now, we 5.1. CALCULUS FOR OPTIMIZATION 133 will simply define the Hessian. n Definition 5.3 (The Hessian) For a twice-differentiable function f : R ! R, the Hessian matrix H is defined as: 2 @2f @2f @2f 3 2 ··· @x1 @x1@x2 @x1@xn 6 @2f @2f @2f 7 2 ··· 6 @x2@x1 @x @x2@xn 7 H = 6 2 7 (5.1) 6 . 7 6 . .. 7 4 5 @2f @2f @2f ··· 2 @xn@x1 @xn@x2 @xn In other words, the Hessian is the matrix of all second-order partial derivatives of f. 5.1.4 Critical Points & Critical Point Tests Before we begin considering more advanced methods of optimizing functions, we will briefly review one of the most fundamental methods of finding the extrema of a function: computing its critical points and evaluating their second-order properties. Definition 5.4 (Critical Points of a Real-Valued Function) Given a continuously n differentiable function f : R ! R, a point x = (x1; : : : ; xn) is considered a critical point of f iff f(x) exists and either rf(x) = ~0 or rf(x) does not exist. In this section, we will only consider functions for which we can fairly easily compute the critical points algebraically. Section 5.2 and much of the rest of this chapter discusses methods for approximating the extrema of functions, which in some cases includes approximating their critical points. In general, the procedure for finding the critical points of a function f is as follows: Compute rf, and then use algebra to find the roots of rf, as well as any points where at least one component of rf does not exist. The resulting set of points is the set of critical points for f. Once we have the critical points of f, we need some way to find out whether or not they are extrema. Recall that while all extrema are critical points, not all critical points are extrema. If f is twice differentiable (meaning that we can compute its second-order gradient), then we can use the second derivative test to check its critical points. n For f of the form we have been considering thus far (i.e. f : R ! R), we can perform the second derivative test for a critical point c by the following procedure: First, compute the Hessian matrix H (see Section 5.1.3) of f at c, and then the 1 eigenvalues of H. If all of the eigenvalues λi of H are positive, then f has a local 1Recall that an eigenvalue λ of a matrix A is a root of the characteristic polynomial of A, jA − λIj = 0, where I is the identity matrix. 134 CHAPTER 5. OPTIMIZATION minimum at c, If all λi are negative, then f has a local maximum at c.
Details
-
File Typepdf
-
Upload Time-
-
Content LanguagesEnglish
-
Upload UserAnonymous/Not logged-in
-
File Pages11 Page
-
File Size-