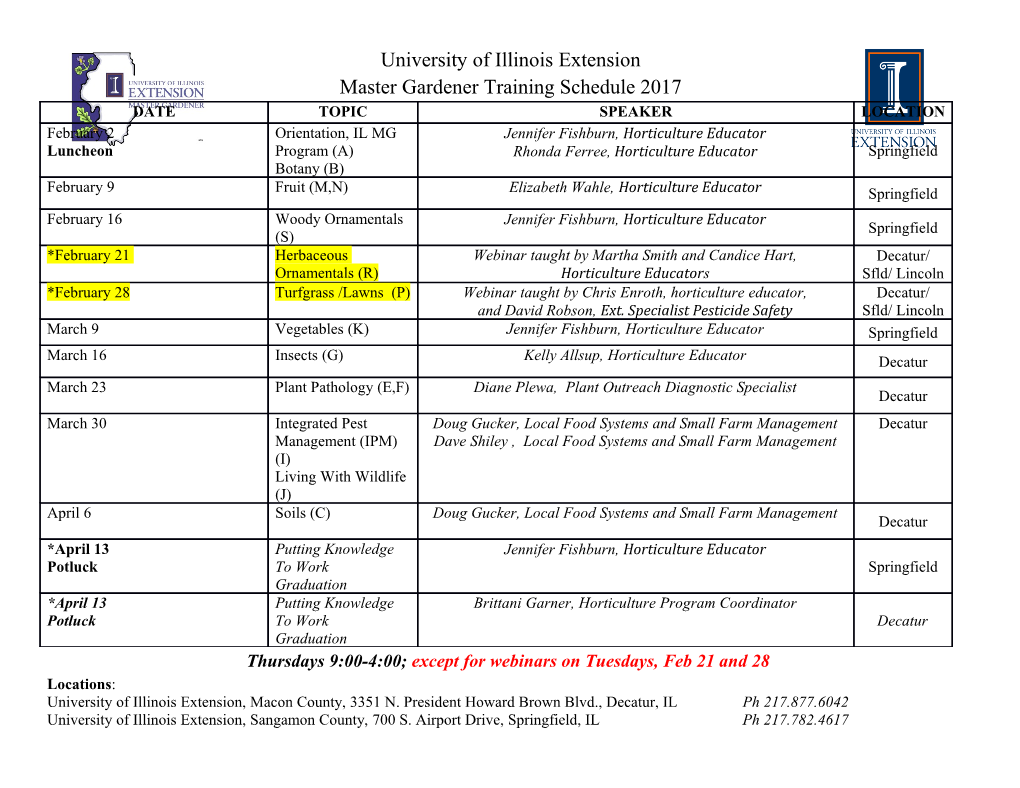
Efficiently Analysing Large Viral Data Sets in Computational Phylogenomics Anna Zhukova, Olivier Gascuel, Sebastián Duchene, Daniel Ayres, Philippe Lemey, Guy Baele To cite this version: Anna Zhukova, Olivier Gascuel, Sebastián Duchene, Daniel Ayres, Philippe Lemey, et al.. Efficiently Analysing Large Viral Data Sets in Computational Phylogenomics. Scornavacca, Celine; Delsuc, Frédéric; Galtier, Nicolas. Phylogenetics in the Genomic Era, No commercial publisher | Authors open access book, pp.5.3:1–5.3:43, 2020. hal-02536435 HAL Id: hal-02536435 https://hal.archives-ouvertes.fr/hal-02536435 Submitted on 10 Apr 2020 HAL is a multi-disciplinary open access L’archive ouverte pluridisciplinaire HAL, est archive for the deposit and dissemination of sci- destinée au dépôt et à la diffusion de documents entific research documents, whether they are pub- scientifiques de niveau recherche, publiés ou non, lished or not. The documents may come from émanant des établissements d’enseignement et de teaching and research institutions in France or recherche français ou étrangers, des laboratoires abroad, or from public or private research centers. publics ou privés. Distributed under a Creative Commons Attribution - NonCommercial - NoDerivatives| 4.0 International License Chapter 5.3 Efficiently Analysing Large Viral Data Sets in Computational Phylogenomics Anna Zhukova Unité Bioinformatique Evolutive, Hub Bioinformatique et Biostatistique, USR3756 (C3BI/DBC), Institut Pasteur & CNRS, Paris, France [email protected] Olivier Gascuel Unité Bioinformatique Evolutive, USR3756 (C3BI/DBC), Institut Pasteur & CNRS, Paris, France Sebastián Duchêne Department of Microbiology and Immunology, Peter Doherty Institute for Infection and Immunity, University of Melbourne, Australia Daniel L. Ayres Center for Bioinformatics and Computational Biology, University of Maryland, USA Philippe Lemey1 Department of Microbiology, Immunology and Transplantation, Rega Institute, KU Leuven – University of Leuven, Leuven, Belgium Guy Baele2 Department of Microbiology, Immunology and Transplantation, Rega Institute, KU Leuven – University of Leuven, Leuven, Belgium [email protected] Abstract Viral evolutionary analyses are confronted with increasingly large sequence data sets, both in terms of sequence length and number of sequences. This can result in considerable computa- tional burden, not only to infer phylogenies but also to obtain associated estimates such as their time scales and phylogeographic patterns. Here, we illustrate two frequently-used approaches to obtain phylogenomic estimates of time-measured trees and spatial dispersal patterns for fast- evolving viruses. First, we discuss computationally efficient procedures that employ a fixed tree topology obtained through maximum likelihood inference to estimate molecular clock rates and phylogeographic spread for Dengue virus genomes. Using the same viral example, we also illustrate Bayesian phylodynamic inference that jointly infers time-measured trees and phylogeo- graphy, including covariates of spatial dispersal, from sequence and trait data. We highlight state-of-the-art efforts to perform such computations more efficiently. Finally, we compare the