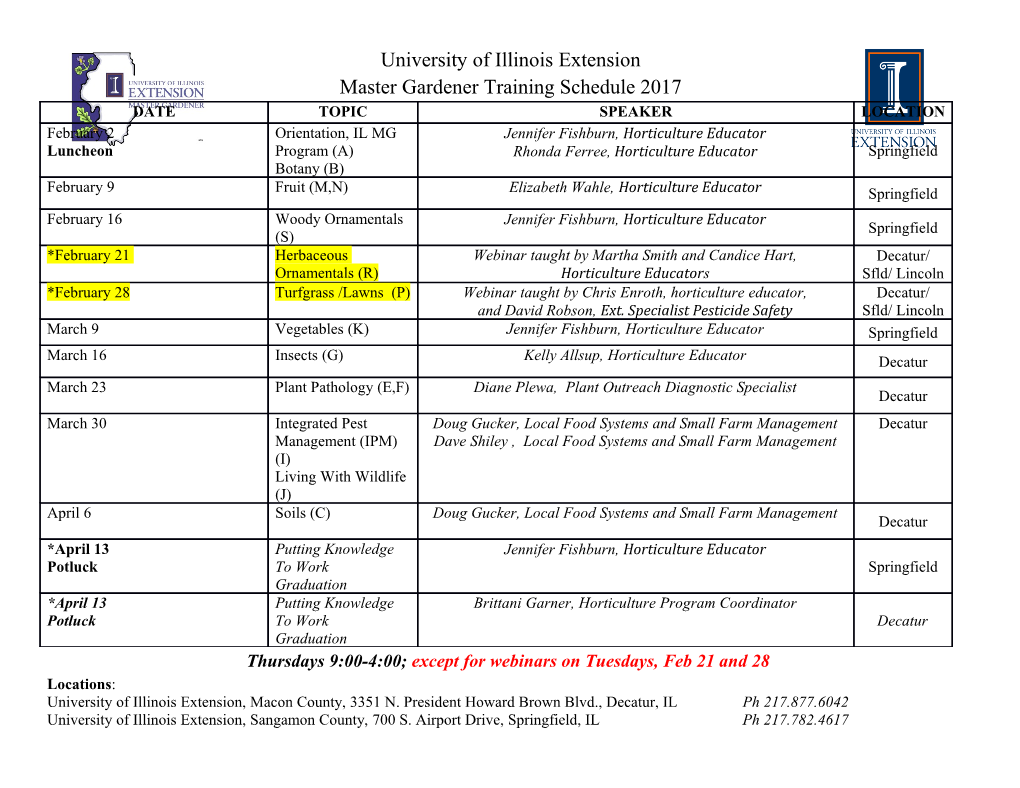
Aston University Final Year Project Revenue Equivalence and the Importance of Auction Design Author: Supervisor: Sulman Khan Prof. David Lowe 119209086 Course: BSc Mathematics with Computing April 30, 2015 Contents Abstract i Acknowledgements ii List of Tables and Figures iii 1 Introduction 1 1.1 Online Advertising . 1 1.2 Game Theory and Ad Auctions . 4 1.2.1 Nash Equilibrium . 5 1.2.2 Risk Neutrality . 6 1.2.3 Designs of Auctions . 6 1.3 Revenue Equivalence Theorem . 7 2 Auctions 9 2.1 Common Auction Designs . 9 2.1.1 Open Bid Auctions . 9 2.1.2 Sealed Bid Auctions . 11 2.2 Importance of Auction Design . 13 2.3 Bidding Functions in First Price Auction . 14 2.3.1 2 Bidders . 14 2.3.2 n Bidders . 16 2.4 Expected Payos in a Second Price Auction . 18 3 Revenue Equivalence Theorem 22 3.1 Expected Revenue . 22 3.1.1 First Price Auction . 22 3.1.2 Second Price Auction . 26 3.2 Violating Equivalence . 30 3.2.1 Risk Approach . 30 3.2.2 Auction Rules . 31 3.3 Example of Auctions Violating Equivalence . 31 4 Conclusion 34 4.1 Evaluation . 34 Appendices 36 1 Basic Statistical Theory . 36 2 Proof of Revenue Equivalence Theorem . 39 References 41 Abstract This project explored the concepts studied in the AM30MR Mathematics Report and develops on the mathematical foundations of the research. It started with a discussion of how auctions have revolutionised the online advertising medium over the last decade. Four common auction designs were discussed; First Price, Second Price, English and the Dutch auction. An important concept from game theory called the Revenue Equivalence Theorem was then explored. Initially derived by William Vickrey in 1961, the theorem states that given bidders are risk neutral with their valuation only known to themselves and that the object is allocated to the bidder with the highest valuation, a seller can expect to gain the same revenue regardless of the auction design. The research concluded that the same revenue can indeed be obtained through various auction mechanisms, veried with the First Price and Second Price designs. Exam- ples using the Uniform distribution on [0,100] were given to illustrate this in practice, followed by a general proof for each mechanism. Scenarios were also proposed where the Revenue Equivalence Theorem may not hold given violation of one or more of the conditions. These included deviating risk attitudes from risk neutrality and breaking of auctions rules such as collusion of bidders, resulting in valuations that are no longer independently distributed. eBay was used as a prime example to show violation of the Revenue Equivalence Theorem, since bidders can publicly view previous bidding information about an item which may aect their bidding behaviour in the remainder of the auction. Primary source reference materials were used when conducting this research, predom- inantly books, journals and original sources where possible to form basic opinions and this was augmented with examples of my own. i Acknowledgements I take this opportunity to thank my supervisor, Professor David Lowe at Aston Univer- sity, for his support this year. He has been a great supervisor to me when completing my Final Year Mathematics Report as well as my Final Year Project, which is greatly appreciated. I would also like to thank my examiner Dr. Juan Neirotti for his time and eorts in examining my work during this research project and in the AM30MR Mathematics Report. The support of my family and friends throughout my studies at Aston is what has helped me to progress. I have developed some great relationships both professionally and personally with students and sta. I stress a big thank you especially to my mother and father for their unreserved support over the last 22 years of my life. ii List of Figures 1 Comparison of Percentage Global Advertising Spend Between 2013-2018 . 2 2 Global Internet Advertising Spend by Category . 3 3 Risk-Utility Indierence Curves . 6 4 Hierarchy of Auction Designs . 10 5 First Price Bidding Prole . 17 6 Bidding in the Second Price Auction b < v ................... 19 7 Overbidding in the Second Price Auction bi < v < b . 19 8 Second Price Payos bi > v ........................... 20 9 Second Price Auction Payos b < v ....................... 20 10 Second Price Auction Payos b < v ....................... 21 11 Bid History in eBay Auctions . 32 12 Uniform Probability Distribution Function (f(x)) . 37 13 Uniform Cumulative Distribution Function (F (x)) . 