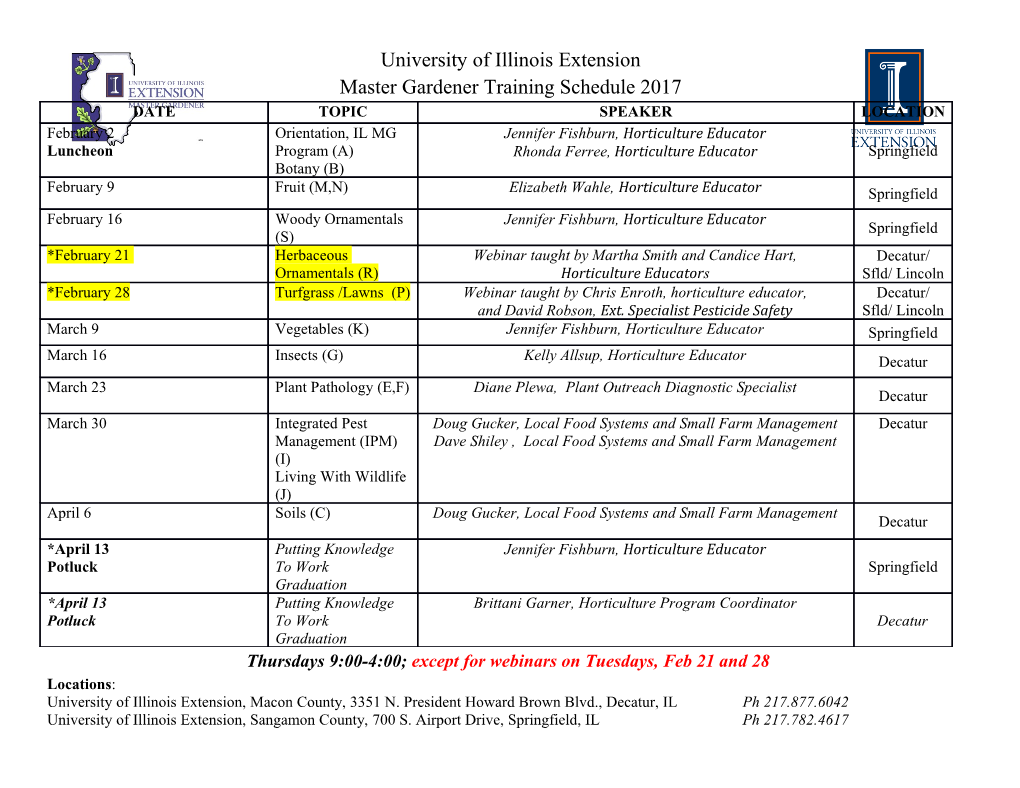
Solving Human Centric Challenges in Ambient Intelligence Environments to Meet Societal Needs A Dissertation Presented to the Faculty of the School of Engineering and Applied Science University of Virginia In Partial Fulfillment of the requirements for the Degree Doctor of Philosophy (Computer Science) by Erin Griffiths December 2019 © 2019 Erin Griffiths Approval Sheet This dissertation is submitted in partial fulfillment of the requirements for the degree of Doctor of Philosophy (Computer Science) Erin Griffiths This dissertation has been read and approved by the Examining Committee: Kamin Whitehouse, Adviser Jack Stankovic, Committee Chair Mary Lou Soffa A.J. Brush John Lach Accepted for the School of Engineering and Applied Science: Dean, School of Engineering and Applied Science December 2019 i To everyone who has helped me along the way. ii Abstract In the world today there exists a large number of problems that are of great societal concern, but suffer from a problem called the tragedy of the commons where there isn`t enough individual incentive for people to change their behavior to benefit the whole. One of the biggest examples of this is in energy consumption where research has shown that we can reduce 20-50% of the energy used in buildings if people would consistently modify their behavior. However, consistent behavior modification to meet societal goals that are often low priority on a personal level is often prohibitively difficult in the long term. Even systems design to assist in meeting these needs may be unused or disabled if they require too much effort, infringe on privacy, or are frustratingly inaccurate. We need a way to automatically meet societal needs while reducing or eliminating the burden on users. The vision of Ambient Intelligent Environments (AmI) has been dedicated to meeting this and other human supportive goals since first being introduced in the late 1990s. Ambient intelligence environments are computational systems embedded in the physical environment that sense, reason about, and act for the benefit of the people in that environment and their objectives. The key factor, and challenge, of an ambient intelligence environment is that it serves its objectives invisibly and transparently, with little to no requirements on the user's behavior or cognitive load, but still incorporates the user in all its actions. It is not by accessing a computer terminal that people interact with the system, but by normal interactions with their environment and the objects within. This seems ideal for meeting societal concerns where users do not want to put any additional effort into the system. This dissertation aims to explore some of the practical uses and challenges of sensing and reasoning in ambient intelligence environments. First, I show how AmI environments are needed to personalize solutions to societal needs, such as shifting the energy peak created by daily energy use. This personalization can improve widespread adoption by allowing a system to benefit every user and not just the average one. Then, I explore two novel solutions to the two main human-centric challenges in ambient intelligence environments that may prevent the adoption of solutions for meeting societal needs: privacy vs. data collection and having the human in the loop vs. automation. Privacy is challenging in AmI because personal data is needed to create iii Abstract iv personalization, but many systems collect more private data than they need for operation. We present a novel solution to one of the worst examples of this, cameras, by using hardware limitations to preserve privacy. Keeping