estimates obtained by both approaches and discuss their strengths and potential pitfalls. How to cite: Anna Zhukova, Olivier Gascuel, Sebastián Duchêne, Daniel L. Ayres, Philippe Lemey, and Guy Baele (2020). Efficiently Analysing Large Viral Data Sets in Computational Phylogenomics. In Scornavacca, C., Delsuc, F., and Galtier, N., editors, Phylogenetics in the 1 PL acknowledges support by the Research Foundation – Flanders (‘Fonds voor Wetenschappelijk Onderzoek – Vlaanderen’, G066215N, G0D5117N and G0B9317N). 2 GB acknowledges support from the Interne Fondsen KU Leuven / Internal Funds KU Leuven under grant agreement C14/18/094, and the Research Foundation – Flanders (‘Fonds voor Wetenschappelijk Onderzoek – Vlaanderen’, G0E1420N). © Anna Zhukova, Olivier Gascuel, Sebastián Duchêne, Daniel L. Ayres, Philippe Lemey, Guy Baele. Licensed under Creative Commons License CC-BY-NC-ND 4.0. Phylogenetics in the genomic era. Editors: Celine Scornavacca, Frédéric Delsuc and Nicolas Galtier; chapter No. 5.3; pp. 5.3:1–5.3:43 $ A book completely handled by researchers. No publisher has been paid. 5.3:2 Efficiently Analysing Large Viral Data Sets Genomic Era, chapter No. 5.3, pp. 5.3:1–5.3:43. No commercial publisher | Authors open access book. The book is freely available at https://hal.inria.fr/PGE. Acknowledgements This work was supported by the EU-H2020 Virogenesis project (grant num- ber 634650), by the INCEPTION project (PIA/ANR-16-CONV-0005), and by the Reservoir- DOCS ERC grant (agreement no. 725422). The Artic Network receives funding from the Wellcome Trust through project 206298/Z/17/Z. 1 Introduction According to a “quick guide” to phylogenomics (Telford, 2007), standard phylogenomic approaches leverage the information present in a large number of genes generated in large- scale genome sequencing efforts. In infectious disease research, phylogenomic alignments that comprise single-nucleotide polymorphism (SNP) data of several hundreds or thousands of genes are now also an important focus of modern microbiology studies. However, for rapidly evolving RNA viruses, phylogenetic and evolutionary analyses remain inherently restricted to small genomes that encode for a limited number of genes. In this chapter, we focus on current challenges and opportunities for evolutionary inference from rapidly evolving viral genomes. This goes beyond common phylogenomic approaches and it is perhaps more in line with some of the earliest published mentions of phylogenomics that refer to a mixed bag of gene or genome analyses within a phylogenetic framework. We will primarily focus on the many different types of analyses that are being used in epidemiology, which shares many common interests with the field of phylodynamics. The mention of “large” viral data sets in our title refers to the increase in two dimensions of the information available for studying molecular epidemiology and virus evolution, which has been brought about by the revolution in sequencing technology. The first dimension concerns the transition from a single gene or a typical PCR amplicon sequenced by Sanger sequencing to complete genomes that are now easily obtained through next generation sequencing, which roughly represents an increase of one order of