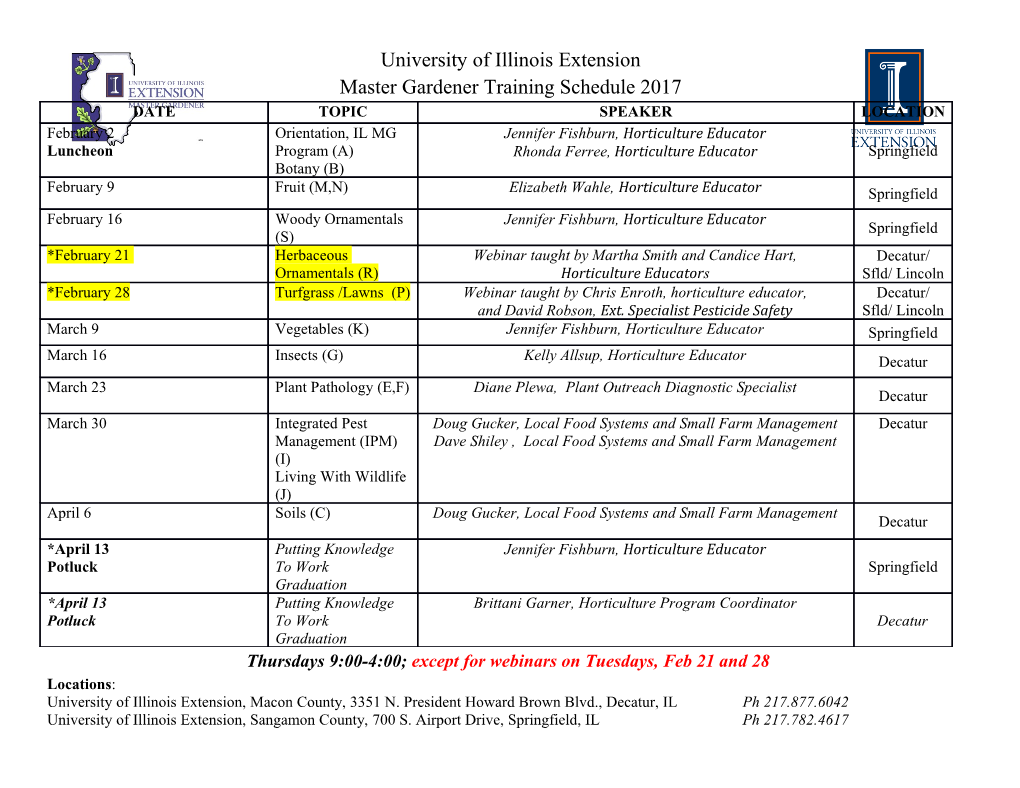
WHITE PAPER Revolutionizing Category Management By Embracing AI ABSTRACT Applied to the important planning process known as category management (CatMan), an emerging class of cross-enterprise AI capabilities can enhance the output of all functions within the consumer-packaged goods (CPG) managerial ecosystem. That makes an AI platform more than just an analytical tool. Because it enables cross-enterprise management and enhances competitive differentiation, CPG executives need to understand why and how to focus the power of AI on specific business- aligned use cases. Written by Gordon Wade, in collaboration with r4 Technologies Revolutionizing Category Management by Embracing AI Table of Contents Executive Summary 3 Shopper Behavior and New Market Realities Complicate CPG Management 4 Organizational Silos Hinder a Cross-Enterprise Approach in an Age of Rapid Change 5 What Is AI? 5 What Is Cross-Enterprise Management? 6 Cross-Enterprise Capabilities Are Fundamentally Transforming CatMan 6 Applied properly, several AI capabilities can enhance CatMan dramatically 6 Identifying changes in shopper behavior and developing insights rapidly 6 Making merchandising decisions at the most granular levels 7 Improving store-level forecasting 7 How These Capabilities Affect Each Step in the CatMan Process 7 Examples of Driving Volume and Profit, Using AI and CatMan Principles 8 Sample use case #1: A factory accident creates a profitability and customer-satisfaction conundrum 8 Sample use case #2: Optimally introducing a new product line 8 Sample use case #3: Optimizing profits in new and limited space 8 Sample use case #4: A retailer wants to improve its “click-and-collect” profitability 8 Sample use case #5: A retailer wants to prioritize investments 9 Sample use case #6: Where to locate new production capacity 9 What to Ask When Evaluating an AI Platform 9 Should we license an existing third-party platform or build our own system? 9 Does the AI solution require redesigning of legacy systems and processes? or is it an agnostic cloud-based system that can link to existing systems and leverage in-place processes? 9 What kind of data does the platform accept? 10 Does the third-party AI platform continuously learn? 10 Can the platform address issues across the entire enterprise, including demand and supply streams, or is its scope limited? 10 What is the platform’s process for selecting data relevant to your specific issues? 10 Can the platform communicate its recommendations using your existing communication systems? 10 Has The Licensed Platform Addressed Issues Similar To Yours, And If So, What Were The Results? 10 The Race Is to the Swift 11 What to Do Tomorrow 11 About the Author, Gordon Wade 11 About r4 12 www.r4.ai 2 Revolutionizing Category Management by Embracing AI Executive Summary As digitally empowered shoppers disrupt the consumer-packaged goods (CPG) environment, executives can’t manage by using legacy (and now-outmoded) tools. Fortunately, new, sophisticated artificial intelligence (AI) platforms can empower CPG executives to manage and master the complex cross-enterprise and market challenges that are eroding share, volume and margins. An emerging class of cross-enterprise AI capabilities can be applied to the important planning process known as category management (CatMan). Here AI’s remarkable capabilities can enhance every step in the CatMan planning process through its granularity, speed and capability to uncover new shopper insights. Indeed, AI enables a new way of practicing category management, taking it from the current model (CatMan 2.0) to the next level: CatMan 3.0. But AI has another major advantage: it improves the execution of the plans at store level by breaking down functional silos with superior communication capabilities across the supply chain—down to the aisle level. An AI-enabled CatMan 3.0 process enhances the output of all the diverse functions within the CPG managerial ecosystem. AI platforms become not just an analytical tool but a management weapon with significant competitive implications. That’s why CPG executives need to understand why it is so important to focus the power of AI on specific business-aligned use cases. Then they can intelligently decide how to integrate the capabilities of AI in their business, and especially into CatMan. Sample use cases provided in this report provide real-world examples of how AI can transform CatMan by leveraging data insights into better category plans spanning demand and supply. The questions to ask provided in this paper will help CPG executives narrow their focus and cut through the hype when starting to evaluate options for AI capabilities enabling cross-enterprise management and CatMan 3.0. www.r4.ai 3 Revolutionizing Category Management by Embracing AI Shopper Behavior and New Market Realities Complicate CPG Management CPG management has developed organizational structures, processes and tools to compete effectively in commercial environments. As these environments change, managers must develop and deploy new options to address new competitive and market realities. During the 65 years following World War II, the pace of