humans in the loop in AmI is challenging since we cannot ask for their preferences and instead need to learn through their natural interactions with the environment. In physical AmI, the interactions used to learn such preferences are often few and far between, resulting in a \small data" problem for learning. This problem is further exacerbated as preferences change over time and the system must quickly adapt. We present a novel solution that leverages the similarity between users in reinforcement learning to quickly learn in the face of these challenges. We anticipate that this work and these solutions to the two challenges of AmI will increase the accuracy, privacy, and ultimate adoption of AmI technologies to meet societal needs. Contents Contents v List of Tables . vii List of Figures . viii 1 Motivation: Meeting Societal Needs Through Sensors, Learning, and Control 1 1.1 Overview and Motivation . 1 1.2 Thesis Statement . 5 1.3 Contributions . 5 1.4 Thesis Outline . 6 2 Background and Related Work: Ambient Intelligence Environments 7 2.1 Ambient Intelligence Environments . 7 2.2 Applications in AmI . 10 2.3 Privacy in AmI . 11 2.4 Human Participation in AmI . 13 3 Using AmI to Meet Societal Needs 15 3.1 Introduction . 15 3.2 Related Work . 17 3.3 ThermalThrift: An Ambient Intelligence Water Heater . 19 3.3.1 Water Heater Tank Model . 20 3.3.2 Consumer-Facing Cost Optimization . 23 3.3.3 Utility-Facing Peak Optimization . 26 3.4 Experimental Setup . 28 3.4.1 Usage Data . 29 3.4.2 TOU Pricing . 29 3.4.3 Baseline and Optimal Algorithms . 30 3.4.4 Water Heater Simulation . 32 3.5 Results . 32 3.5.1 Consumer-Facing Cost Minimization . 33 3.5.2 Utility-Facing Peak Optimization . 35 3.5.3 Learning Period Analysis . 36 3.5.4 TES Potential and the Mixing Valve . 36 3.5.5 TES Power Peak . 37 3.6 Limitations and Future Work . 38 3.7 Conclusions . 40 4 Preserving Image Privacy for AmI Sensing 41 4.1 Introduction . 41 4.2 Related Work . 44 4.3 Lethe: A Privacy Preserving Camera for Multi-User Environments . 45 4.3.1 Crossing Detection . 46 4.3.2 Identity Detection . 50 v Contents vi 4.3.3 Memory, Data Transmission, and Privacy . 54 4.4 Experimental Setup . 55 4.4.1 Thermal Camera Implementation . 56 4.4.2 Ground Truth . 56 4.4.3 Height Variation Data Collection . 57 4.4.4 Mixed Crossing/Non-crossing Data Collection . 57 4.4.5 Evaluation Metrics . 58 4.5 Results . 58 4.5.1 Crossing Detection . 59 4.5.2 Identity Detection . 61 4.5.3 Hair, Head Coverings, and Background Temperature . 63 4.5.4 The Effect of Frame Rate and Resolution on Identity . 64 4.5.5 Why not use an RGB camera? . 66 4.6 Limitations and Future Work . 67 4.7 Conclusions . 69 5 Leveraging Similarity for Learning in AmI 71 5.1 Introduction . 71 5.2 Related Work . 73 5.3 Reinforcement Learning Background Information . 75 5.4 KindredRL: Leveraging Human Similarity to Learn Changing Behaviors . 77 5.4.1 Learning Framework . 79 5.4.2 Neighborhood Creation . 80 5.4.3 Collaborative Preference Estimation . 82 5.5 Experimental Setup . 84 5.5.1 Thermostat Application . 86 5.5.2 Blinds Application . 87 5.5.3 Water Heating Application . 87 5.5.4 Approach Parameters . 89 5.5.5 Simulation Parameters . 89 5.5.6 Baselines . 90 5.5.7 Metrics . 91 5.6 Results . 92 5.6.1 Selection of Learning Rate . 93 5.6.2 Selection of Neighborhood Parameter . 95 5.6.3 Selection of Estimation Horizon . 95 5.6.4 Effect of Occupancy and Response Rate . 96 5.6.5 Effect of Staggered Preference Change Period and Preference Length . 97 5.6.6 Water Heating . ..
Details
-
File Typepdf
-
Upload Time-
-
Content LanguagesEnglish
-
Upload UserAnonymous/Not logged-in
-
File Pages129 Page
-
File Size-