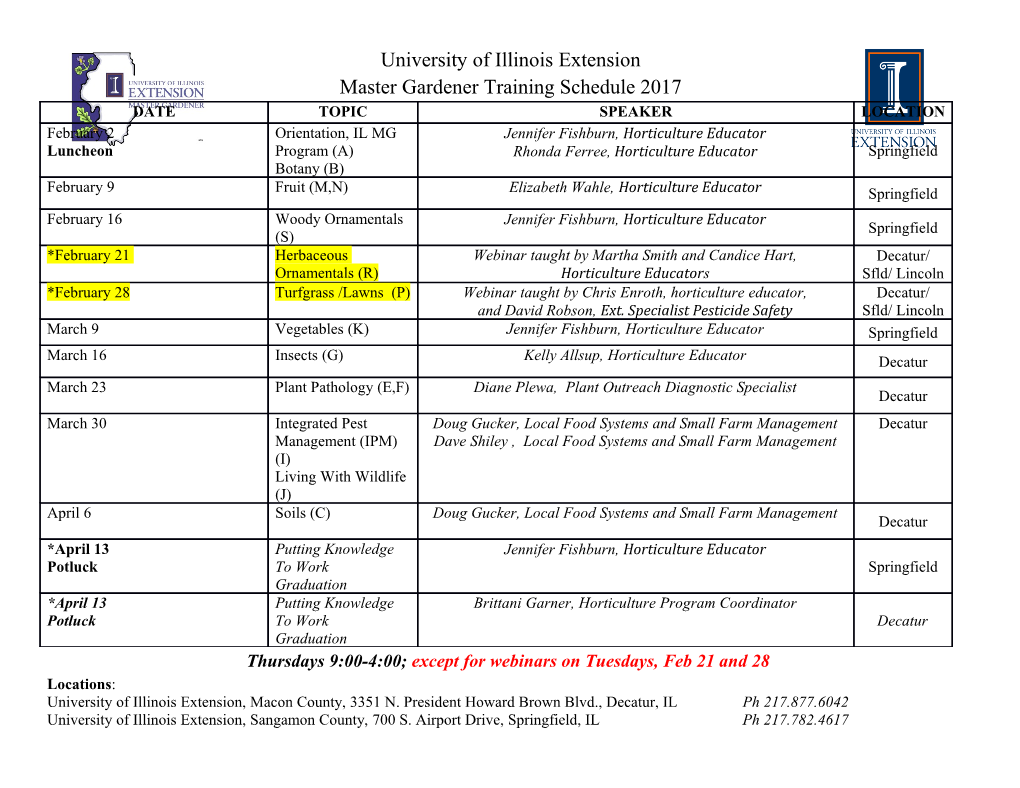
Accelerating Applications with Pattern-specific Optimizations on Accelerators and Coprocessors Dissertation Presented in Partial Fulfillment of the Requirements for the Degree Doctor of Philosophy in the Graduate School of The Ohio State University By Linchuan Chen, B.S., M.S. Graduate Program in Computer Science and Engineering The Ohio State University 2015 Dissertation Committee: Dr. Gagan Agrawal, Advisor Dr. P. Sadayappan Dr. Feng Qin ⃝c Copyright by Linchuan Chen 2015 Abstract Because of the bottleneck in the increase of clock frequency, multi-cores emerged as a way of improving the overall performance of CPUs. In the recent decade, many-cores begin to play a more and more important role in scientific computing. The highly cost- effective nature of many-cores makes them extremely suitable for data-intensive computa- tions. Specifically, many-cores are in the forms of GPUs (e.g., NVIDIA or AMD GPUs) and more recently, coprocessers (Intel MIC). Even though these highly parallel architec- tures offer significant amount of computation power, it is very hard to program them, and harder to fully exploit the computation power of them. Combing the power of multi-cores and many-cores, i.e., making use of the heterogeneous cores is extremely complicated. Our efforts have been made on performing optimizations to important sets of appli- cations on such parallel systems. We address this issue from the perspective of commu- nication patterns. Scientific applications can be classified based on the properties (com- munication patterns), which have been specified in the Berkeley Dwarfs many years ago. By investigating the characteristics of each class, we are able to derive efficient execution strategies, across different levels of the parallelism. We design a high-level programming API, as well as implement an efficient runtime system with pattern-specific optimization- s, considering the characteristics of the hardware platform. Thus, instead of providing a general programming model, we provide separate APIs for each communication pattern. ii We have worked on a selected subset of the communication patterns, including MapRe- duce, generalized reductions, irregular reductions, stencil computations and graph process- ing. Our targeted platforms are single GPUs, coupled CPU-GPUs, heterogeneous clusters, and Intel Xeon Phis. Our work not only focuses on efficiently executing a communication pattern on a single multi-core or many-core, but also considers inter-device and inter-node task scheduling. While implementing a specific communication pattern, we consider as- pects including lock-reducing, data locality, and load balancing. Our work starts with the optimization of the MapReduce on a single GPU, specifically aiming to efficiently utilize the shared memory. We design a reduction based approach, which is able to keep the memory consumption low by avoiding the storage of intermediate key-value pairs. To support such an approach, we design a general data structure, referred to as the reduction object, which is placed in the memory hierarchy of the GPU. The limited memory requirement of the reduction object allows us to extensively utilize the small but fast shared memory. Our approach performs well for a popular set of MapReduce appli- cations, especially the reduction intensive ones. The comparison with former state-of-art accelerator based approaches shows that our approach is much more efficient at utilizing the shared memory. Even though MapReduce significantly reduces the complexity of parallel programming, it is not easy to achieve efficient execution, for complicated applications, on heterogeneous clusters with multi-core and multiple GPUs within each node. In view of this, we design a programming framework, which aims to reduce the programming difficulty, as well as provide automatic optimizations to applications. Our approach is to classify applications based on communication patterns. The patterns we study include Generalized Reductions, iii Irregular Reductions and Stencil Computations, which are important ones that are frequent- ly used in scientific and data intensive computations. For each pattern, we design a simple API, as well as a runtime with pattern-specific optimizations at different parallelism levels. Besides, we also investigate graph applications. We design a graph processing system over the Intel Xeon Phi and CPU. We design a vertex-centric programming API, and a novel condensed static message buffer that supports less memory consumption and SIMD message reduction. We also use a pipelining scheme to avoid frequent locking. The hybrid graph partitioning is able to achieve load balance between CPU and Xeon Phi, as well as to reduce the communication overhead. Executing irregular applications on SIMD architectures is always challenging. The ir- regularity leads to