change proceeded at a brisk but manageable rate. Changes were evolutionary, and responses emerged in a timely enough manner to address them effectively. However, in the last 10 years—and especially in the last five years—the rate of change in shopper behavior and attitudes, communication platforms and retail models has accelerated significantly. The rate of change itself has become a source of disruption because managers simply cannot cope with changes coming this rapidly. The bad news is that the rate of change is accelerating. And even more data will be created because of the “digital natives” we know as millennials and Generation Z. These younger consumers, who are completely comfortable with the new shopping tools, don’t shop the way their parents did. They don’t read printed circulars and plan a weekly shopping trip. Instead, digital natives scan screen-based circulars and emails, search the Internet for all kinds of deals from many different retailers, and want to shop whenever it's convenient for them. In short, “shopping” has changed in profound ways. The very act of shopping and buying is creating more data than ever, which in turn is driving the need for and use of more sophisticated analytics. For example, managers depend on data to make informed decisions. But data springs up quickly. It comes in different types with varying levels of detail. Managers often can’t readily tell which data is good, bad, meaningful or meaningless—or which data is critical but missing. They often lack the framework and the tools necessary to develop new conclusions and integrate them into new strategies and tactics. When new retail channels spring up simultaneously, and new supply chain options emerge to accommodate the retail options, the new shopping behavior creates myriad problems and opportunities. Three examples illustrate how new data reveals new opportunities: • A new in-store shopper tracking tool revealed that the placement of end-aisle displays in bricks and mortar retailers had widely varying effects on volume and profit by category and brand. No retailer had measured this effect or quantified the opportunity for incremental volume until the new data revealed it. • A retailer, frustrated by flat category volumes, combined various data sources to reveal that its own customers were buying 30 percent of category volume in other retailers. This huge leakage problem revealed a major volume opportunity that few retailers even knew existed and almost none had developed plans to capture. • New online purchase tracking tools uncovered a wealth of data about shoppers’ evolving retail choices being often driven by price. This opened new opportunities to vary pricing by category, brand and item to attract bargain-hungry shoppers. These are but three examples of opportunities driven by changing shopper attitudes and revealed through new data sources. But the same opportunities also represent threats. As newer brands and more nimble startups have taken advantage of lower cost of entry to address changing behaviors, the practical effect of all these reinforcing changes has been share loss by many existing brands and slow growth by most leading companies. In at least one recent year, nearly all leading brands and retailers lost volume, share or margin; in some cases, it was all three. www.r4.ai 4 Revolutionizing Category Management by Embracing AI Organizational Silos Hinder a Cross-Enterprise Approach in an Age of Rapid Change While shopper behavior is changing rapidly, CPG operational structures and processes are mired in the past. For more than 50 years, CPG companies have organized themselves into functional silos on the demand and supply sides of the enterprise. This model of vertical silos that interconnect and interact primarily in the C-suite served managers well in times of relatively slow change. But in this new era of accelerated change, the silos have become outmoded structures, like the French Maginot Line. Even within the individual demand and supply silos, the pace of change conflicts sharply with established operational structures and processes, hindering rapid decision making. Against fast-changing operational targets, demand data can be overwhelming. Sometimes, it’s not specific enough to be of real value. Other times, the data that’s sufficiently granular comes in such high volumes that it’s too difficult to grasp. Managers don’t know how to piece it together, make sense of it, and most importantly distill insights that align to business needs and goals. Before being passed to a related silo, decisions and processes are delayed as data-derived judgments slowly move up and down a functional silo. The CPG business moves at a snail’s pace. The situation is worse on the supply side. Dramatically different neighborhood demographics make store-by-store forecasting a daunting challenge—especially during promotion periods. Forecasting errors create out-of-stock or overstocked conditions across multiple stores, causing lost sales from out of stocks or increased inventory cost from sub-optimized space usage.
Details
-
File Typepdf
-
Upload Time-
-
Content LanguagesEnglish
-
Upload UserAnonymous/Not logged-in
-
File Pages12 Page
-
File Size-