38 iii 1 Introduction The AM30MR Mathematics Report introduced the concept of online advertisement (ad) auctions and their relation to mathematical auction theory. Concepts such as the Nash Equilibrium were discussed with an analysis into why search engines preferred the Gener- alised Second Price auction to the Generalised First Price. This coincided with Google's unique choice of auction style involving a factor known as quality score when ranking adver- tisements in search results. This research project is about a game theoretical concept that shows dierent auction design types gives the same return to the seller. When designing auc- tions, sellers want to gain the highest revenue possible from their sales. Using the theory of auctions and a brief overview of four of the commons designs used today, the concept known as the Revenue Equivalence Theorem (RET) will be explored. Specic examples implement- ing two dierent auction designs known as the First Price and Second Price mechanisms are used to prove that the RET holds provided particular conditions are fullled. Cases are discussed where designs can violate these conditions to show that the revenue would not be equivalent, concluding with ndings, reection and developments to this research. 1.1 Online Advertising Online advertising has matured dramatically over the past decade[30], from selling adver- tisement spaces, to the introduction of clickability moving towards pop up adverts[16] and then the paid placement model (PPM)[4], an approach that allowed advertisers to purchase more desirable advertising slots on search engines[13]. Why online? User behaviour and surng habits can be measured more eectively online compared to oine[27]. In 1998, a company called GoTo.com introduced the rst auction feature where online adverts were ranked subject to bids submitted by advertisers[13]. Known as ad auctions, they became a new economic revenue stream and by 1999, the online advertising medium had become a $1 billion industry[13]. Today this form of advertising is closing in to become the largest en- tertainment and media advertising segment, forecast to be worth $194.5 billion by 2018[30]. Combined with television advertising, online advertising occupied a joint share of almost 50% of the advertising market in 2013[4]. Figure 1 shows that by 2018, this is forecast to rise to 55% at the expense of newspaper advertising. As of May 2006, the combined value of Google and Yahoo!'s revenue from online advertising exceeded $150 billion[10]. Vieria and Camilo (2014) discuss how the ranking of webpages shares a similar principle to that of supermarket shelf stacking[40]. Product manufacturers pay for their items to be stacked on prime shelves i.e. at eye level or within arm's reach of customers[40]. These items tend to be of a higher price than those on the lowest shelf or topmost shelf, resulting in greater sales and maximum prots[40]. There are limited items that can be stacked on prime shelves. Likewise, a limited number of adverts can be displayed on a page, ranked in order of prominence[13]. Each position has a desirability unique to advertisers[10]. A user is more likely to click a link at the top of their search rather than further down the page[10]. Hence, a website more likely to receive a click has a greater chance of improving business[4] 1 Figure 1: Comparison of global percentage categoric advertising spend between 2013 and 2018. Television and internet occupied 49% of the advertising market in 2013 and is forecast to reach 55% by 2018 with newspapers making the largest cuts in advertising spends. (*CAGR: Compound Annual Growth Rate)[30]. making the top advertising space more demanding to certain advertisers based on their needs. Today companies utilise search engines for advertisement purposes. There are three com- mon forms of online advertising; display adverts, contextual adverts and sponsored search adverts. Display adverts come in dierent forms of banners such as animations or videos often delivered by famous brands[14]. Prices can be xed, negotiated in advance or even decided through auctions similar to those used for sponsored search ad auctions[27]. Con- textual adverts are customised and based on the content a user is currently viewing or has previously viewed. For web search, advertisers submit their maximum bids for particular keywords to the search engine provider[10]. When a user's search query matches the adver- tiser's keyword, an auction commences[38]. The auction concludes when the page, including the ads, has fully loaded[1]. The advertiser with the highest bid has their webpage ranked rst in the search results, with advertisers of successive bids ranked one after the other[10]. On clicking these links, the user is navigated to the company's website. In turn, the company pays the search engine a fee. This approach is called pay per click (PPC)[38]. When multiple companies require the same keyword(s), the ranking of these links and the amount payable to the search engine (per click) invokes the need of auctions. Easley and Kleinberg (2010) discuss how bids for particular queries such as loan consolidation, mortgage renancing, and mesothelioma can reach an excess of $50 per click, for every user that visits the site[8].
Details
-
File Typepdf
-
Upload Time-
-
Content LanguagesEnglish
-
Upload UserAnonymous/Not logged-in
-
File Pages48 Page
-
File Size-