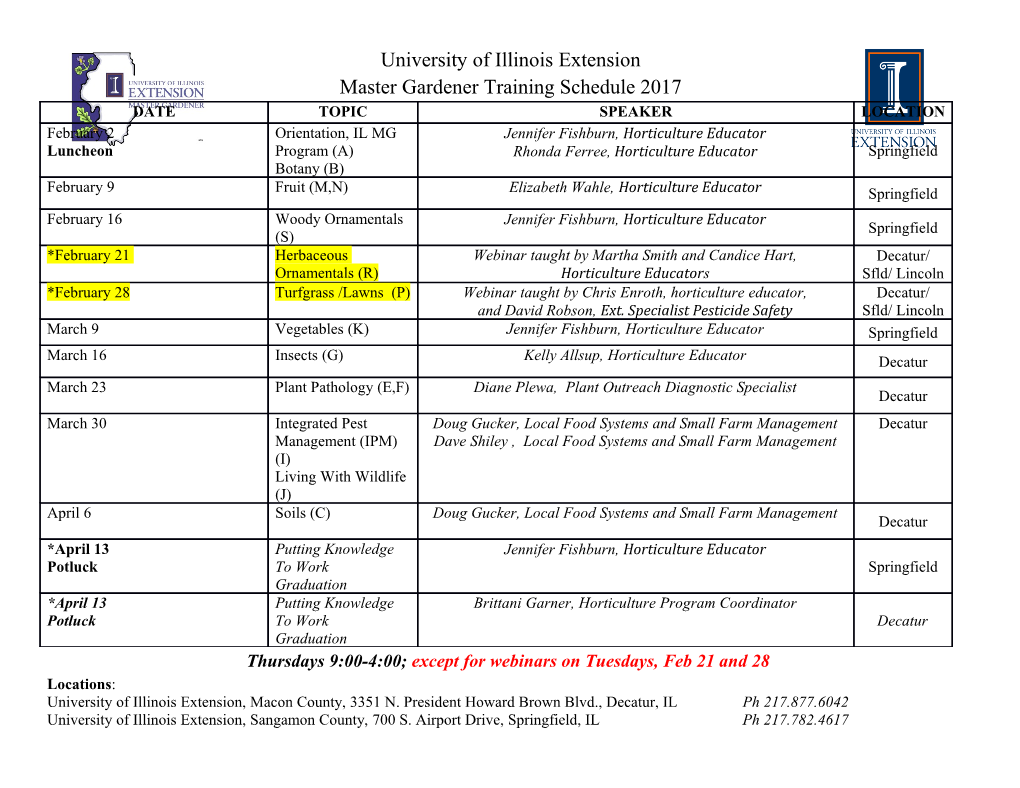
Vector Spaces Matrix Properties Examples Matrix Exponential and Jordan Forms State Space Solutions Module 03 Linear Algebra Review & Solutions to State Space Ahmad F. Taha EE 5143: Linear Systems and Control Email: [email protected] Webpage: http://engineering.utsa.edu/˜taha September 7, 2017 ©Ahmad F. Taha Module 03 — Linear Algebra Review & Solutions to State Space 1 / 32 Vector Spaces Matrix Properties Examples Matrix Exponential and Jordan Forms State Space Solutions Vector Space (aka Linear Space) ©Ahmad F. Taha Module 03 — Linear Algebra Review & Solutions to State Space 2 / 32 Vector Spaces Matrix Properties Examples Matrix Exponential and Jordan Forms State Space Solutions Examples of Vector Space ©Ahmad F. Taha Module 03 — Linear Algebra Review & Solutions to State Space 3 / 32 Vector Spaces Matrix Properties Examples Matrix Exponential and Jordan Forms State Space Solutions Supspaces and Product Spaces ©Ahmad F. Taha Module 03 — Linear Algebra Review & Solutions to State Space 4 / 32 Vector Spaces Matrix Properties Examples Matrix Exponential and Jordan Forms State Space Solutions Bases and Dimension of Vector Spaces ©Ahmad F. Taha Module 03 — Linear Algebra Review & Solutions to State Space 5 / 32 Vector Spaces Matrix Properties Examples Matrix Exponential and Jordan Forms State Space Solutions Linear Maps ©Ahmad F. Taha Module 03 — Linear Algebra Review & Solutions to State Space 6 / 32 Vector Spaces Matrix Properties Examples Matrix Exponential and Jordan Forms State Space Solutions One-To-One Mapping ©Ahmad F. Taha Module 03 — Linear Algebra Review & Solutions to State Space 7 / 32 Vector Spaces Matrix Properties Examples Matrix Exponential and Jordan Forms State Space Solutions Matrix Rank ©Ahmad F. Taha Module 03 — Linear Algebra Review & Solutions to State Space 8 / 32 Vector Spaces Matrix Properties Examples Matrix Exponential and Jordan Forms State Space Solutions Matrix Transpose ©Ahmad F. Taha Module 03 — Linear Algebra Review & Solutions to State Space 9 / 32 Vector Spaces Matrix Properties Examples Matrix Exponential and Jordan Forms State Space Solutions Inner Products ©Ahmad F. Taha Module 03 — Linear Algebra Review & Solutions to State Space 10 / 32 Vector Spaces Matrix Properties Examples Matrix Exponential and Jordan Forms State Space Solutions Eigenvalues and Eigenvectors Eigenvalues/Eigenvectors of a matrix Evalues/vectors are only defined for square1 matrices For a matrix A ∈ Rn×n, we always have n evalues/evectors – Some of these evalues might be distinct, real, repeated, imaginary – To find evalues(A), solve this equation (In: identity matrix of size n) n n−1 det(λIn − A) = 0 or det(A − λIn) = 0 ⇒ a0λ + a1λ + ··· + an = 0 a b Example: det = ad − bc. c d Eigenvectors: A number λ and a non-zero vector v satisfying Av = λv ⇒ (A − λIn)v = 0 are called an eigenvalue and an eigenvector of A – λ is an eigenvalue of an n × n-matrix A if and only if λIn − A is not invertible, which is equivalent to det(A − λIn) = 0. 