problems including poor data access locality, data dependency, as well as inefficient utilization of SIMD lanes. We propose a general optimization methodolo- gy for irregular applications, including irregular reductions, graph algorithms and sparse matrix matrix multiplications. The key observation of our approach is that the major data structures accessed by irregular applications can be treated as sparse matrices. The steps of our methodology include: matrix tiling, data access pattern identification, and conflict re- moval. As a consequence, our approach is able to efficiently utilize both SIMD and MIMD parallelism on the Intel Xeon Phi. iv This is dedicated to the ones I love: my grandmother, my parents and my sister. v Acknowledgments There are so many people whom I want to extend my deepest appreciation to. It would not have been possible for me to complete my dissertation, without their advise, help, and support. To only some of them, it is possible to give particular mention here. I would first thank my advisor, Prof. Gagan Agrawal for his guidance. This dissertation would not have been possible without his direction, encouragement and patience. His broad knowledge and extraordinary foresight in the area of high performance computing, as well as his insightful advice, not only established my interest in my research area, but also helped me to develop the capability of identifying and solving problems, which would benefit my future career. At the same time, I sincerely appreciate all his supports, both financial and spiritual, without which it would have been hard for me to pass through the ups and downs during my Ph.D. study. He is not only a successful educator and computer scientist, but also an excellent example for my life. I would also like to thank my thesis committee members, Drs. P. Sadayappan, Feng Qin, and Christopher Charles Stewart. Their incisive and critical analysis, as well as intensive interest have significantly helped me with my research. Thanks to my host family, Woody and Dorothy, the nice couple who provided warm and friendly help when I first arrived in Columbus five years ago. It was their hospitality that helped me to quickly adapt to the new place. I also would like to thank Dr. Kalluri Eswar and Dr. Zhaohui Fu, who helped me to significantly improve my programming skills while I was doing my internship at Google. vi I also appreciate the support from National Science Foundation, Ohio State, as well as Department of Computer Science and Engineering, especially the head of our department, Dr. Xiaodong Zhang, and all friendly staffs in our department, especially Kathryn M. Reeves, Catrena Collins and Lynn Lyons. I also would like to thank the colleagues in our group, Data-Intensive and High Per- formance Computing Research Group. My Ph.D. life would not have been so wonderful without the friendship from them. Former and present members of our lab have provided me with valuable support and help, in both everyday life and research, and they are: Wei Jiang, Vignesh Trichy Ravi, Tantan Liu, Bin Ren, Xin Huo, Tekin Bicer, Yu Su, Yi Wang, Mehmet Can Kurt, Mucahid Kutlu, Sameh Shohdy, Jiaqi Liu, Roee Ebenstein, Surabhi Jain, Gangyi Zhu, Peng Jiang and Jiankai Sun. Finally, special thanks are extended to my parents and my sister, who have been always supportive. I am also thankful to my friends out of our department, as well as the friends who are in China. vii Vita December 5th, 1988 . Born - Ganyu, China 2010 . B.S. Software Engineering, Nanjing University, Nanjing, China 2014 . M.S. Computer Science & Engineering, Ohio State University, Columbus, OH 2014 . Software Engineering Intern, Google, Mountain View, CA Publications Research Publications Linchuan Chen, Peng Jiang, and Gagan Agrawal. “Exploiting Recent SIMD Architec- tural Advances for Irregular Applications”. Under Submission. Linchuan Chen, Xin Huo, Bin Ren, Surabhi Jain, and Gagan Agrawal. “Efficient and Simplified Parallel Graph Processing over CPU and MIC”. In Proceedings of International Parallel & Distributed Processing Symposium 2015 (IPDPS’15), May 2015. Linchuan Chen, Xin Huo, and Gagan Agrawal. “A Pattern Specification and Optimiza- tions Framework for Accelerating Scientific Computations on Heterogeneous Clusters”. In Proceedings of International Parallel & Distributed Processing Symposium 2015 (IPDP- S’15), May 2015. Linchuan Chen, Xin Huo, and Gagan Agrawal. “Scheduling Methods for Accelerating Applications on Architectures with Heterogeneous Cores”. In Proceedings of 23rd Interna- tional Heterogeneity in Computing Workshop (HCW’14) in Conjunction with International Parallel & Distributed Processing Symposium (IPDPS’14), May 2014. viii Linchuan Chen, Xin Huo, and Gagan Agrawal. “Accelerating
Details
-
File Typepdf
-
Upload Time-
-
Content LanguagesEnglish
-
Upload UserAnonymous/Not logged-in
-
File Pages228 Page
-
File Size-