magnitude for many RNA viruses. This seems less impressive than the increase from a single gene to hundreds of genes that phylogenomic studies of many other organisms have to confront. In addition, due to evolutionary rates that are about a million times faster than our own cellular genes, a limited marker in an RNA virus genome may already offer reasonable resolution for reconstructing evolutionary histories with time-scales of a decade or older. For example, a polymerase gene fragment of about 1 000 bp that is routinely sequenced for drug resistance testing has been extensively and successively used in HIV molecular epidemiology (e.g. Hué et al. 2005) while a fragment of less than half this size – but for the more variable envelope gene – has been used to reconstruct the origin and spread of the virus in Central Africa (Faria et al., 2014). Nevertheless, complete genomes offer an important increase in the resolution of the inferred phylogenies (Yebra et al., 2016), which for short-term outbreak dynamics in particular opens up new opportunities for epidemic reconstructions and tracking transmission. We illustrate this increase of phylogenetic resolution by comparing maximum likelihood trees for complete genome data and the corresponding glycoprotein gene sequences for the 2013 − 2016 West African Ebola virus outbreak in Figure 1. In this case, a single gene does not provide sufficient information about clustering of Ebola virus isolates whereas complete genomes do offer reasonable phylogenetic resolution allowing to identify some degree of structuring by country of sampling. Complete genome sequencing has in recent years become the standard in outbreak Zhukova et al. 5.3:3 complete genome glycoprotein 2.0E-4 4.0E-4 Figure 1 Maximum likelihood phylogenetic trees of Ebola virus inferred using complete genomes (left) and only the glycoprotein gene (right). Branches are coloured according to country (green: Guinea; blue: Sierra Leone; red: Liberia), based on a parsimony reconstruction for internal nodes. Complete genome data allow to infer reasonably resolved phylogenetic trees with a discernible structuring of lineages by country, whereas a single gene produces a multitude of polytomies and essentially prevents from uncovering any relevant (geographic) structure in the resulting phylogeny. surveillance as illustrated in recent work on Zika (Faria et al., 2017), Chikungunya (Naveca et al., 2019) and Yellow Fever (Faria et al., 2018). Such complete genome data also come with specific challenges, such as the need to take into account recombination in different viruses or the difficulty to combine segments for viruses that undergo reassortment, or with the general challenge of increased computation times in likelihood-based phylogenetics (Chapter 1.2 [Stamatakis and Kozlov 2020]). In this chapter, we devote a great deal of attention to describing the methods that can be employed to address the computational challenges caused by these increases in data set sizes and model complexity. Related to this, Chapter 5.4 (Ayres et al. 2020) describes improvements in the latest version of the
Details
-
File Typepdf
-
Upload Time-
-
Content LanguagesEnglish
-
Upload UserAnonymous/Not logged-in
-
File Pages44 Page
-
File Size-