1A square matrix has equal number of rows and columns. ©Ahmad F. Taha Module 03 — Linear Algebra Review & Solutions to State Space 11 / 32 Vector Spaces Matrix Properties Examples Matrix Exponential and Jordan Forms State Space Solutions Matrix Inverse Inverse of a generic 2by2 matrix: − a b 1 1 d −b 1 d −b A−1 = = = c d det(A) −c a ad − bc −c a −1 −1 – Notice that A A = AA = I2 Inverse of a generic 3by3 matrix: − T a b c 1 ABC ADG 1 1 A−1 = d e f = DEF = BEH det(A) det(A) g h i GHI CFI A = (ei − fh) D = −(bi − ch) G = (bf − ce) B = −(di − fg) E = (ai − cg) H = −(af − cd) C = (dh − eg) F = −(ah − bg) I = (ae − bd) det(A) = aA + bB + cC. −1 −1 – Notice that A A = AA = I3 ©Ahmad F. Taha Module 03 — Linear Algebra Review & Solutions to State Space 12 / 32 Vector Spaces Matrix Properties Examples Matrix Exponential and Jordan Forms State Space Solutions Linear Algebra — Example 1 Find the eigenvalues, eigenvectors, and inverse of matrix 1 4 A = 3 2 – Eigenvalues: λ1,2 = 5, −2 > > – Eigenvectors: v = 1 1 , v = − 4 1 1 2 3 2 −4 – Inverse: A−1 = − 1 10 −3 1 Write A in the matrix diagonal transformation, i.e., A = TDT −1 where D is the diagonal matrix containing the eigenvalues of A: λ1 λ2 − A = v v ··· v v v ··· v 1 1 2 n .. 1 2 n . λn – Only valid for matrices with distinct, real eigenvalues ©Ahmad F. Taha Module 03 — Linear Algebra Review & Solutions to State Space 13 / 32 Vector Spaces Matrix Properties Examples Matrix Exponential and Jordan Forms State Space Solutions Rank of a Matrix Rank of a matrix: rank(A) is equal to the number of linearly independent rows or columns 1 1 0 2 – Example 1: rank =? −1 −1 0 −2 1 2 1 – Example 2: rank −2 −3 1 =? 3 5 0 Rank computation: reduce the matrix to a simpler form, generally row echelon form, by elementary row operations – Example 2 Solution: 1 2 1 1 2 1 1 2 1 −2 −3 1 → 2r1 + r2 0 1 3 → −3r1 + r3 0 1 3 3 5 0 3 5 0 0 −1 −3 1 2 1 1 0 −5 → r2 + r3 0 1 3 → −2r2 + r10 1 3 ⇒ rank(A) = 2 0 0 0 0 0 0 For a matrix A ∈ Rm×n: rank(A) ≤ min(m, n) ©Ahmad F. Taha Module 03 — Linear Algebra Review & Solutions to State Space 14 / 32 Vector Spaces Matrix Properties Examples Matrix Exponential and Jordan Forms State Space Solutions Null Space of a Matrix The Null Space of any matrix A is the subspace K defined as follows: N(A) = Null(A) = ker(A) = {x ∈ K|Ax = 0} Null(A) has the following three properties: – Null(A) always contains the zero vector, since A0 = 0 – If x ∈ Null(A) and y ∈ Null(A), then x + y ∈ Null(A) – If x ∈ Null(A) and c is a scalar, then cx ∈ Null(A) Example: Find N(A) a 2 3 5 2 3 5 0 2 3 5 0 A = ⇒ b = ⇒ ⇒ −4 2 3 −4 2 3 0 −4 2 3 0 c a −1/16 −1 1 0 1/16 0 1 13 ⇒ a = − c, b = − c ⇒ b = α −13/8 =α ˜ −26 0 1 13/8 0 16 8 c 1 16 ©Ahmad F. Taha