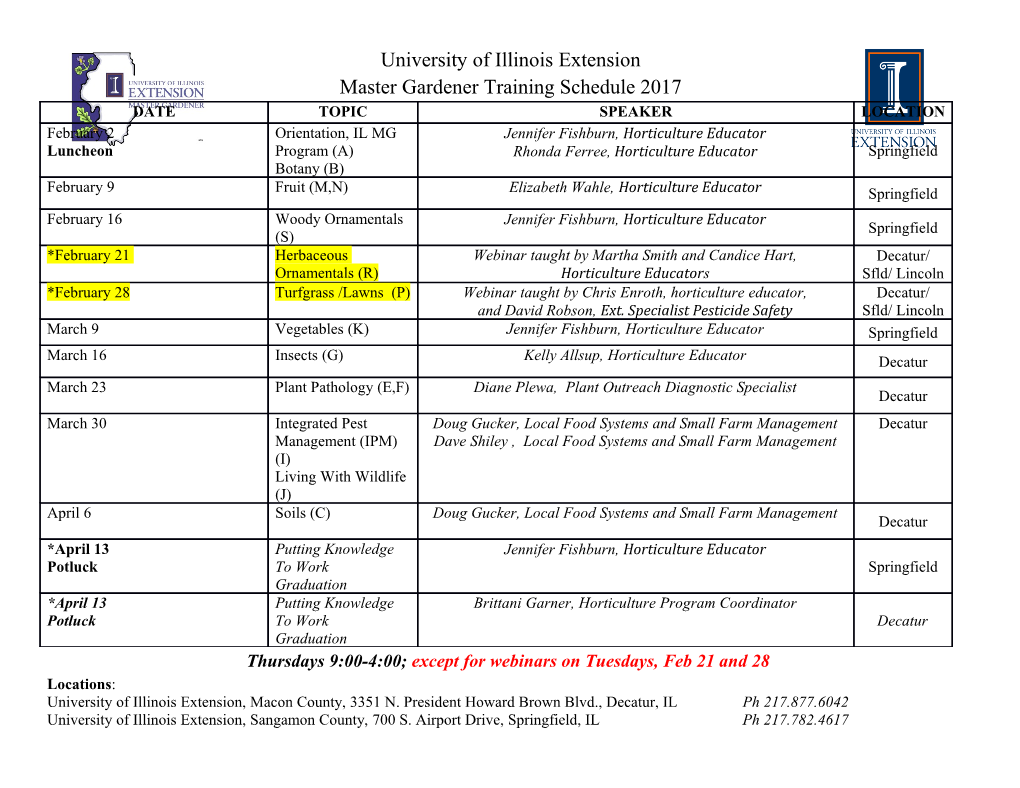
PROTEINS:Structure,Function,andGenetics43:161–174(2001) LatticeProteinFoldingWithTwoandFour-BodyStatistical Potentials HinHarkGan,1 AlexanderTropsha,2 andTamarSchlick1* 1DepartmentofChemistryandCourantInstituteofMathematicalSciences,NewYorkUniversityandtheHowardHughes MedicalInstitute,NewYork,NewYork 2LaboratoryforMolecularModeling,SchoolofPharmacy,UniversityofNorthCarolina,ChapelHill,NorthCarolina ABSTRACT Thecooperativefoldingofpro- cooperativeinteractionsinnativeproteins—becomesfea- teinsimpliesadescriptionbymultibodypotentials. sible. Suchmultibodypotentialscanbegeneralizedfrom Whiletwo-bodypotentialsareadequateformostcon- commontwo-bodystatisticalpotentialsthrougha densedsystems,theimportanceofmultibodypotentials relationtoprobabilitydistributionsofresidueclus- increasesfordensemolecularsystemssuchascompact tersviatheBoltzmanncondition.Inthisexplor- nativeproteinstructures.Multibodypotentialsmayhelp atorystudy,wecompareafour-bodystatistical improveourunderstandingofthecooperativityofprotein potential,definedbytheDelaunaytessellationof foldingprocessandtheregularityofproteinstructures. proteinstructures,totheMiyazawa–Jernigan(MJ) Recentproteinfoldingstudieswithmultibodypotential potentialforproteinstructureprediction,usinga termsshowthattheyplayaroleinstabilizingproteinfolds latticechaingrowthalgorithm.Weusethefour- andinenhancingcooperativityofthefolding/unfolding bodypotentialasadiscriminatoryfunctionfor process.Forexample,Liwoetal.4,11 haveintroducedthree conformationalensemblesgeneratedwiththeMJ andfour-bodycorrelationtermsintheirunited-residue potentialandexamineperformanceonasetof22 potentials,whicharisefromtheexpansionofmeanpoten- proteinsof30–76residuesinlength.Wefindthatthe tials.Four-bodyhydrogenbondingcorrelationtermshave four-bodypotentialyieldscomparableresultstothe alsobeenintroducedphenomenologicallybyKolinskiand two-bodyMJpotential,namely,anaveragecoordi- Skolnick8 intheirlatticeMonteCarloproteinstructure nateroot-mean-squaredeviation(cRMSD)valueof8 predictionalgorithm. ␣ Åforthelowestenergyconfigurationsofall- pro- Togainabetterunderstandingofmultibodypotentials, teins,andsomewhatpoorercRMSDvaluesforother weformulatemultibodymeanpotentialsintermsofthe proteinclasses.Forbothtwoandfour-bodypoten- potentialsofmeanforceinstatisticalmechanics.12 This tials,superpositionsofsomepredictedandnative formulationimpliesthatthemultibodycontactenergyand structuresshowaroughoverallagreement.Formu- probabilityofobservingclustersofresiduesaregenerally latingthefour-bodypotentialusinglargerdatasets relatedbytheBoltzmanncondition.Furthermore,lower- anddirect,butcostly,generationofconformational ordermeanpotentialscanbederivedfromthehigher- ensembleswithmultibodypotentialsmayofferfur- orderexpressions.Followingthemethodologyofstatistical therimprovements.Proteins2001;43:161–174. functions,3,13 suchmeanpotentialscanbeapproximately ©2001Wiley-Liss,Inc. derivedfromtheproteinstructuraldatabase.Sincethey yieldagreateramountofinformationaboutmany-body Keywords:latticemodel;statisticalpotential;multi- correlationsincompactmolecularsystems,multibody bodypotentials;chaingrowthalgo- potentialsmaybettercharacterizenativeproteins.How- rithm;MonteCarlo