Module 03 — Linear Algebra Review & Solutions to State Space 15 / 32 Vector Spaces Matrix Properties Examples Matrix Exponential and Jordan Forms State Space Solutions Linear Algebra — Example 2 Find the determinant, rank, and null-space set of this matrix: 0 1 2 B = 1 2 1 2 7 8 – det(B) = 0 – rank(B) = 2 3 – null(B) = α −2 , ∀ α ∈ R 1 Is there a relationship between the determinant and the rank of a matrix? – Yes! Matrix drops rank if determinant = zero ⇒ 1 zero evalue True or False? – AB = BA for all A and B—FALSE! – A and B are invertible → (A + B) is invertible—FALSE! ©Ahmad F. Taha Module 03 — Linear Algebra Review & Solutions to State Space 16 / 32 Vector Spaces Matrix Properties Examples Matrix Exponential and Jordan Forms State Space Solutions Matrix Exponential — 1 Exponential of scalar variable: ∞ X ai a2 a3 a4 ea = = 1 + a + + + + ··· i! 2! 3! 4! i=0 Power series converges ∀ a ∈ R How about matrices? For A ∈ Rn×n, matrix exponential: ∞ X Ai A2 A3 A4 eA = = I + A + + + + ··· i! n 2! 3! 4! i=0 What if we have a time-variable? ∞ X (tA)i (tA)2 (tA)3 (tA)4 etA = = I + tA + + + + ··· i! n 2! 3! 4! i=0 ©Ahmad F. Taha Module 03 — Linear Algebra Review & Solutions to State Space 17 / 32 Vector Spaces Matrix Properties Examples Matrix Exponential and Jordan Forms State Space Solutions Matrix Exponential Properties For a matrix A ∈ Rn×n and a constant t ∈ R: At λt 1 Av = λv ⇒ e v = e v At A t 2 2det(e ) = e(trace( )) At − −At 3 (e ) 1 = e A>t At > 4 e = (e ) A B t At Bt Bt At 5 If A, B commute, then: e( + ) = e e = e e A t t At At At At 6 e ( 1+ 2) = e 1 e 2 = e 2 e 1 2Trace of a matrix is the sum of its diagonal entries. ©Ahmad F. Taha Module 03 — Linear Algebra Review & Solutions to State Space 18 / 32 Vector Spaces Matrix Properties Examples Matrix Exponential and Jordan Forms State Space Solutions When Is It Easy to Find eA? Method 1 Well...Obviously if we can directly use eA = I + A + A2 + ··· n 2! Three cases for Method 1 Case 1 A is nilpotent3, i.e., Ak = 0 for some k. Example: 5 −3 2 A = 15 −9 6 10 −6 4 Case 2 A is idempotent, i.e., A2 = A. Example: 2 −2 −4 A = −1 3 4 1 −2 −3 Case 3 A is of rank one: A = uv T for u, v ∈ Rn Ak = (v T u)k−1A, k = 1, 2,... 3Any triangular matrix with 0s along the main diagonal is nilpotent ©Ahmad F. Taha Module 03 — Linear Algebra Review & Solutions to State Space 19 / 32 Vector Spaces Matrix Properties Examples Matrix Exponential and Jordan Forms State Space Solutions Method 2 — Jordan Canonical Form All matrices, whether diagonalizable or not, have a Jordan canonical form: A = TJT −1, then eAt = TeJt T −1 λi 1 J 1 .. λi . Generally, J = .. J = ∈ ni ×ni ⇒ . i . R .. 1 Jp λi ni −1 λi t eλi t teλi t ... t e (ni −1)! eJ1t . ni −2 λi t λi t . t e Ji t 0 e .
Details
-
File Typepdf
-
Upload Time-
-
Content LanguagesEnglish
-
Upload UserAnonymous/Not logged-in
-
File Pages32 Page
-
File Size-