ever,derivingthesepotentialsrequireslargeproteinstruc- INTRODUCTION turaldatabasesforaccurateformulations. Inthiswork,weexamineafour-bodypotentialdevel- Statisticalpotentialsderivedfromsimplifiedrepresenta- 14 tionsofproteinresiduesarewidelyusedinproteinstruc- opedbyTropshaandVaismanandcoworkers. Byusinga tureprediction.Becauseofrapidgrowthofproteinstruc- threadingtechnique,theseresearchersshowedthattheir turaldatabases,manyofthecurrentresiduepotentials statisticalpotentialcandiscerncorrectsequence/structure areeitherderiveddirectlyfromtheproteindatabase1–3 or indirectlybyhavingtheparametersofthechosenfunc- 4 Grantsponsor:NationalInstitutesofHealth;Grantnumber: tionalformsdeterminedbythecrystalstructures. These GM55164;Grantnumber:RR08102;Grantsponsor:NationalScience statisticalpotentialshavefoundwide-rangingapplica- Foundation;Grantnumber:BIR-94-23827ER;Grantnumber:ASC- tionsintheevaluationofstructure/sequencecompatibility 9704681. 5,6 7 *Correspondenceto:TamarSchlick,DepartmentofChemistryand ofproteins, homologymodeling, andproteinfolding CourantInstituteofMathematicalSciences,NewYorkUniversityand 8–10 simulations. Currently,moststatisticalpotentialsare theHowardHughesMedicalInstitute,251MercerStreet,NewYork, two-body,suchastheMiyazawa–Jernigan1,2 (MJ)and NY10012.E-mail:[email protected] Sippl3 potentials.Asalargerstructuraldatabaseemerges, Received4August2000;Accepted8December2000 thedevelopmentofmultibodypotentials—tomodelthe Publishedonline00Month2001 ©2001WILEY-LISS,INC. 162 H.H. GAN ET AL. matches better than two-body statistical potentials.15,16 In in native proteins are related to their observed frequency the present investigation, we discuss the evaluation of this in a representative structural database. Since energies are potential and its implementation for lattice protein folding computed from a set of folded structures, they are more using a chain growth algorithm. The evaluation is per- appropriately interpreted as a mean, rather than as bare formed by means of a statistical geometrical method in interaction energies. These mean energies are related to which the four-body residue neighbors are systematically potentials of mean force in statistical mechanics, obtained enumerated using the Delaunay tessellation technique. by ensemble averaging over equilibrium states.13,18 In Four-body energies are then related to the frequencies of structure-derived potentials, ensemble averaging is effec- observed four-residue clusters in protein structures via the tively replaced by averaging over a set of representative Boltzmann relation. Our evaluation shows that while most protein structures. Critical assessment of this interpreta- terms have attained their saturation values, some have tion using a simple two-residue hydrophobic–hydrophilic not converged, indicating that a larger structural database (HP) protein model shows that it yields a correct ranking of is needed to determine this potential more accurately. contact energies, but their absolute values are imprecise.18 We compare the performance of the four-body potential The following discussion presents two-body and multibody in protein structure prediction to the two-body MJ poten- potentials as potentials of mean force. tial using a lattice C␣ protein model. On the (311) cubic For a protein chain with N residue interaction centers, lattice, we generate configurational ensembles for proteins we define the n-body potential of mean force w(n) for the 17 with our recently implemented chain growth algorithm. residue-cluster (i1i2...in)as We have shown that this variant is effective for calculating ͑n͒ ϭ Ϫ ͓ ͑n͒ ͔ conformational and thermodynamic properties of several wi1i2 ...in kBT ln Fi1i2 ...in/Ri1i2 ...in (1) test proteins.17 In this article, we examine the quality of where R is the reference state (defined below) and the two and four-body potentials using coordinate root- i1i2...in (n) mean-square deviation (cRMSD) between native and pre- F is the probability density of finding the residues in a dicted structures and energy/cRMSD scatter plots. As the cluster: computational cost in generating ensembles using the four-body potential is currently prohibitive, we can only ͑ ͒ ͑ Ϫ ͒ ͑ Ϫ ͒ F n ϭ ͵ dV N n exp͑ϪE ͒/͵ dV N 1 exp͑ϪE ͒ (2) apply it to the conformational ensembles generated with i1i2 ...in N N the two-body potential. We find that the shapes of the  ϭ energy/cRMSD plots for the two and four-body potentials where the temperature parameter 1/(kBT), the vol- (N Ϫ n) ϭ are correlated for low-energy and low cRMSD configura- ume element dV (dV1dV2...dVN)/ (dV dV ...dV ), and E is the total energy of the protein. tions. The two and four-body energies are generally weakly i1 i2 in N correlated. The n-body potential is defined with respect to a “reference state” R determined by the specific problem of inter- For a set of 22 proteins, the predicted cRMSD values by i1i2...in the MJ potential are about8Åfor␣ proteins and some- est. Moreover, what poorer, around 9 Å, for  and ␣/ protein classes. ͑n͒ ϭ Superpositions of predicted and native structures show Fi1i2 ...in Ri1i2 ...in (3) rough overall agreements for some proteins. Our four-body when the mean potential w(n) ϭ 0. potential yields comparable results. Improving the four- i1i2...in body potential requires larger protein data sets than used It is useful to separate correlated from uncorrelated as- in the present study. Furthermore, a more sophisticated pects of many-body interactions. The reference state is often protein model that uses finer representation for each chosen to be the uncorrelated state where there are no residue may be required. Thus, although the present interactions between residues. If the interaction energy EN vanishes, F(n) in eq. 2 becomes a product of the uncorre- four-body potential may be adequate for protein fold i1i2...in recognition, further improvements are necessary for more lated, single residue properties. Mathematically, we write R ϳ ͟n R , where R is the frequency of occurrence of accurate ab initio structure prediction. i1i2...in a ia ia individual residues. Consequently, the mean potential w(n) i1i2...in STATISTICAL POTENTIALS is interpreted as a measure of the nonrandom nature of residue distributions, or contacts, in protein structures. The We begin by presenting the modified MJ potential, the correlations among the residues in the cluster (i i
Details
-
File Typepdf
-
Upload Time-
-
Content LanguagesEnglish
-
Upload UserAnonymous/Not logged-in
-
File Pages14 Page
-
File Size-